One-Shot Tuner for Deep Learning Compilers
CC'22: PROCEEDINGS OF THE 31ST ACM SIGPLAN INTERNATIONAL CONFERENCE ON COMPILER CONSTRUCTION(2022)
Abstract
Auto-tuning DL compilers are gaining ground as an optimizing back-end for DL frameworks. While existing work can generate deep learning models that exceed the performance of hand-tuned libraries, they still suffer from prohibitively long auto-tuning time due to repeated hardware measurements in large search spaces. In this paper, we take a neural-predictor inspired approach to reduce the auto-tuning overhead and show that a performance predictor model trained prior to compilation can produce optimized tensor operation codes without repeated search and hardware measurements. To generate a sample-efficient training dataset, we extend input representation to include task-specific information and to guide data sampling methods to focus on learning high-performing codes. We evaluated the resulting predictor model, One-Shot Tuner, against AutoTVM and other prior work, and the results show that One-Shot Tuner speeds up compilation by 2.81x to 67.7x compared to prior work while providing comparable or improved inference time for CNN and Transformer models.
MoreTranslated text
Key words
optimizing compilers,autotuning,performance models,deep neural networks
AI Read Science
Must-Reading Tree
Example
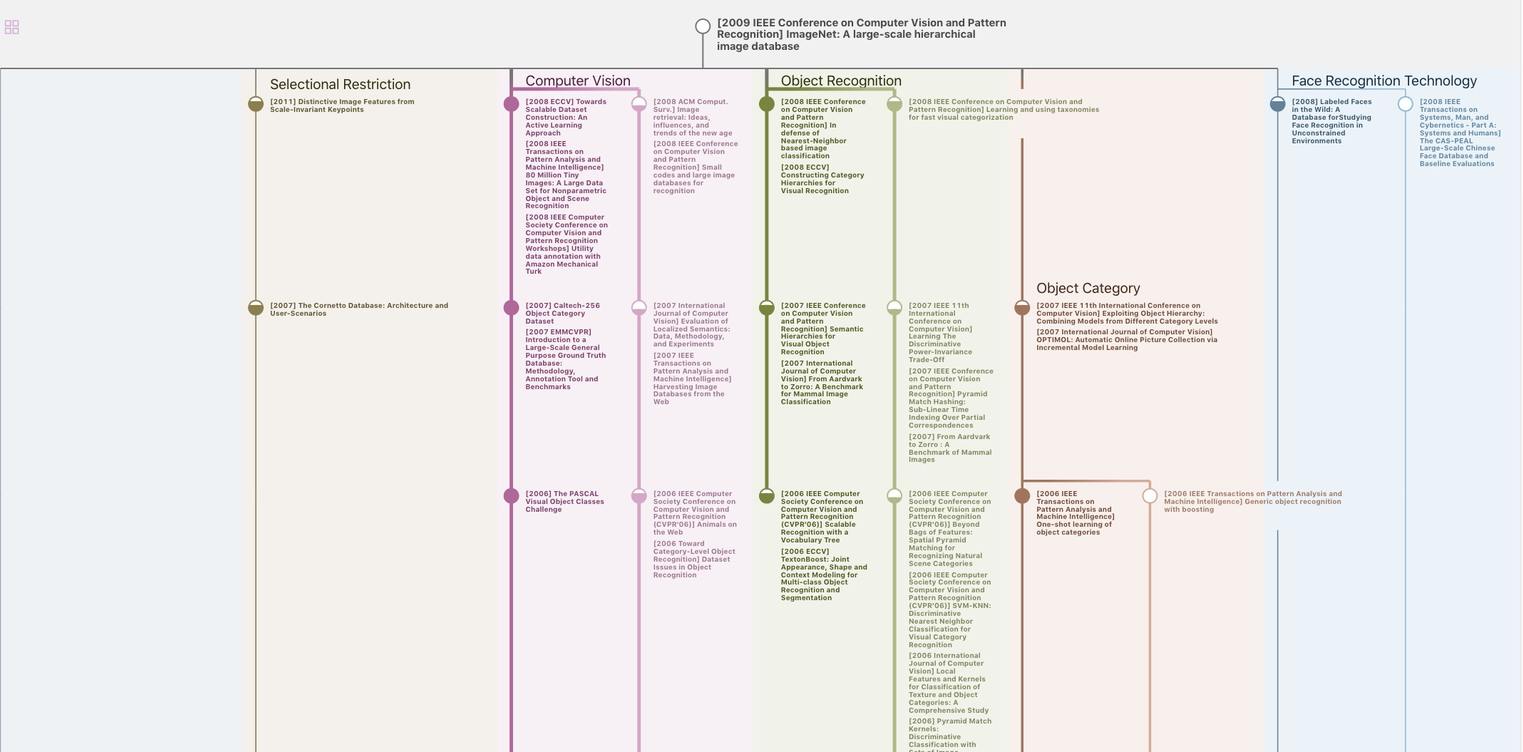
Generate MRT to find the research sequence of this paper
Chat Paper
Summary is being generated by the instructions you defined