A Comparison of Policy Gradient Methods for Multitask Learning
semanticscholar
摘要
This paper compares two policy gradient methods using multitask learning (MTL) on the Atari visual environments. These environments are complex and take millions of time steps to learn. This paper investigates Advantage Actor-Critic (A2C) and Proximal Policy Optimization’s (PPO) performance on one, two and four tasks from the Arcade Learning Environment. The results show that agents trained with both PPO and A2C have improved performance when trained on multiple tasks when compared to a single task. PPO showed the most consistent improvement and scored the best overall. However, A2C’s improved the most on average compared to its baseline. This shows that the trust-region approximation of PPO may not be as beneficial in MTL as in a single task.
更多查看译文
AI 理解论文
溯源树
样例
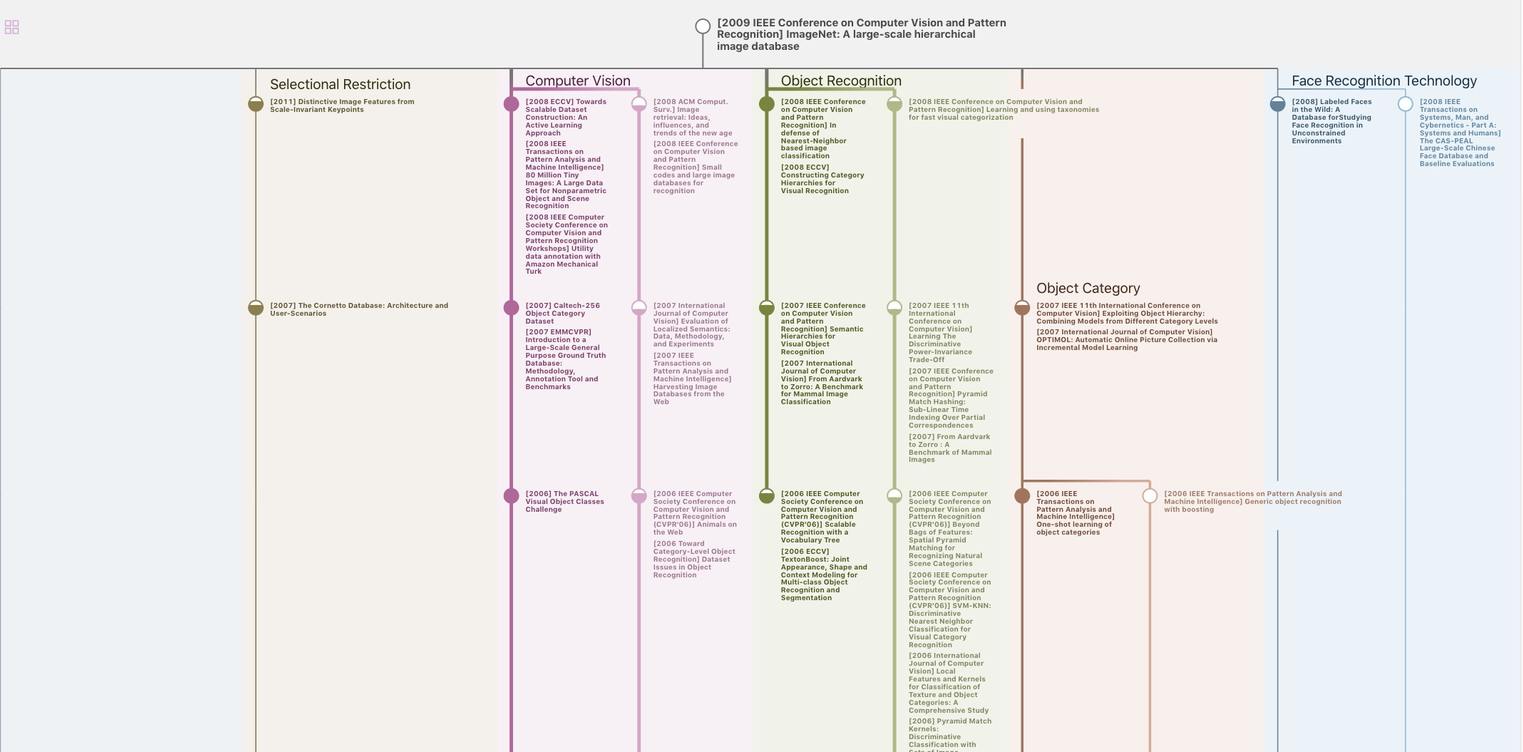
生成溯源树,研究论文发展脉络
Chat Paper
正在生成论文摘要