Semi-supervised machine learning framework for network intrusion detection
The Journal of Supercomputing(2022)
摘要
Network intrusion detection plays an important role as tools for managing and identifying potential threats, which presents various challenges. Redundant features and difficult marking in data cause a long-term problem in network traffic detection. In this paper, we propose a semi-supervised machine learning framework based on multi-strategy feature filtering, principal component analysis (PCA), and an improved Tri-Light Gradient Boosting Machine (Tri-LightGBM) based on stratified sampling. This multi-strategy feature filtering method employing Fisher score and Information gain can select features that have good category discrimination and are more relevant to category labels. After that, we combine PCA to convert multiple features into comprehensive features, which are used as the input of the Tri-LightGBM model. Tri-LightGBM can exploit unlabeled data cooperatively and maintain a large disagreement among the base learners. Moreover, we propose a stratified sampling based on labeled categories to reduce the probability of being selected as the same category during the model update process. Thus, the Tri-LightGBM based on stratified sampling can compensate for the classification error rate caused by the imbalance of the dataset. The semi-supervised machine learning framework is evaluated on two intrusion detection evaluation datasets, namely UNSW-NB15 and CIC-IDS-2017. The evaluation results show that the multi-strategy feature filtering method can increase the accuracy, recall, precision, and F-measure by up to 0.5%, and reduce the false-positive rate by up to 0.5%. Furthermore, the precision rate of minority categories can be increased by about 1–2%.
更多查看译文
关键词
Network intrusion detection,Fisher score,Information gain,PCA,Tri-LightGBM
AI 理解论文
溯源树
样例
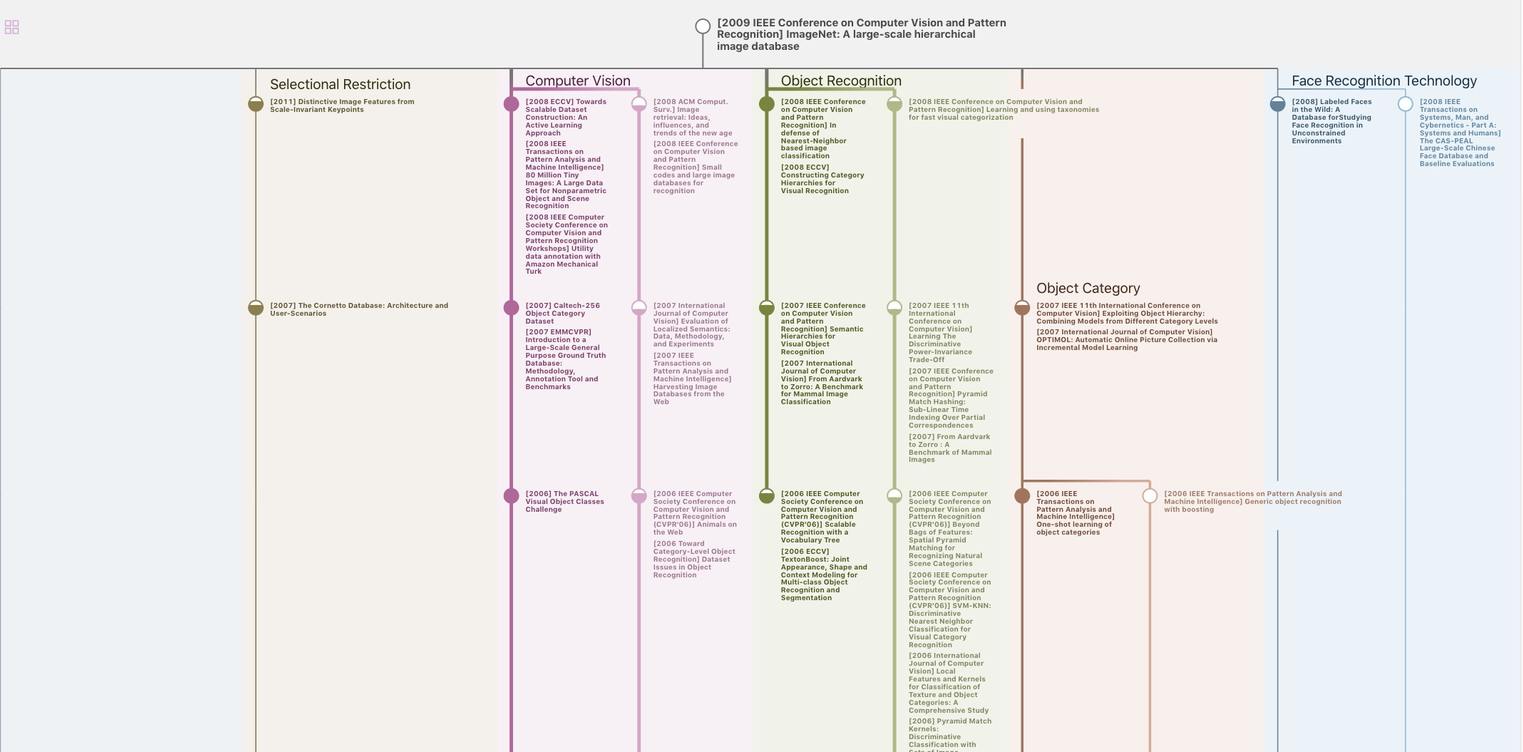
生成溯源树,研究论文发展脉络
Chat Paper
正在生成论文摘要