Unsupervised sparse deconvolutional learning of features driving neural activity∗
semanticscholar(2022)
Abstract
Understanding the activity of single neurons in relation to features in the environment is the first step in many neuroscience studies. We propose a method using algorithm unrolling, an emerging technique in interpretable deep learning, to deconvolve single-trial neuronal activity into interpretable components. Specifically, we model the firing rates of single neurons using a set of kernels characterizing neurons’ responses to time-sensitive sparse events/stimuli. The kernels can be either unique or shared across the population and are weighted by codes whose amplitude and timing are trial-specific. Our inference results in a deep sparse deconvolutional encoder and, unlike sequential deep encoder approaches, is based on a generative model; hence, the learned parameters and encoding are directly interpretable. First, we characterize the performance regime of our method; this guides end users to understand the model’s accuracy and limitations. Second, we apply our method to deconvolve overlapping signals in the response of dopaminergic neurons to rewards of varying size. Previous studies have suggested that reward prediction error responses of dopaminergic neurons are modulated by two components: salience and value. However, this multiplexing is often ignored or analyzed using ad-hoc windows to estimate the two contributions. Here, we deconvolve the two factors in an unsupervised manner; one kernel corresponds to salience whose code is common across reward sizes and another to value whose code changes as a function of reward amount. We show that the inferred codes are more informative than firing rates estimated using ad-hoc windows. Third, we study the response of piriform cortex neurons to brief odor pulses delivered at random time across trials. Based on the learned neural impulse responses, we uncover 3 clusters of response types across the population. Overall, we propose a novel method to deconvolve into interpretable components the factors driving neural activity in single trials. Methods. Given a neuron’s activity, the spikes at trial j are binned at B ms resolution and modeled using the
MoreTranslated text
AI Read Science
Must-Reading Tree
Example
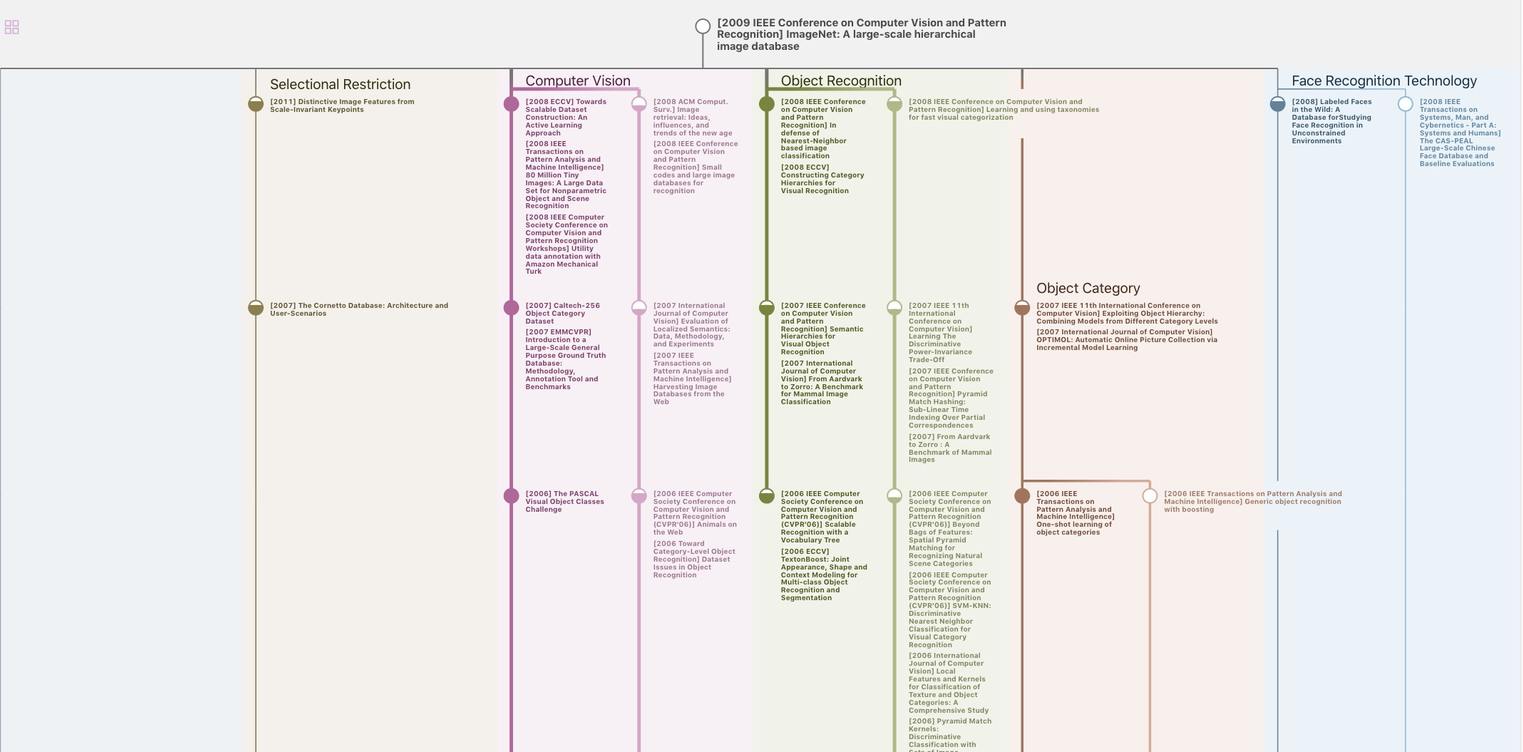
Generate MRT to find the research sequence of this paper
Chat Paper
Summary is being generated by the instructions you defined