Counterfactual Generation Through Multi-objective Constrained Optimisation
semanticscholar(2022)
摘要
Explainable artificial intelligence (XAI) techniques are often adopted to provide transparent feedback to decision-makers evaluating the results of trained machine learning (ML) models. One of these techniques covers the generation of counterfactuals -- slightly modified instances based on a given input that can change its predicted outcome. These counterfactuals enable decision-makers to know what actions they could do to modify an outcome and improve the trust and transparency of a trained ML model. This research aims to develop and apply a novel constrained multi-objective optimisation design (MOOD) to generate counterfactuals in classification and regression problems. The MOOD is composed of three steps: the definition of a constrained multi-objective problem (MOP); the multi-objective optimisation (MOO) using a multi-objective evolutionary algorithm (MOEA); and the multi-criteria decision making (MCDM) with a domination filter and the TOPSIS ranking technique. This MOOD generated large numbers of valid counterfactuals and selected and ranked only the most relevant to the decision-maker. Furthermore, the results show that the proposed MOOD outperformed other architectures available for production use in at least one of the objectives measured for all datasets analysed in both regression and classification problems.
更多查看译文
AI 理解论文
溯源树
样例
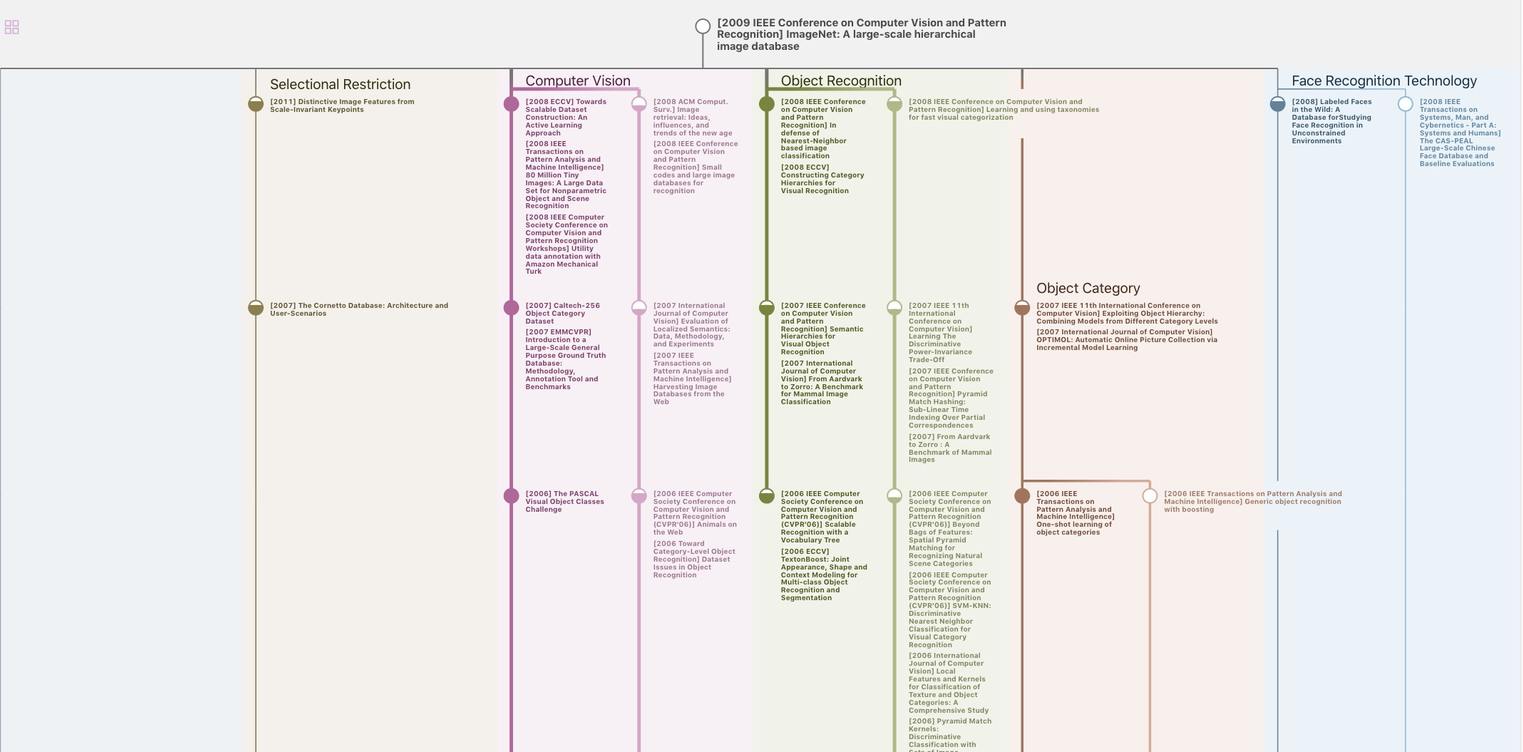
生成溯源树,研究论文发展脉络
Chat Paper
正在生成论文摘要