Receptance coupling substructure analysis and chatter frequency-informed machine learning for milling stability
CIRP Annals(2022)
摘要
This paper describes a milling stability identification approach that simultaneously considers: physics-based models for the tool tip frequency response functions and stability predictions; the binary result from a milling test (automatically labeled as stable or unstable based on frequency content); chatter frequency when an unstable result is obtained; and user risk tolerance. The algorithm applies probabilistic Bayesian machine learning with adaptive, parallelized Markov Chain Monte Carlo sampling to update the probability of stability with each milling test. The result is a robust solution for rapid convergence to optimized milling parameters for maximum metal removal rate using all available information.
更多查看译文
关键词
Milling,Stability,Machine learning
AI 理解论文
溯源树
样例
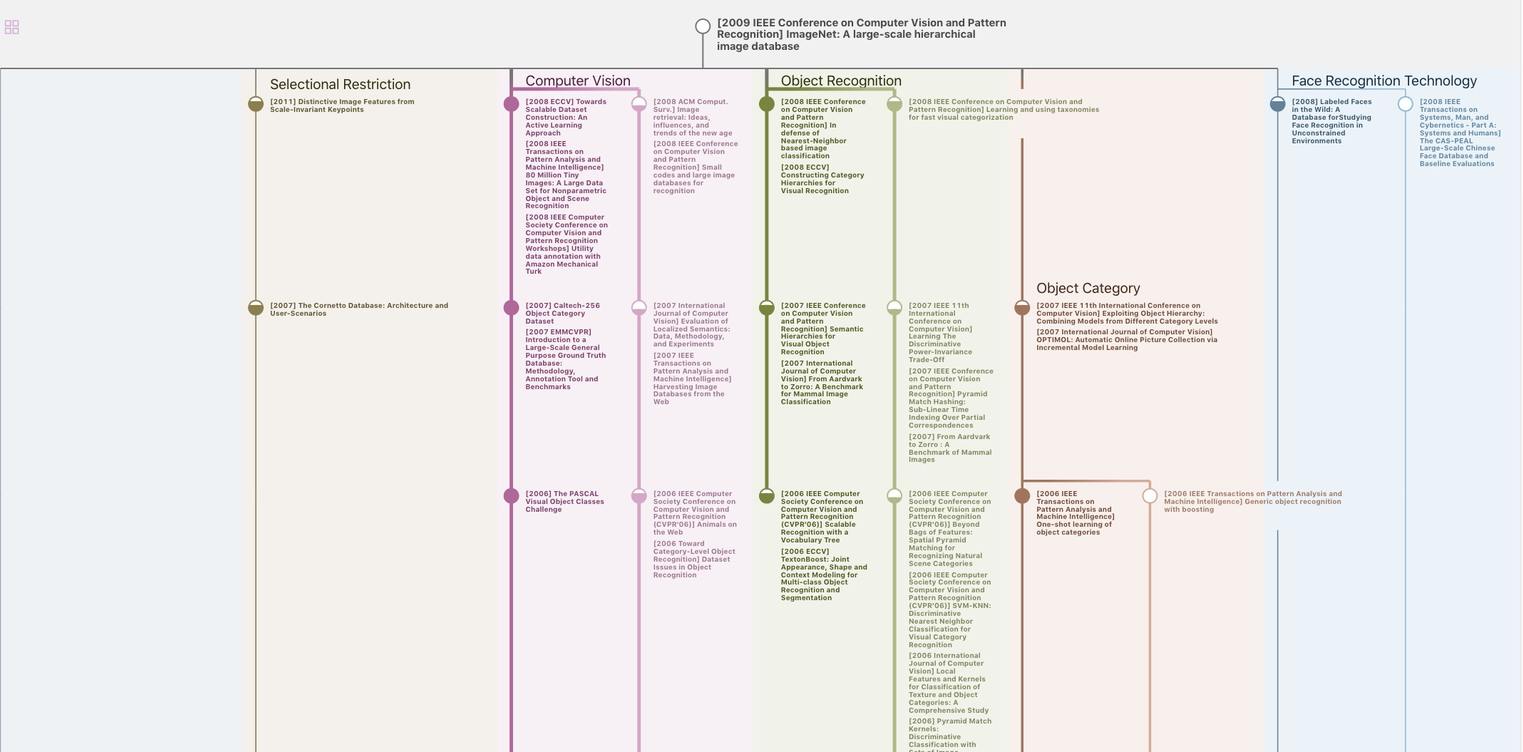
生成溯源树,研究论文发展脉络
Chat Paper
正在生成论文摘要