Minimum variance and range-based k-means optimization algorithm-Rdk-means algorithm
2022 IEEE 6th Information Technology and Mechatronics Engineering Conference (ITOEC)(2022)
摘要
To address the problem that the traditional k-means randomly generates initialized centroids leading to the instability of the algorithm, Rdk-means (Range and density k-means) is proposed to improve the clustering algorithm. The algorithm introduces the concept of global density to select sample points. The centroids are initialized by selecting the sample points with the maximum density and distributed by a certain distance. At the same time, the concept of spatial location is introduced in the process of sample partitioning to filter out the centroids that are closer to each sample point, thus avoiding unnecessary centroid distance calculation and optimizing the shortage of longer clustering time caused by the initialization centroid process. Through experimental validation and analysis, the algorithm has been improved in clustering effect and stability.
更多查看译文
关键词
k-means algorithm,optimization,density,distance,spatial location,clustering speed,Rdk-means algorithm
AI 理解论文
溯源树
样例
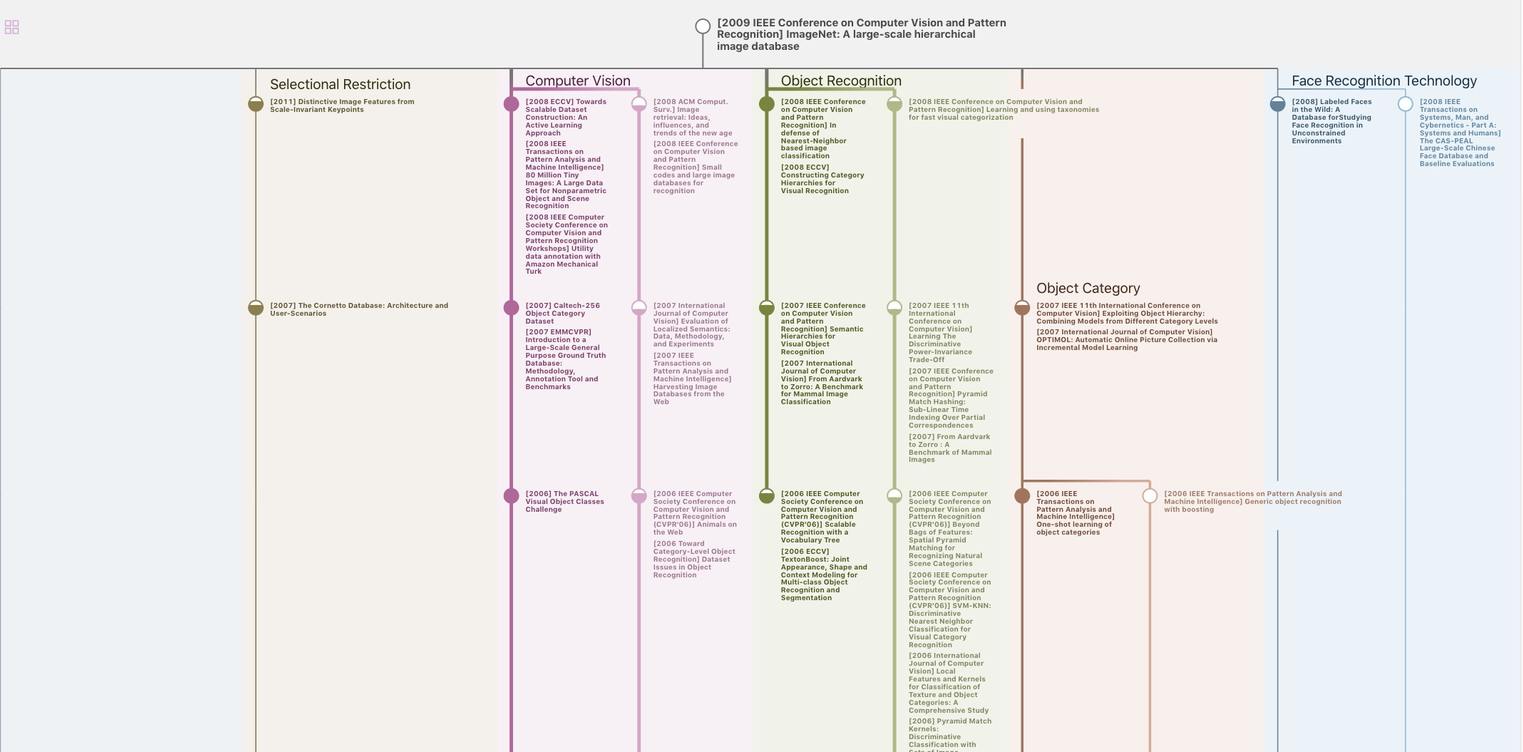
生成溯源树,研究论文发展脉络
Chat Paper
正在生成论文摘要