Adversarial confound regression and uncertainty measurements to classify heterogeneous clinical MRI in Mass General Brigham
arxiv(2022)
摘要
Automated disease detection in neuroimaging holds promise to improve the diagnostic ability of radiologists, but routinely collected clinical data frequently contains technical and demographic confounding factors that cause data to both differ between sites and be systematically associated with the disease of interest, thus negatively affecting the robustness of diagnostic models. There is a critical need for diagnostic deep learning models that can train on such imbalanced datasets without being influenced by these confounds. In this work, we introduce a novel deep learning architecture, MUCRAN (Multi-Confound Regression Adversarial Network), to train a deep learning model on clinical brain MRI while regressing demographic and technical confounding factors. We trained MUCRAN using 17,076 clinical T1 Axial brain MRIs collected from Massachusetts General Hospital before 2019 and demonstrated that MUCRAN could successfully regress major confounding factors in the vast clinical data. We also applied a method for quantifying uncertainty across an ensemble of these models to automatically exclude out-of-distribution data in the AD detection. By combining MUCRAN and the uncertainty quantification method, we showed consistent and significant increases in the AD detection accuracy for newly collected MGH data (post-2019) and for data from other hospitals. MUCRAN offers a generalizable approach for heterogenous clinical data for deep-learning-based automatic disease detection.
更多查看译文
关键词
heterogeneous clinical mri,adversarial confound regression,uncertainty measurements
AI 理解论文
溯源树
样例
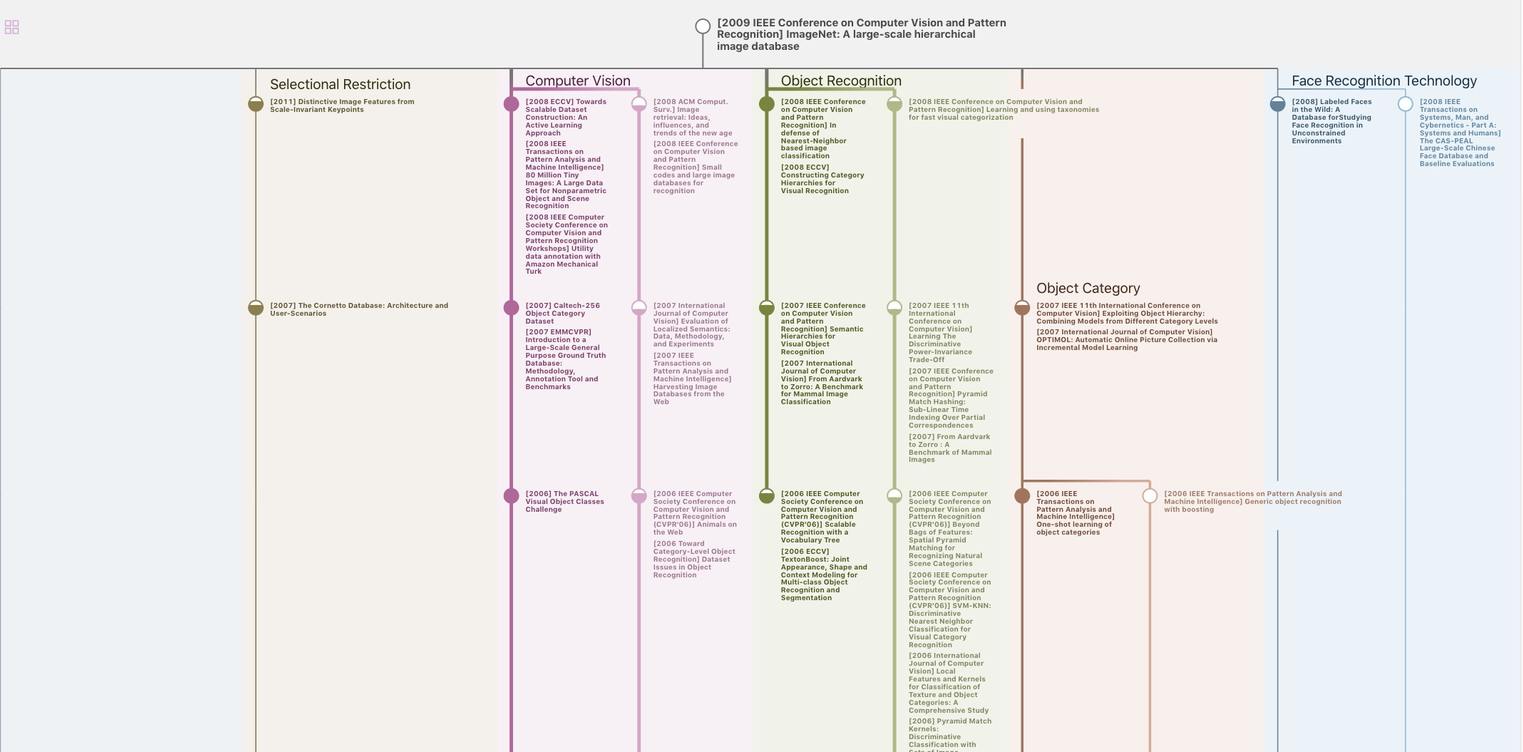
生成溯源树,研究论文发展脉络
Chat Paper
正在生成论文摘要