MS-ML-SNYOLOv3: A robust lightweight modification of SqueezeNet based YOLOv3 for pedestrian detection
Optik(2022)
摘要
Pedestrian detection is a vital problem area in computer vision applications such as surveillance, automotive safety, and robotics. To continue the progress made in this field, a deep learning based approach is given in this work. A modified lightweight YOLOv3 is proposed, which is named as MultiScale-MultiLevel-SqueezeNetYOLOv3 (MS-ML-SNYOLOv3). An improved base network forms the basis of the proposed YOLOv3. Two Squeeze & Expand blocks are embodied in SqueezeNet at specific levels of the network to extract a hierarchical feature representation. An addition of a larger receptive field is made in the Expand section as well to encapsulate the feature information at various scales. The proposed method is compared against state-of-the-art detection methods, Faster RCNN, YOLOv2, YOLOv3, and SSD. The comparison also includes various base networks for YOLOv2 and YOLOv3. The performance metrics used for comparison are Detection Error Trade-Off, and Precision-Recall Curve. The proposed method is evaluated on benchmark datasets INRIA and Caltech Pedestrian datasets. A statistical analysis, Friedman Test is conducted to verify the application of MS-ML-SNYOLOv3 in real world data.
更多查看译文
关键词
Detection,Receptive field,Squeeze and expand
AI 理解论文
溯源树
样例
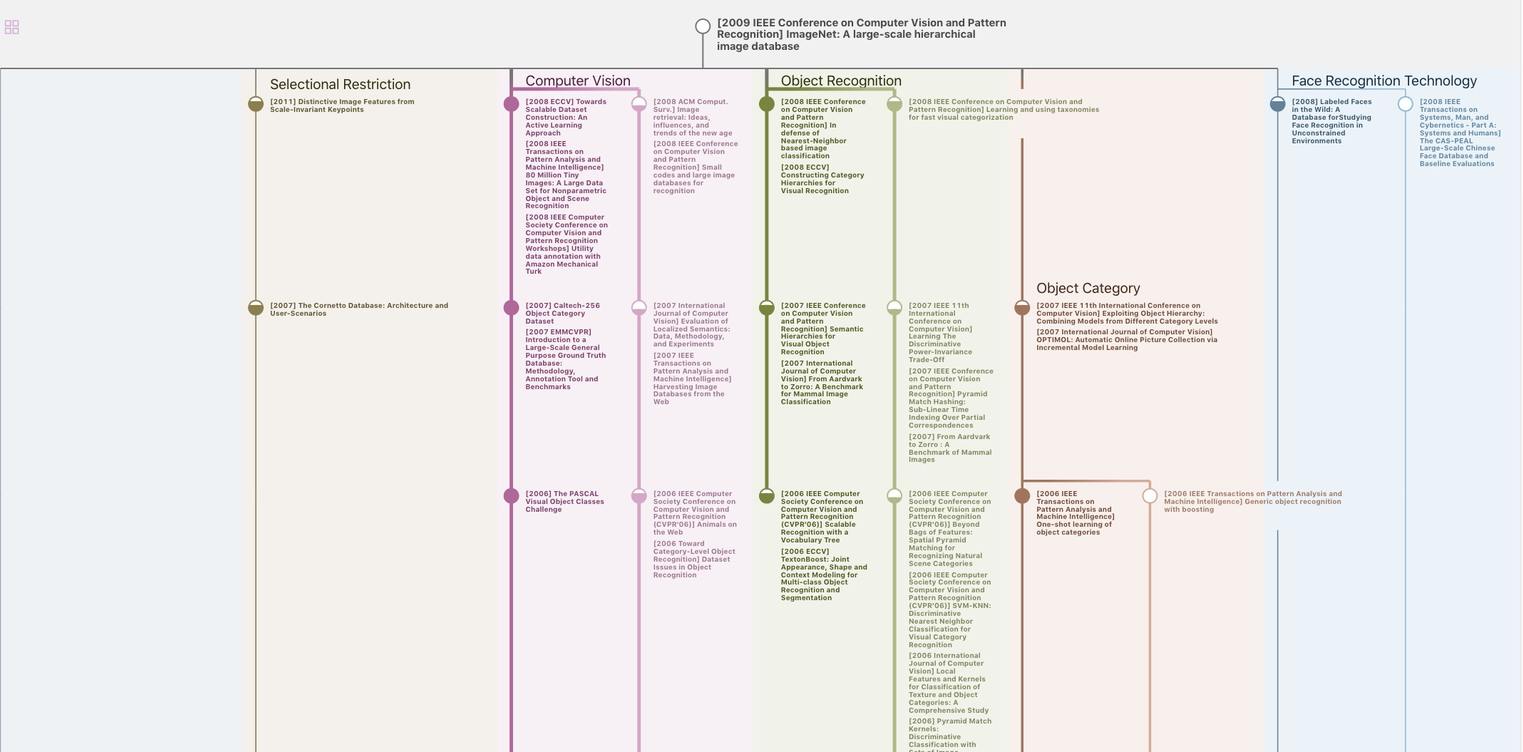
生成溯源树,研究论文发展脉络
Chat Paper
正在生成论文摘要