Multi-scale low-rank approximation method for image denoising
Multimedia Tools and Applications(2022)
摘要
Nonlocal self-similarity (NSS) as a prior has been widely used in image denoising techniques, such as block-matching and 3-D filtering (BM3D) and weighted nuclear norm minimization (WNNM). However, for local regions that do not have sufficiently similar structures in the image, these methods usually produce ringing artifacts. In light of the fact that NSS exists within both the same scale and different scales, this paper proposes an image denoising method that simultaneously explores multi-scale NSS prior and low-rank prior of natural images. Specifically, we first select similar patches from different scales to make full use of multi-scale NSS prior. Then, a constrained nuclear norm minimization model is introduced to account for low-rank prior. Finally, eigenvalue thresholding technique is exploited to obtain the solution. We demonstrate the proposed method on both synthetic and real noisy image denoising. Results show competitive performance in terms of quantification and visualization compared to state-of-the-art competing methods.
更多查看译文
关键词
Multi-scale nonlocal self-similarity,Low-rank approximation,Singular value decomposition,Image denoising
AI 理解论文
溯源树
样例
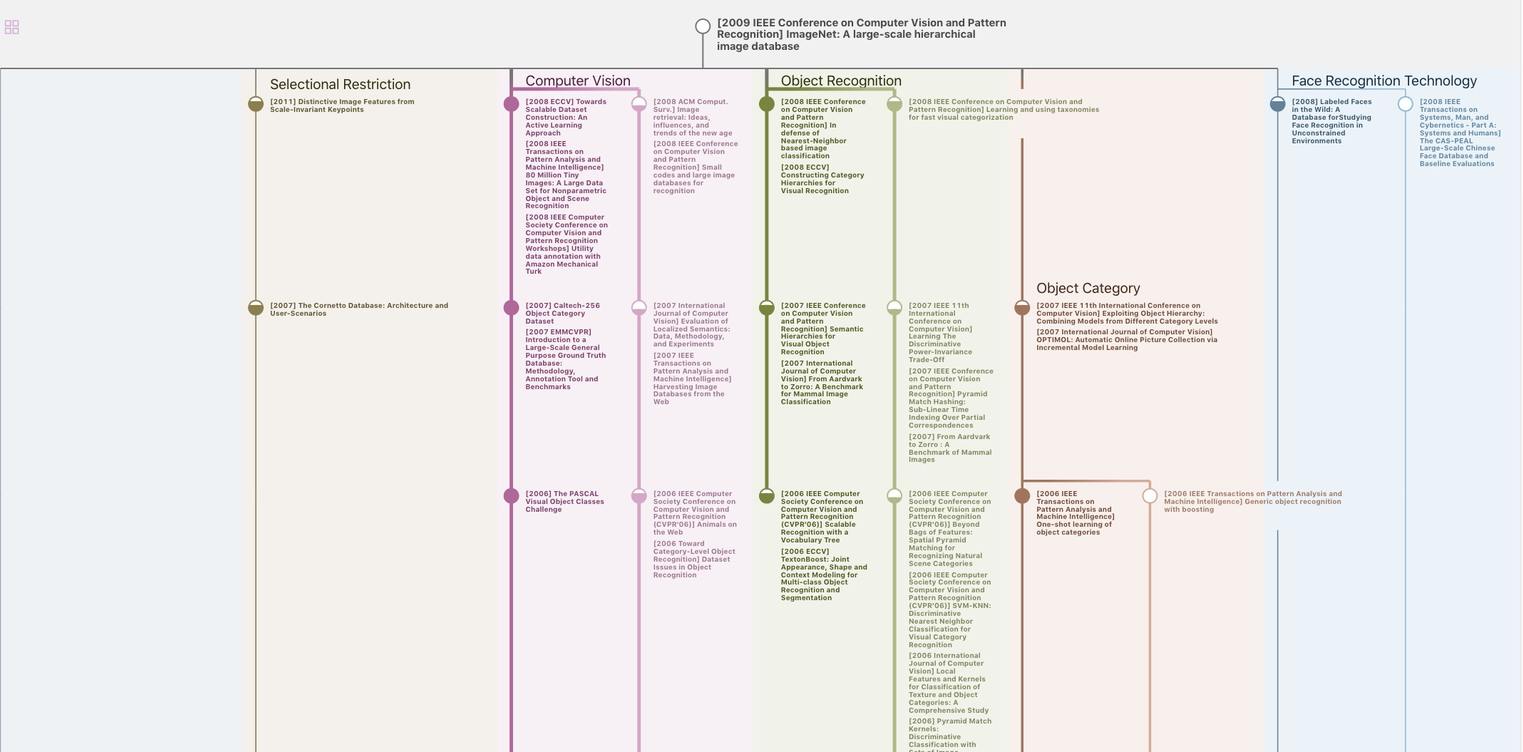
生成溯源树,研究论文发展脉络
Chat Paper
正在生成论文摘要