Nonlocal-based Tensor-Average-rank Minimization and Tensor Transform-Sparsity for 3D Image Denoising
Knowledge-Based Systems(2022)
Abstract
Three-dimensional (3D) image denoising is an essential problem of low-level computer vision tasks, and it is also a key preprocessing step for subsequent applications. Recently, nonlocal-based models have received increasing attention in the field of 3D image denoising because they are powerful in promoting the redundancy of 3D images. In this paper, we propose a nonlocal-based denoising model combining tensor-average-rank minimization with tensor transform-sparsity (NLRS) to exploit the redundancy of each similar group. In the proposed model, we employ tensor-average-rank minimization to exploit the low-rankness of the underlying clean similar groups. Moreover, we introduce a tensor-based transform learning so that each underlying clean similar group gains sparse representation under the corresponding transform. The low-rank term and the transform-sparsity term are complementary in terms of noise suppression and information preservation. To tackle the nonconvex model, we develop a proximal alternating minimization (PAM) algorithm and theoretically prove the convergence of the algorithm. Numerical experiments on simulated and real data sets are conducted to demonstrate the effectiveness of the proposed method for 3D image denoising.
MoreTranslated text
Key words
Three-dimensional (3D) image denoising,Sparse representation,Tensor-average-rank,Proximal alternating minimization (PAM)
AI Read Science
Must-Reading Tree
Example
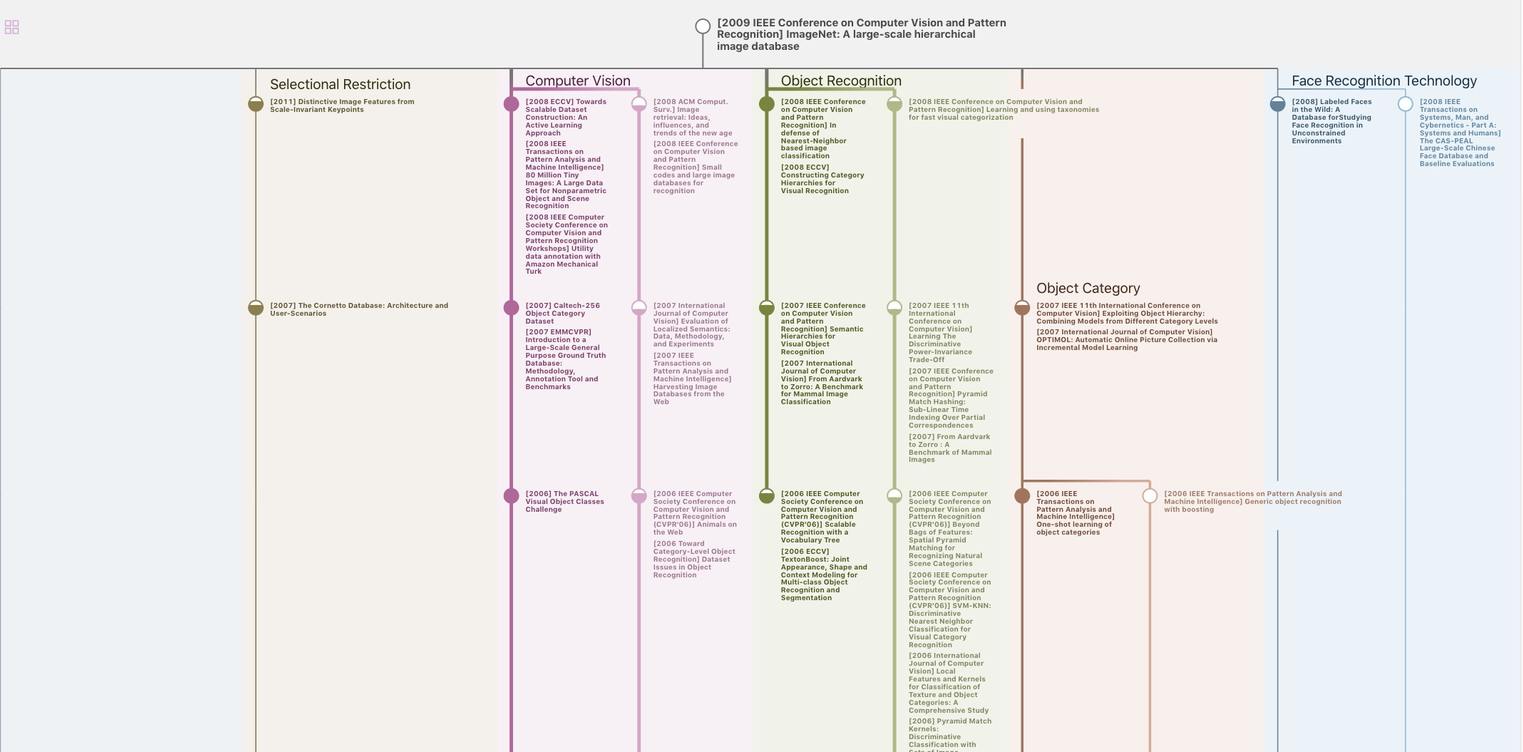
Generate MRT to find the research sequence of this paper
Chat Paper
Summary is being generated by the instructions you defined