Description of collective magnetization processes with machine learning models
arxiv(2022)
摘要
This work introduces a latent space method to calculate the demagnetization reversal process of multigrain permanent magnets. The algorithm consists of two deep learning models based on neural networks. The embedded Stoner-Wohlfarth method is used as a reduced order model for computing samples to train and test the machine learning approach. The work is a proof of concept of the method since the used microstructures are simple and the only varying parameters are the magnetic anisotropy axes of the hard magnetic grains. A convolutional autoencoder is used for nonlinear dimensionality reduction, in order to reduce the required amount of training samples for predicting the hysteresis loops. We enriched the loss function with physical information about the underlying problem which increases the accuracy of the machine learning approach. A deep learning regressor is operating in the latent space of the autoencoder. The predictor takes a series of previously encoded magnetization states and outputs the next magnetization state along the hysteresis curve. To predict the complete demagnetization loop, we apply a recursive learning scheme.
更多查看译文
关键词
collective magnetization,machine learning
AI 理解论文
溯源树
样例
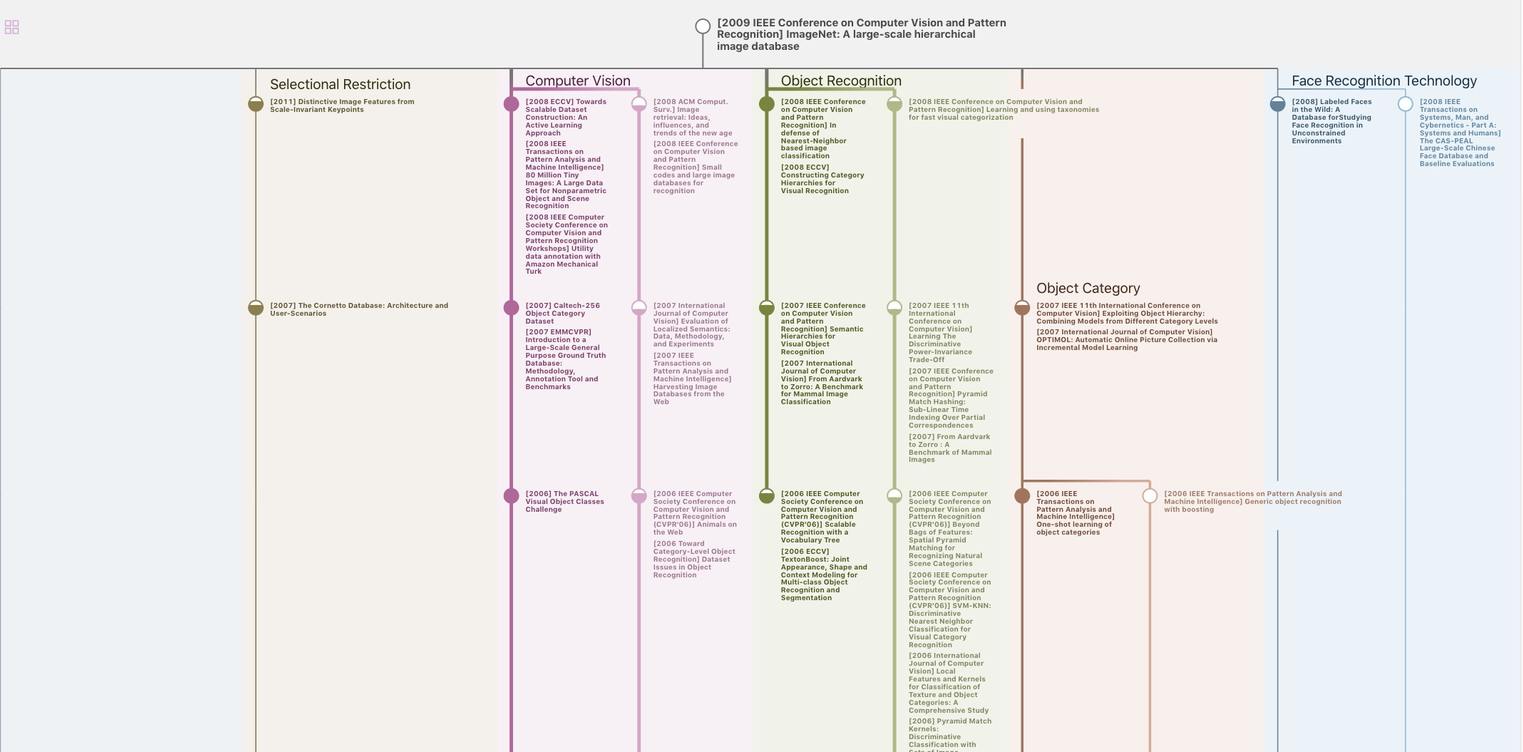
生成溯源树,研究论文发展脉络
Chat Paper
正在生成论文摘要