PGADA: Perturbation-Guided Adversarial Alignment for Few-Shot Learning Under the Support-Query Shift
ADVANCES IN KNOWLEDGE DISCOVERY AND DATA MINING, PAKDD 2022, PT I(2022)
摘要
Few-shot learning methods aim to embed the data to a low-dimensional embedding space and then classify the unseen query data to the seen support set. While these works assume that the support set and the query set lie in the same embedding space, a distribution shift usually occurs between the support set and the query set, i.e., the Support-Query Shift, in the real world. Though optimal transportation has shown convincing results in aligning different distributions, we find that the small perturbations in the images would significantly misguide the optimal transportation and thus degrade the model performance. To relieve the misalignment, we first propose a novel adversarial data augmentation method, namely Perturbation-Guided Adversarial Alignment (PGADA), which generates the hard examples in a self-supervised manner. In addition, we introduce Regularized Optimal Transportation to derive a smooth optimal transportation plan. Extensive experiments on three benchmark datasets manifest that our framework significantly outperforms the eleven state-of-the-art methods on three datasets.
更多查看译文
关键词
Few-shot learning, Adversarial data augmentation, Optimal transportation
AI 理解论文
溯源树
样例
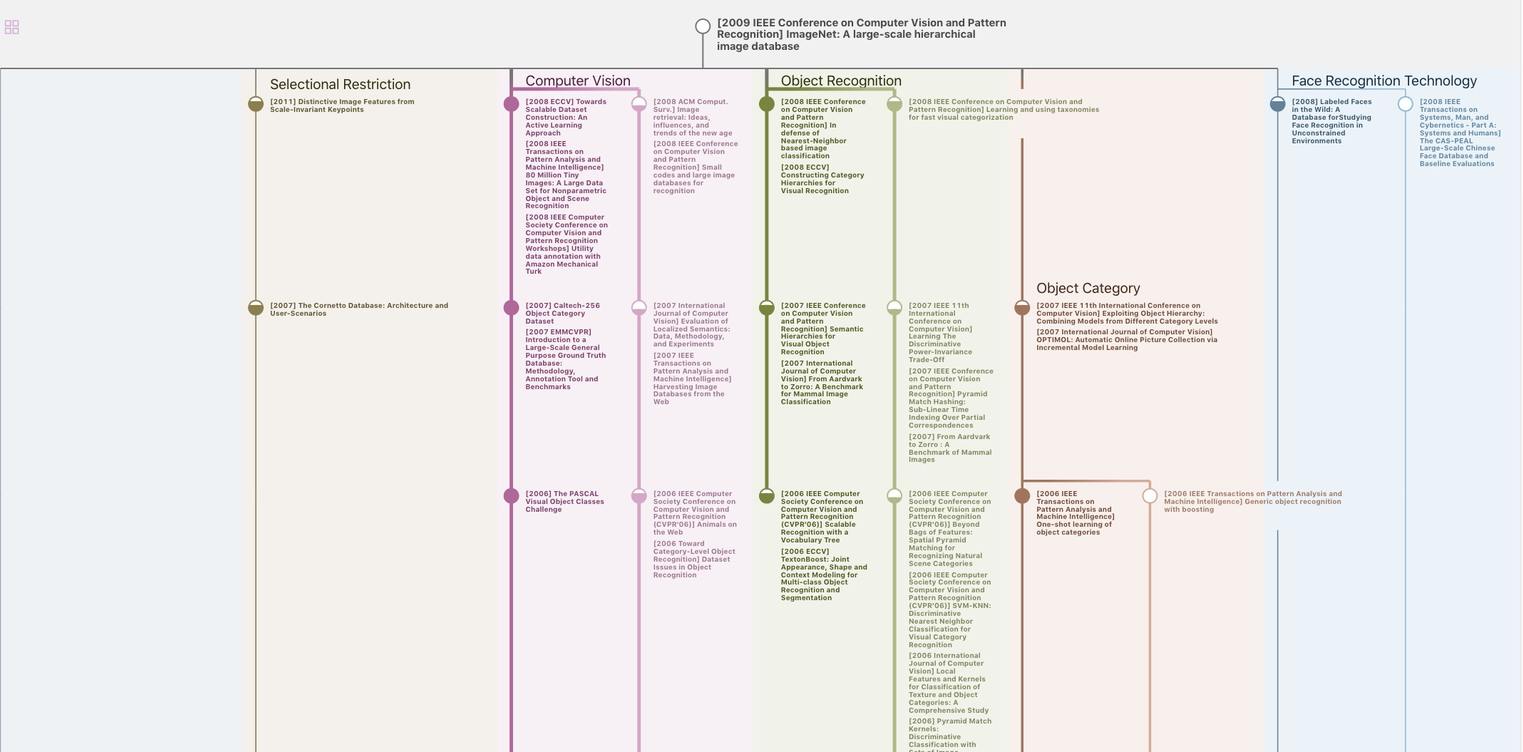
生成溯源树,研究论文发展脉络
Chat Paper
正在生成论文摘要