Do DNNs trained on Natural Images acquire Gestalt Properties?
ArXiv(2022)
摘要
Under some circumstances, humans tend to perceive individual elements as a group or ‘whole’. This has been widely investigated for more than a century by the school of Gestalt Psychology, which formulated several laws of perceptual grouping. Recently, Deep Neural Networks (DNNs) trained on natural images have been proposed as compelling models of human vision based on reports that they learn internal representations similar to the primate ventral visual stream and show similar patterns of errors in object classification tasks. That is, DNNs often perform well on brain and behavioral benchmarks. Here we compared human and DNN responses in discrimination judgments that assess a range of Gestalt organization principles (Pomerantz et al., 1977; Pomerantz and Portillo, 2011). Amongst the DNNs tested we selected models that perform well on the Brain-Score benchmark (Schrimpf et al., 2018). We found that network trained on natural images exhibited sensitivity to shapes at the last stage of classification, which in some cases matched humans responses. When shape familiarity was controlled for (by using dot patterns that would not resemble shapes) we found the networks were insensitive to the standard Gestalt principles of proximity, orientation, and linearity, which have been shown to have a strong and robust effect on humans. This shows that models that perform well on behavioral and brain benchmarks nevertheless miss fundamental principles of human vision.
更多查看译文
AI 理解论文
溯源树
样例
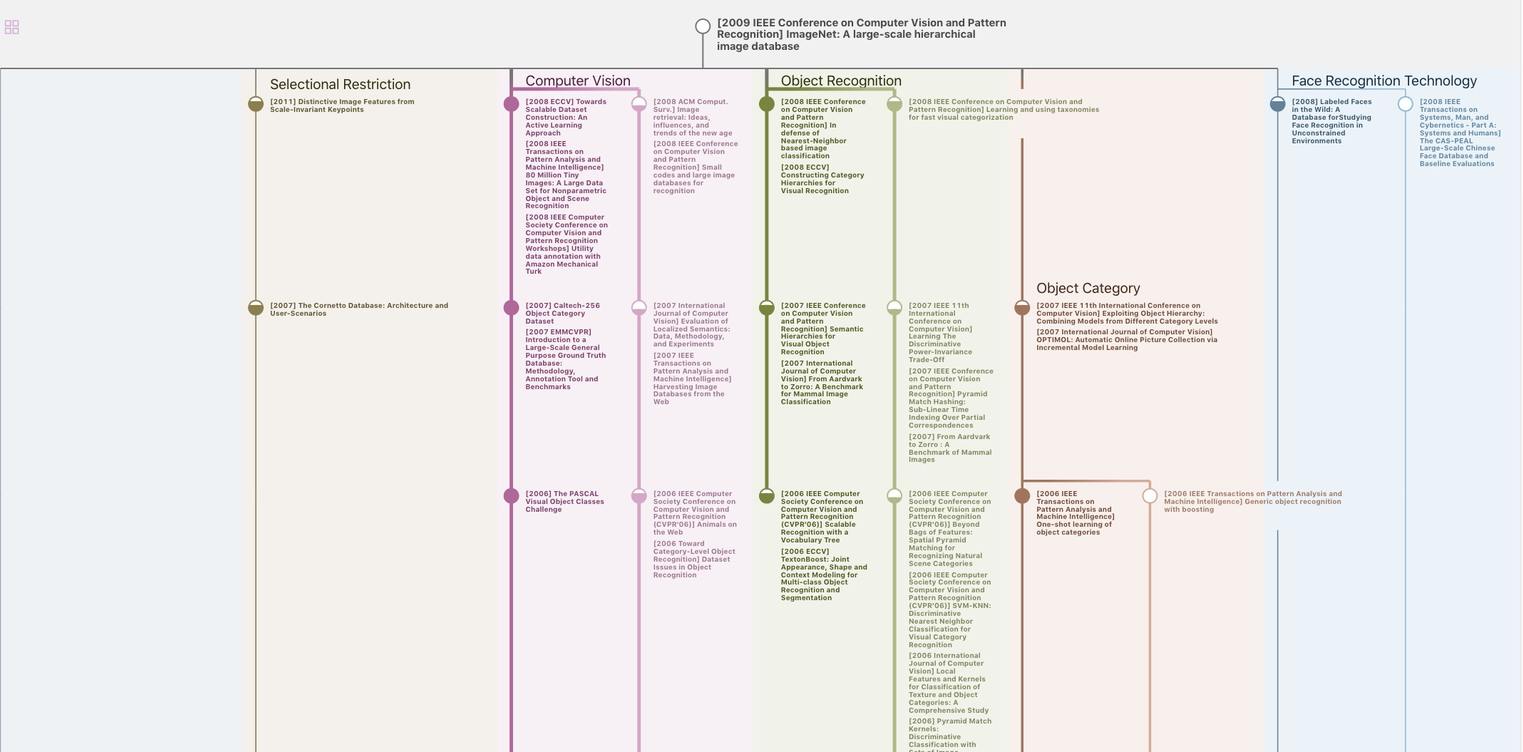
生成溯源树,研究论文发展脉络
Chat Paper
正在生成论文摘要