Prediction of Alpine Foehn from time series of GNSS troposphere products using machine learning
semanticscholar(2022)
摘要
Abstract. Remote sensing of water vapor using the Global Navigation Satellite System (GNSS) is a well-established technique and reliable data source for Numerical Weather Prediction (NWP). One of the phenomena rarely studied using GNSS are foehn winds. Since foehn winds are associated with significant humidity gradients between lee/luv side of a mountain range, tropospheric estimates from GNSS are also affected by their occurrence. Time series reveal characteristic features like distinctive minima/maxima and significant decrease in correlation between the stations. However, detecting such signals becomes increasingly difficult for large data sets. Therefore, we suggest the application of machine learning algorithms for detection and prediction of foehn events from GNSS troposphere products. The present study uses long-term time series of high-quality GNSS troposphere products from the Automated GNSS Network Switzerland (AGNES) as well as records of operational foehn index to investigate the performance of several different classification algorithms based on appropriate statistical metrics. The two best-performing algorithms are fine-tuned and employed on two years of test data. The results show very promising results, especially when reprocessed GNSS products are utilized. Detection- and alarm-based measures reach levels of 70–85 % for both tested algorithms and thus are comparable to those from studies using data from meteorological stations and NWP. For operational prediction, some limitations due to the availability and quality of GNSS products in near-real time (NRT) exist. However, they might be mitigated to a significant extend by provision of additional NRT products and improved data processing in the future.
更多查看译文
AI 理解论文
溯源树
样例
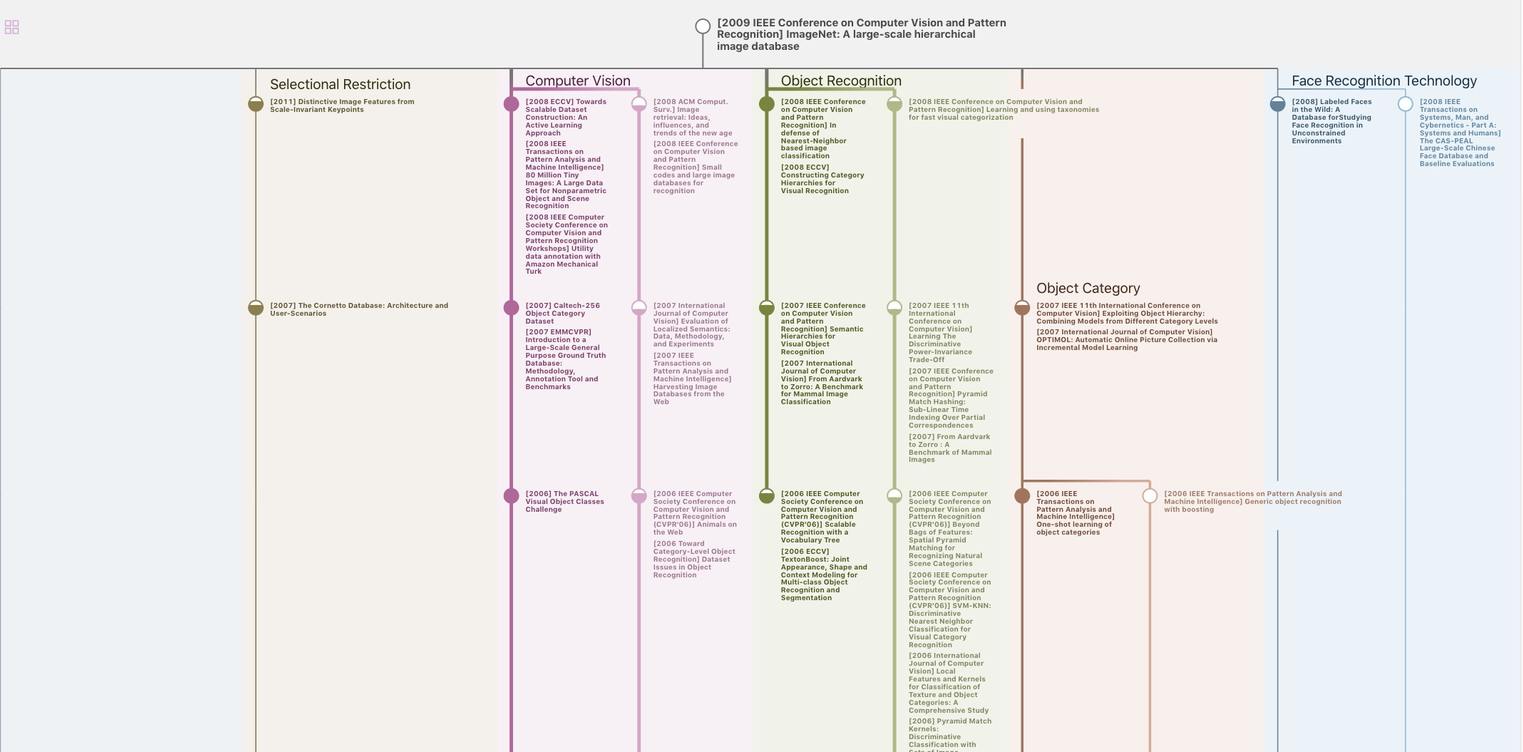
生成溯源树,研究论文发展脉络
Chat Paper
正在生成论文摘要