Bayesian Prior Learning via Neural Networks for Next-item Recommendation
arxiv(2022)
摘要
Next-item prediction is a a popular problem in the recommender systems domain. As the name suggests, the task is to recommend subsequent items that a user would be interested in given contextual information and historical interaction data. In our paper, we model a general notion of context via a sequence of item interactions. We model the next item prediction problem using the Bayesian framework and capture the probability of appearance of a sequence through the posterior mean of the Beta distribution. We train two neural networks to accurately predict the alpha & beta parameter values of the Beta distribution. Our novel approach of combining black-box style neural networks, known to be suitable for function approximation with Bayesian estimation methods have resulted in an innovative method that outperforms various state-of-the-art baselines. We demonstrate the effectiveness of our method in two real world datasets. Our framework is an important step towards the goal of building privacy preserving recommender systems.
更多查看译文
关键词
neural networks,learning,next-item
AI 理解论文
溯源树
样例
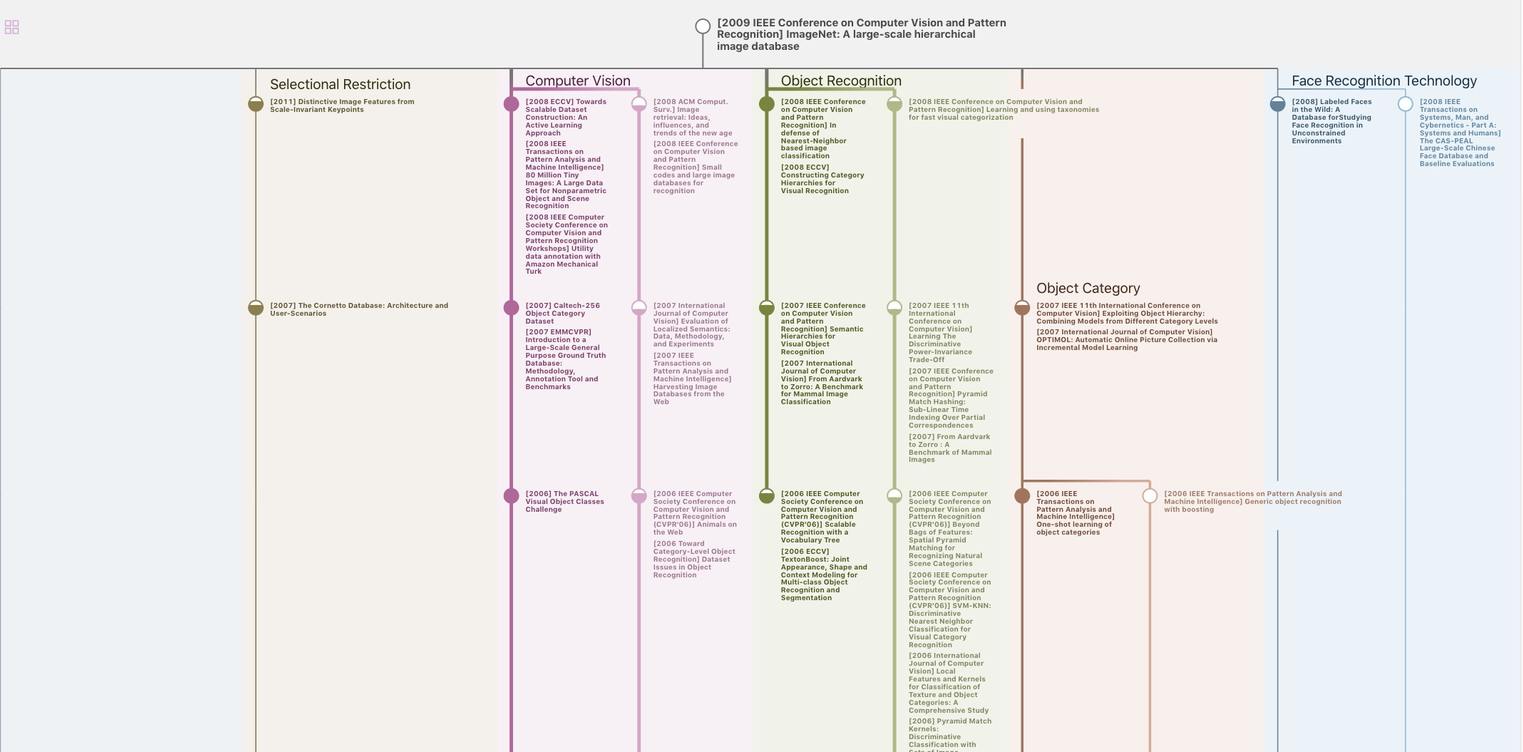
生成溯源树,研究论文发展脉络
Chat Paper
正在生成论文摘要