Evaluation of Dimensionality Reduction Using PCA on EMG-Based Signal Pattern Classification
Engineering Proceedings(2022)
Key words
electromyogram,principal component analysis,KNN,PNN,LVQ,classification rate
AI Read Science
Must-Reading Tree
Example
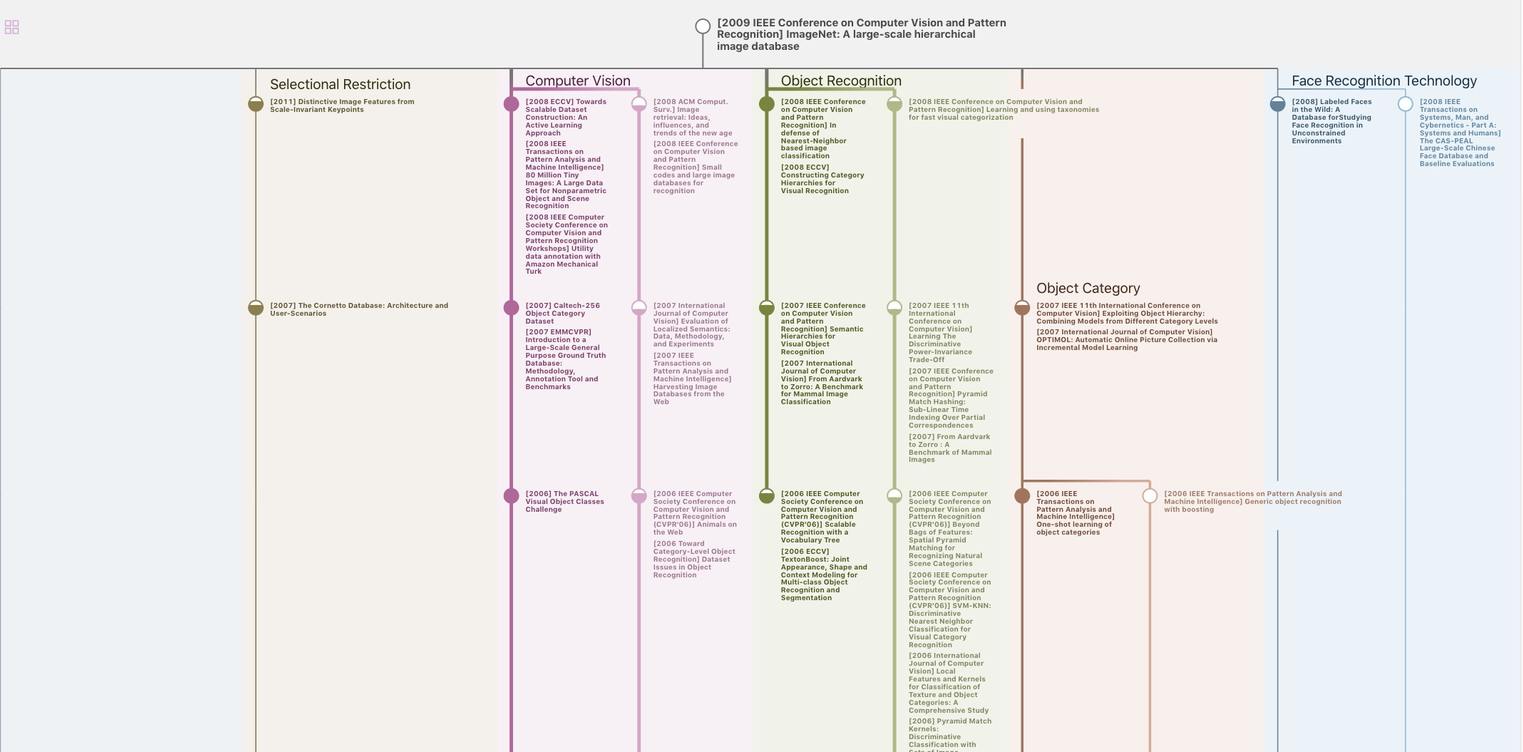
Generate MRT to find the research sequence of this paper
Chat Paper
Summary is being generated by the instructions you defined