Identification of diffusive states in tracking applications using unsupervised deep learning methods
2022 IEEE INTERNATIONAL SYMPOSIUM ON BIOMEDICAL IMAGING (IEEE ISBI 2022)(2022)
摘要
The most widely used method for analysis of diffusive motion in particle tracking is based on estimation of the mean squared displacement (MSD) and subsequently relevant motion parameters. This approach is only valid for a population of particles exhibiting a single type of motion (e.g., super or sub-diffusive). Thus, to deal with trajectories that describe dynamics with switching motion patterns, trajectory segmentation techniques are of major importance. Here, we propose an unsupervised trajectory segmentation technique, which employs the ideas of the state-of-theart image denoising "noise2noise" approach. Using typical single-particle tracking data, our method is capable of unsupervised trajectory segmentation in the most difficult situations (e.g. unknown number of purely diffusive states), and computation of the relevant parameters. The applicability of the method is demonstrated using simulated and real experimental data, showing that its performance is comparable to similar top performing supervised methods.
更多查看译文
关键词
Single-particle tracking, trajectory segmentation, unsupervised learning, recurrent neural networks
AI 理解论文
溯源树
样例
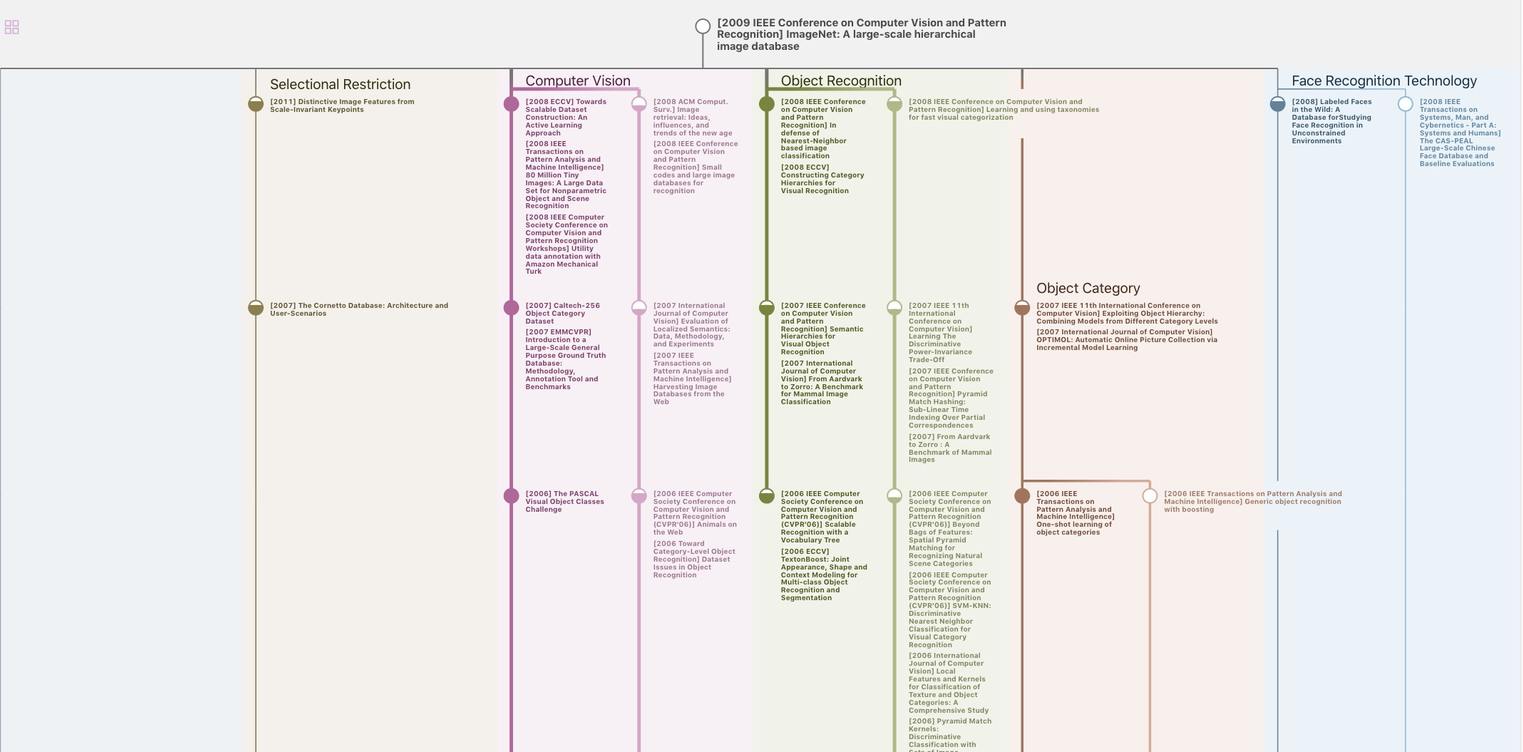
生成溯源树,研究论文发展脉络
Chat Paper
正在生成论文摘要