Robust Data-Driven Output Feedback Control via Bootstrapped Multiplicative Noise
arXiv (Cornell University)(2022)
Abstract
We propose a robust data-driven output feedback control algorithm that explicitly incorporates inherent finite-sample model estimate uncertainties into the control design. The algorithm has three components: (1) a subspace identification nominal model estimator; (2) a bootstrap resampling method that quantifies non-asymptotic variance of the nominal model estimate; and (3) a non-conventional robust control design method comprising a coupled optimal dynamic output feedback filter and controller with multiplicative noise. A key advantage of the proposed approach is that the system identification and robust control design procedures both use stochastic uncertainty representations, so that the actual inherent statistical estimation uncertainty directly aligns with the uncertainty the robust controller is being designed against. Moreover, the control design method accommodates a highly structured uncertainty representation that can capture uncertainty shape more effectively than existing approaches. We show through numerical experiments that the proposed robust data-driven output feedback controller can significantly outperform a certainty equivalent controller on various measures of sample complexity and stability robustness.
MoreTranslated text
AI Read Science
Must-Reading Tree
Example
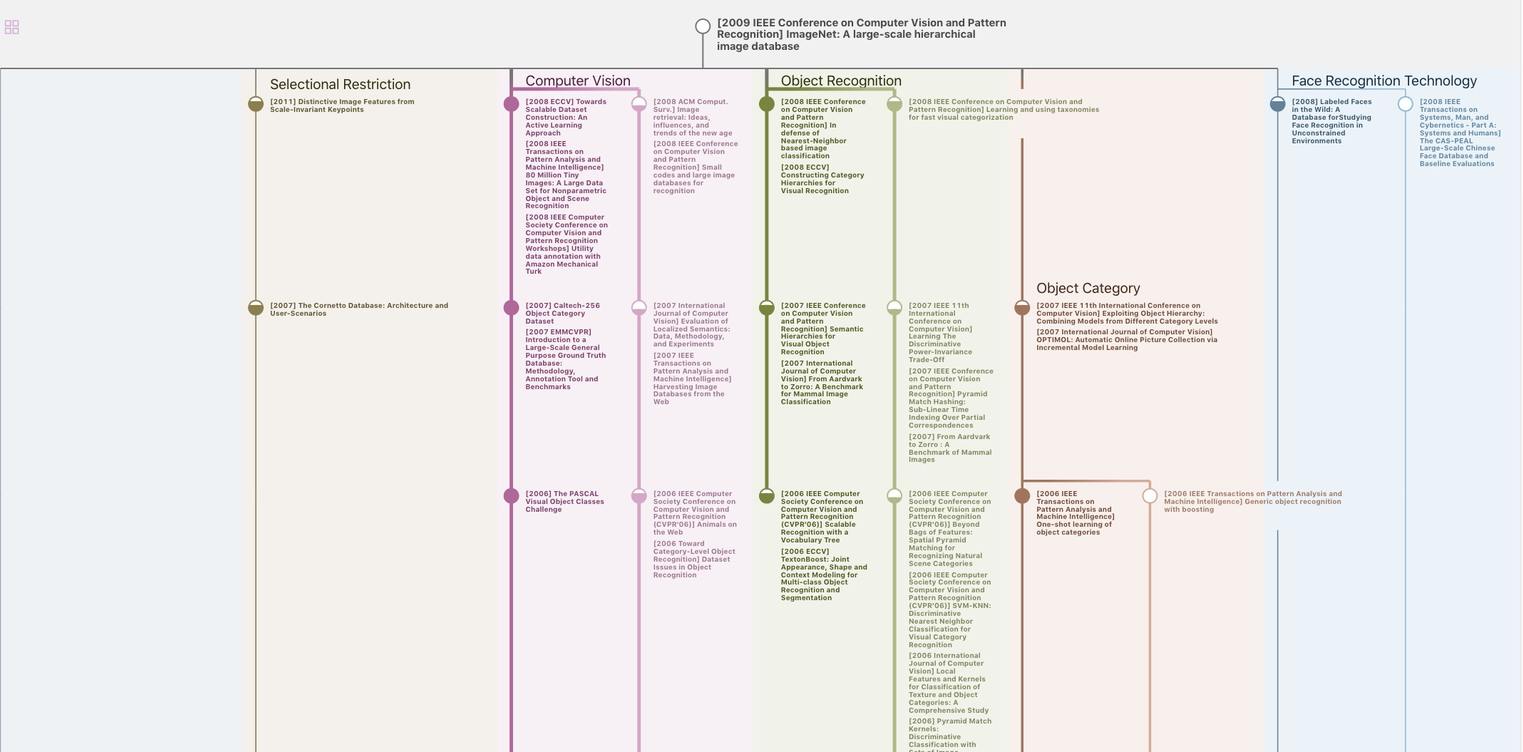
Generate MRT to find the research sequence of this paper
Chat Paper
Summary is being generated by the instructions you defined