Automatic No-reference Quality Assessment in Chest Radiographs Based on Deep Convolutional Neural Networks
Research Square (Research Square)(2022)
Abstract
Abstract Background: Chest radiography is the most frequently performed examinations in the department of radiology. The image quality plays an essential role in further decision making of dignosis. The current manual assessment is low-efficiency with significant inter-observer variability. The purpose of this study is to develop and evaluate the performance of deep learning-based model for the automatic quality assessment in chest radiographs. Methods: A set of 1138 posterior-anterior chest radiographs were included in this retrospective study, which were randomly divided into training (n = 826), validation (n = 207), and testing sets (n = 105). The image quality was evaluated on the aspect of gray-level and sharpness. Ten experienced experts independently assessed all radiographs with a score of 1 to 10 based on their subjective perception for the image quality. On the testing set, three of the ten experts classified chest radiographs as acceptable or non-acceptable based on the image quality further. A neural network model CaHDC-RGA was trained to output the quality score of the gray-level and sharpness automaticlly. The intra-class correlation coefficient (ICC), Pearson correlation coefficient (r), and mean absolute difference (MAD) were used for quantitative scoring. The AUC value, sensitivity, and specificity were used for binary classification. Time spent by model and experts were recorded, respectively. Results: A statistically significant correlation was observed between the model and experts on the aspect of gray-level (ICC=0.92, r = 0.91, MAD=0.45) and sharpness (ICC=0.90, r = 0.89, MAD=0.44). For the classification of image quality as acceptable or non-acceptable, the model achieved an AUC of 0.972 for gray-level, with a sensitivity of 93.90% and a specificity of 95.69%. The AUC for sharpness was 0.970, with a sensitivity of 87.95% and a specificity of 100%. The average time spent by the model was significantly shorter than the human expert's (3.03 seconds VS. 10.96 seconds, P < 0.05).Conclusions: The developed deep learning model could rapidly and automatically evaluate the gray-level and sharpness of chest radiographs, the performance of the model was comparable with the subjective perception of human experts. The model may be further applied to automated quality audits of full samples.
MoreTranslated text
Key words
chest radiographs,no-reference
AI Read Science
Must-Reading Tree
Example
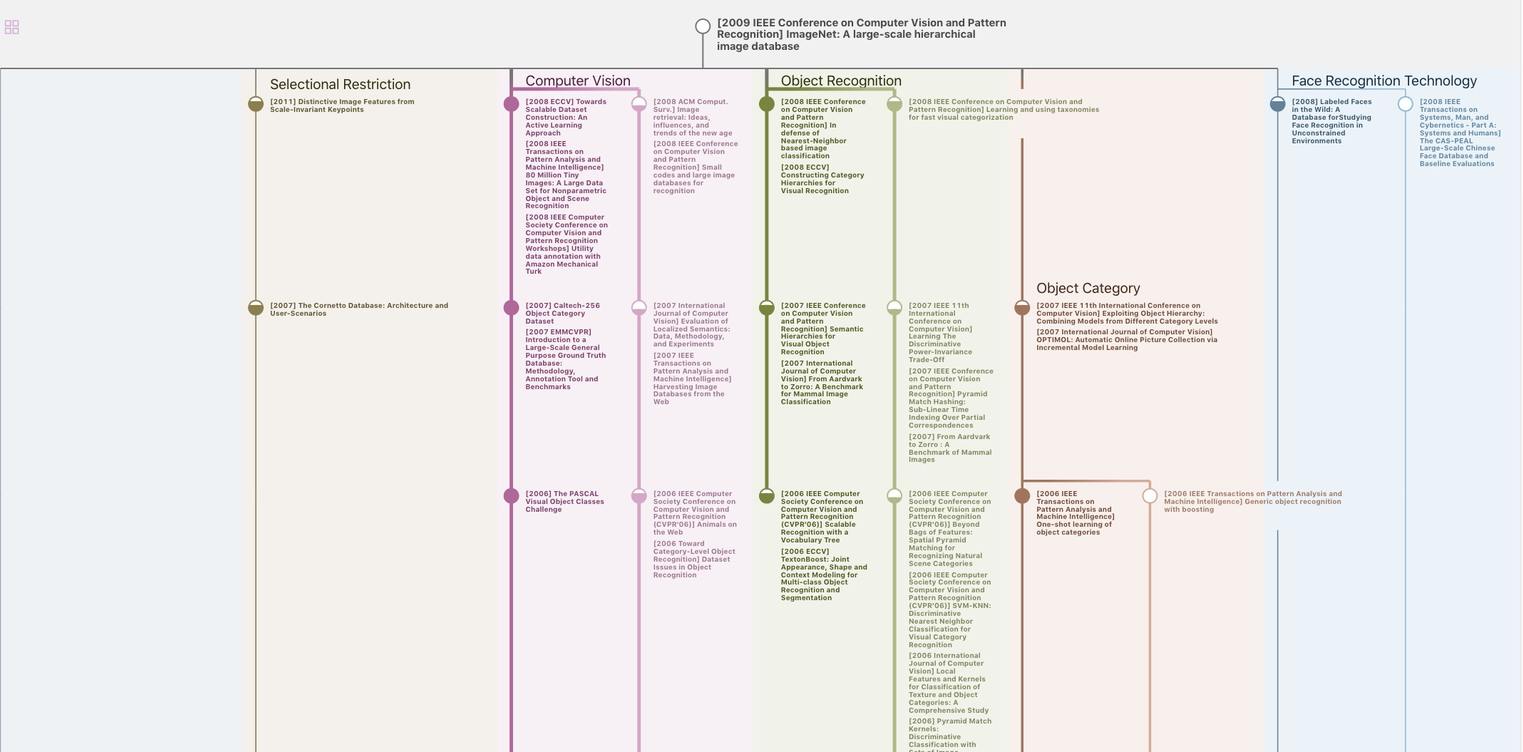
Generate MRT to find the research sequence of this paper
Chat Paper
Summary is being generated by the instructions you defined