Dual-Domain Reconstruction Networks with V-Net and K-Net for fast MRI.
CoRR(2022)
摘要
Partial scan is a common approach for accelerating Magnetic Resonance Imaging (MRI) data acquisition. However, it is challenging to accurately reconstruct images from partial scan data (i.e., incomplete k-space matrices). Most state-of-the-art reconstruction methods apply U-Net (a classical encoder-decoder form of convolutional neural network) or cascaded U-Nets in image domain and/or k-space domain. These methods have great advantages over traditional methods where deep learning is not involved in. Nevertheless, these methods have following problems: (1) Directly applying U-Net in k-space domain is not optimal for extracting features in k-space domain; (2) Classical image-domain oriented U-Net is heavy-weight and hence is inefficient to be cascaded many times for yielding good reconstruction accuracy; (3) Classical image-domain oriented U-Net does not fully make use information of encoder network for extracting features in decoder network; and (4) Existing methods are ineffective in simultaneously extracting and fusing features in image domain and its dual k-space domain. To tackle these problems, we propose in this paper (1) an imagedomain encoder-decoder sub-network called V-Net which is more light-weight for cascading and effective in fully utilizing features in the encoder for decoding, (2) a k-space domain sub-network called K-Net which is more suitable for extracting hierarchical features in k-space domain, and (3) a dual-domain reconstruction network where V-Nets and KNets are parallelly and effectively combined and cascaded. The effectiveness of KV-Net is demonstrated on the challenging fastMRI dataset where large-scale raw k-space training data are available and ground truth of test data is not released.
更多查看译文
AI 理解论文
溯源树
样例
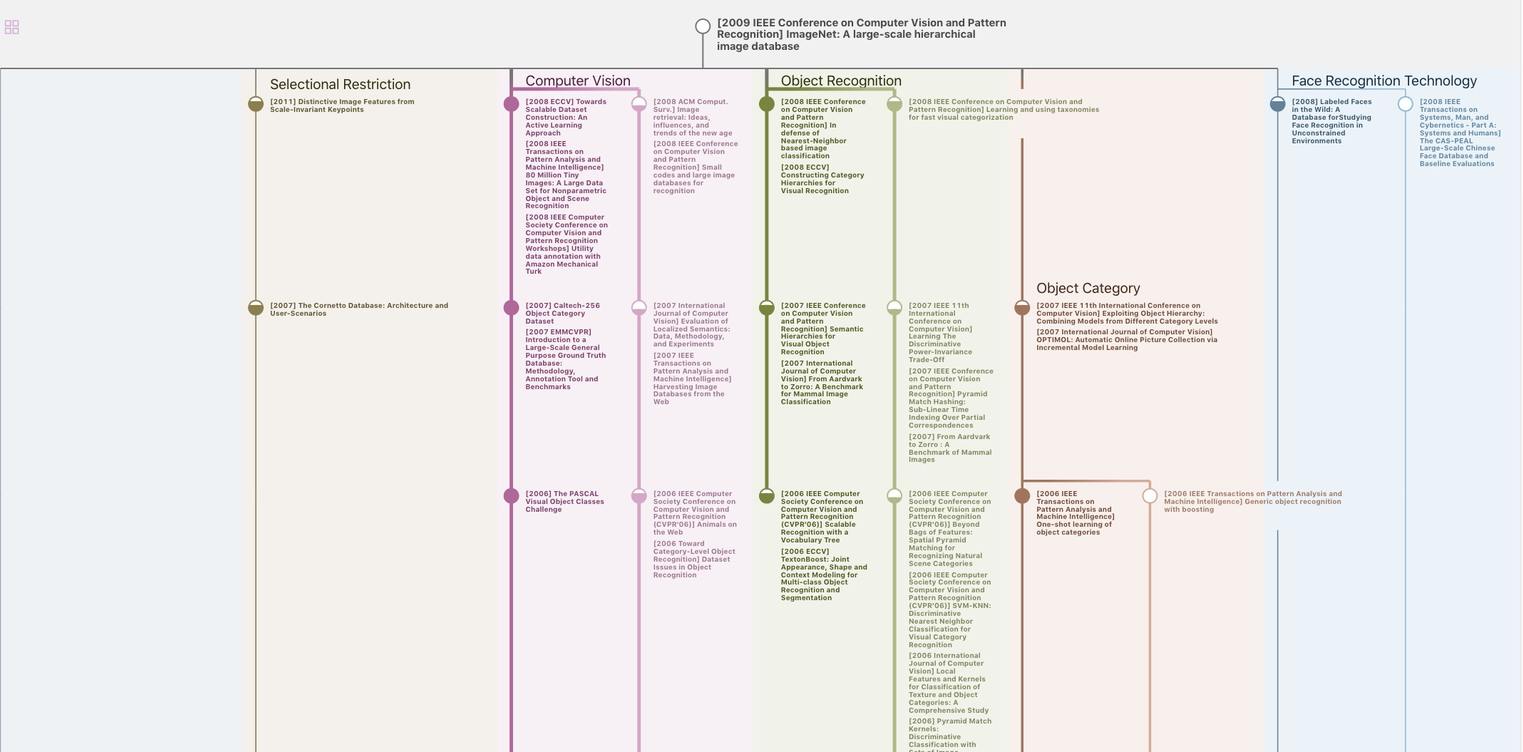
生成溯源树,研究论文发展脉络
Chat Paper
正在生成论文摘要