Improved transfer learning of CNN through fine-tuning and classifier ensemble for scene classification
Soft Computing(2022)
摘要
In high-resolution remote sensing imageries, the scene classification is one of the challenging problems due to the similarity of image structure and available datasets are all small. Performing training with small datasets on new convolutional neural network (CNN) is inclined to overfitting, and attainability is poor. To overcome this, we go for a stream of transfer learning, fine-tuning strategy. Here, we consider AlexNet, VGG 19, and VGG 16 pre-trained CNNs. First, design a network by replacing the classifier stage layers with revised ones through transfer learning. Second, apply fine-tuning from right to left and perform retraining on the classifier stage and part of the feature extraction stage (last convolutional block). Third, form a classifier ensemble by using the majority voting learner strategy to explore better classification results. The datasets called UCM and SIRI-WHU were used and compared with the state-of-the-art methods. Finally, to check the usefulness of our proposed methods, form sub-datasets from AID and WHU-RS19 datasets with likely labeled class names. To assess the performance of the proposed classifiers compute overall accuracy using confusion matrix and F1-score. The results of the proposed methods improve the accuracy from 93.57 to 99.04% for UCM and 91.34 to 99.16% for SIRI-WHU.
更多查看译文
关键词
Classifier ensemble,Fine-tuning,Pre-trained network,Transfer learning,Scene classification
AI 理解论文
溯源树
样例
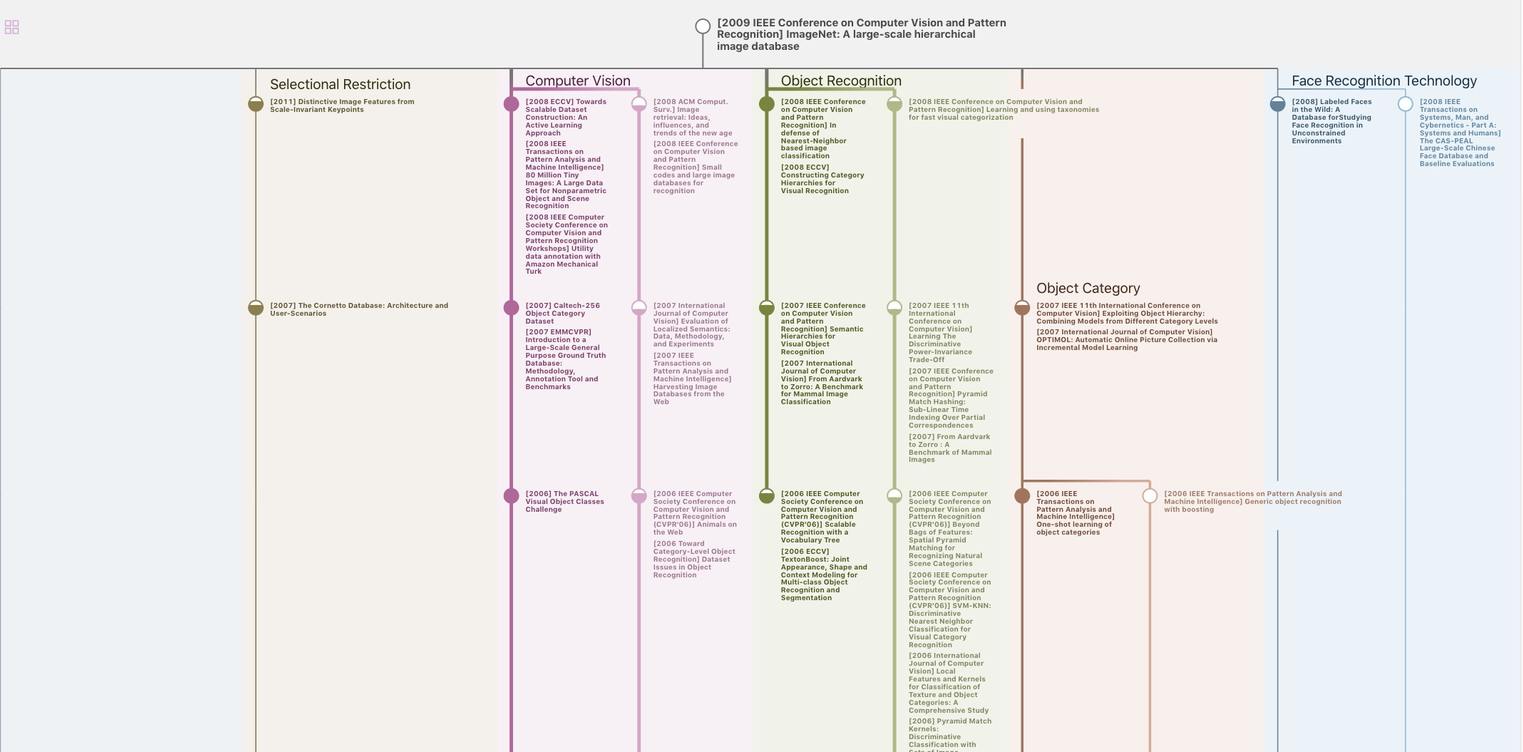
生成溯源树,研究论文发展脉络
Chat Paper
正在生成论文摘要