Correlation-Based Data Augmentation for Machine Learning and Its Application to Road Environment Recognition
IEEE Transactions on Vehicular Technology(2022)
摘要
The accuracy of machine learning depends largely on the quantity and quality of the training data. However, it is generally difficult to prepare a large number of high-quality data. To generate diverse image data, image generation techniques using deep learning, such as a generative adversarial network, can be used. However, because these methods require a large number of training data and a significant calculation time, they are unsuitable for generating training data for machine learning. In this article, we propose an image data augmentation model based on the statistical properties of the training data. With the proposed method, each image is divided into sub-regions based on the correlation calculated using a set of images. The image of each sub-region is modeled through a Gaussian mixture, and data augmentation is conducted by generating images based on this model. The proposed method does not require a large number of training data and can generate data within a relatively short calculation time. The proposed method is applied to the task of road environment recognition. The experiment results showed that the accuracy was improved through image augmentation using the proposed model.
更多查看译文
关键词
Data augmentation,statistical model,correlation,road environment recognition
AI 理解论文
溯源树
样例
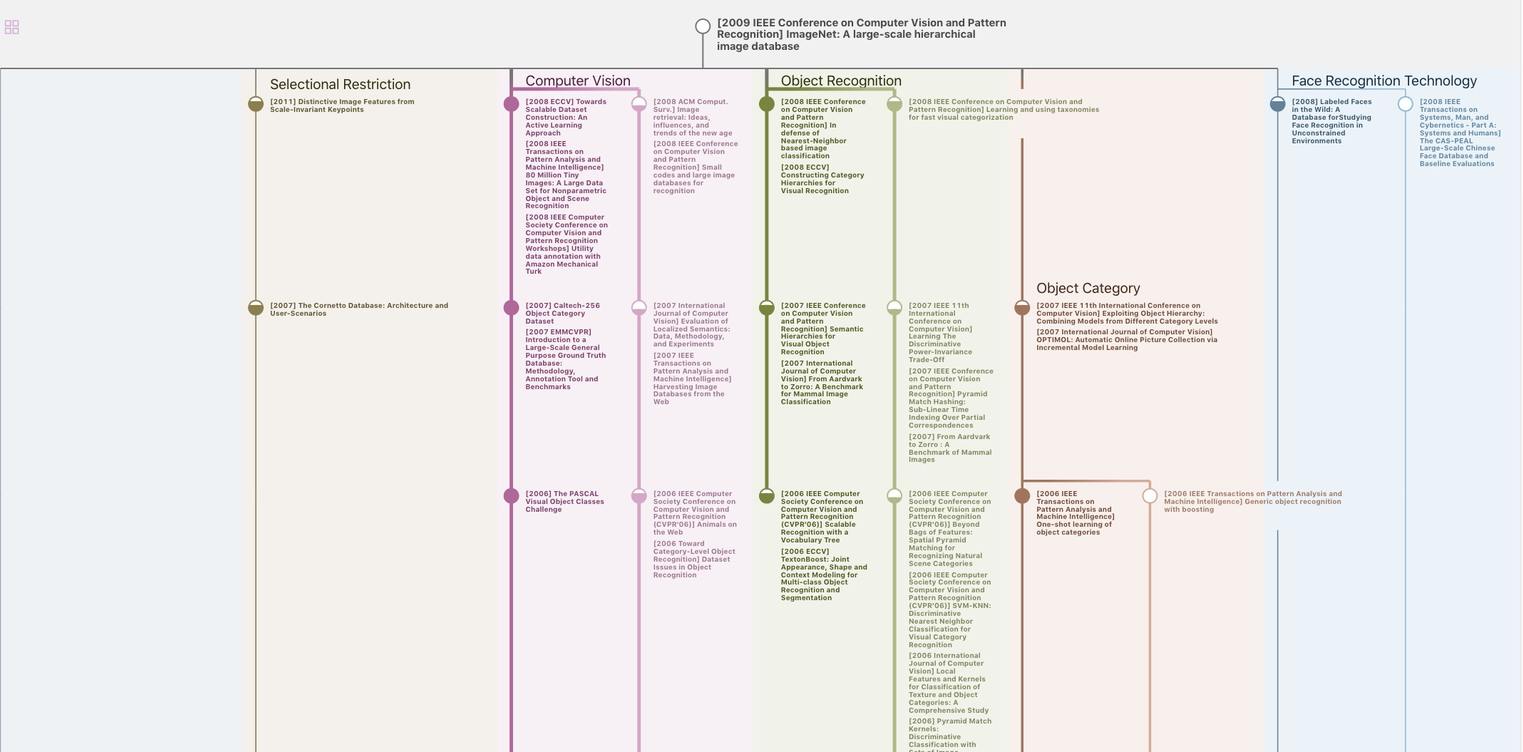
生成溯源树,研究论文发展脉络
Chat Paper
正在生成论文摘要