UvA-DARE Approximate Slice Sampling for Bayesian Posterior Inference Approximate Slice Sampling for Bayesian Posterior Inference
semanticscholar(2014)
摘要
In this paper, we advance the theory of large scale Bayesian posterior inference by intro-ducing a new approximate slice sampler that uses only small mini-batches of data in every iteration. While this introduces a bias in the stationary distribution, the computational savings allow us to draw more samples in a given amount of time and reduce sampling variance. We empirically verify on three different models that the approximate slice sampling algorithm can significantly outperform a traditional slice sampler if we are allowed only a fixed amount of computing time for our simulations.
更多查看译文
AI 理解论文
溯源树
样例
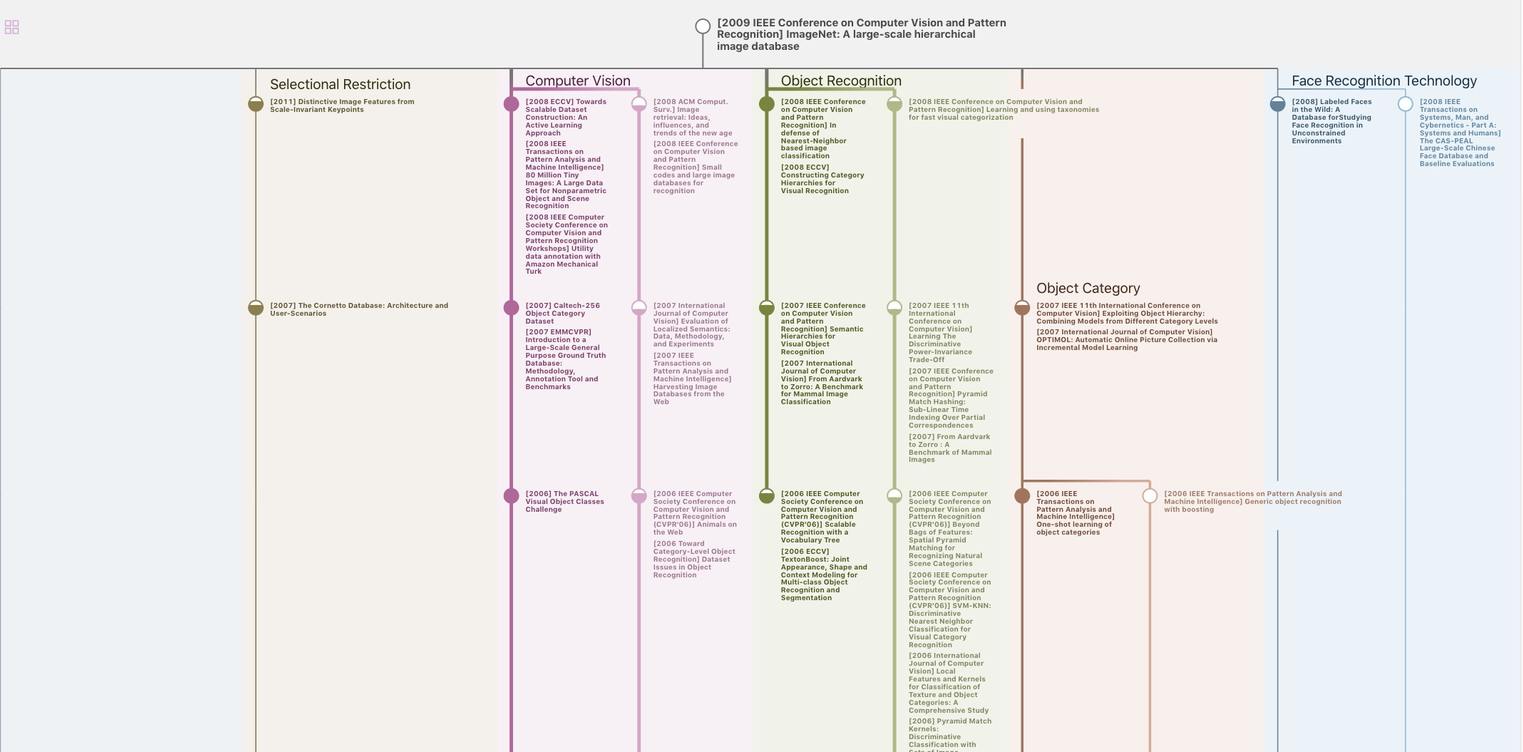
生成溯源树,研究论文发展脉络
Chat Paper
正在生成论文摘要