Enhancing Race and Ethnicity using Bayesian Imputation in an All Payer Claims Database
semanticscholar(2022)
摘要
Background: All Payer Claims Databases (APCD) are a rich source of health information, however, race and ethnicity (R&E) data are largely missing. Bayesian Improved Surname Geocoding (BISG) is a common R&E imputation method, yet, validation of BISG in APCDs is lacking. We used the BISG to impute missing R&E in the Oregon APCD. Methods: BISG imputed R&E for Asian Pacific Islanders (API), Blacks, Hispanics and Whites were contrasted to the gold standard (vital statistics) and sensitivity and specificity improvements were assessed. Logistic regression examined whether missing R&E was random across patient characteristics. Results: Among 85,857 individuals in the study, 32.1% (n=27,594) had missing R&E. Missing R&E was not randomly distributed. There were higher odds of missingness among males, Whites, those age 65 and older, and commercially insured individuals. Differences in the percent missing were also found by co-morbid conditions and mortality causes. Imputing the missing R&E with BISG method improved the sensitivity to identify White, Black, API, and Hispanics. Conclusions: APCDs can benefit from enhancing missing R&E with BISG imputation to perform more robust population-health level analyses and identify inequities according to R&E without losing power or dropping non-random records with missing R&E data.
更多查看译文
AI 理解论文
溯源树
样例
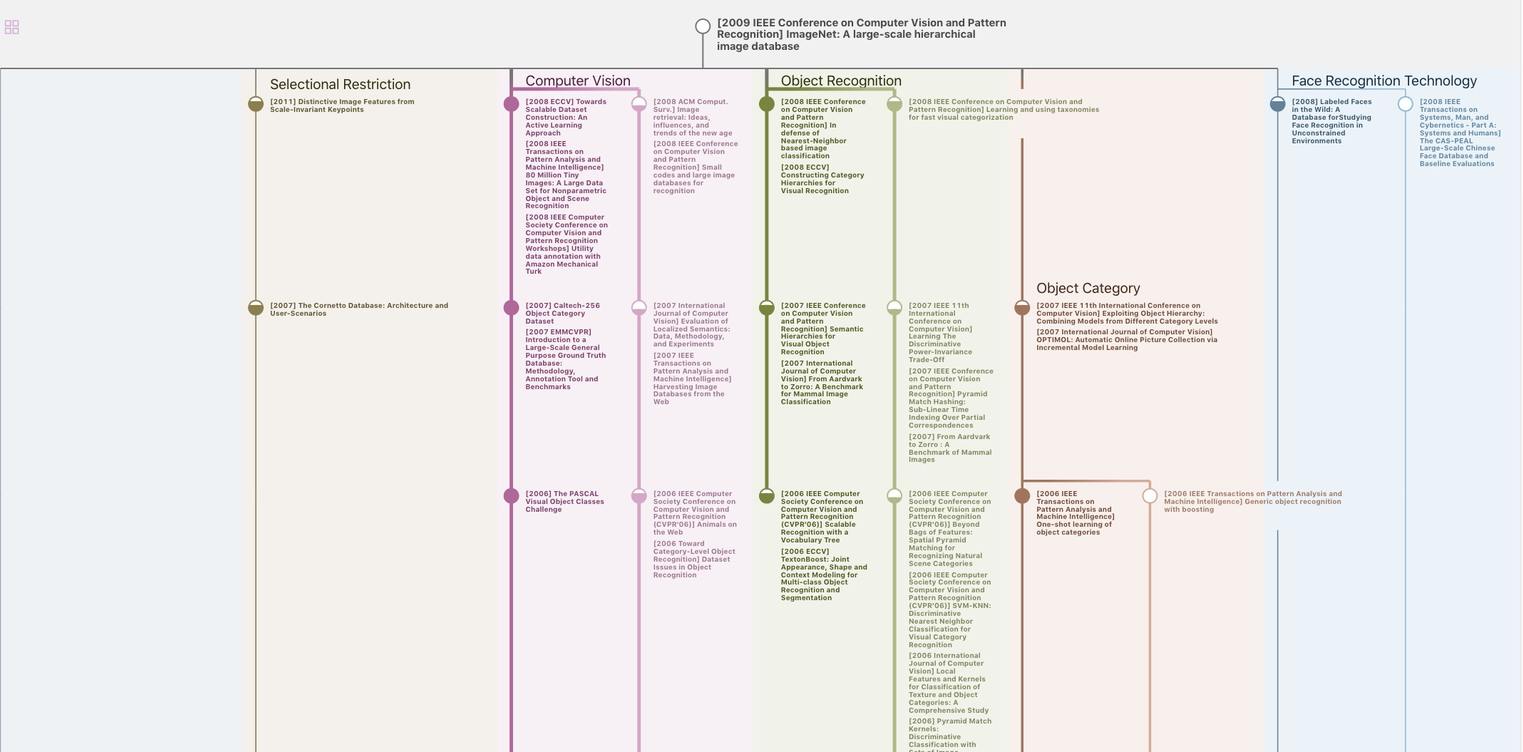
生成溯源树,研究论文发展脉络
Chat Paper
正在生成论文摘要