Towards Preserving Server-Side Privacy of On-Device Models
COMPANION PROCEEDINGS OF THE WEB CONFERENCE 2022, WWW 2022 COMPANION(2022)
Abstract
Machine learning-based predictions are popular in many applications including healthcare, recommender systems and finance. More recently, the development of low-end edge hardware (e.g., Apple's Neural Engine and Intel's Movidius VPU) has provided a path for the proliferation of machine learning on the edge with on-device modeling. Modeling on the device reduces latency and helps maintain the user's privacy. However, on-device modeling can leak private server-side information. In this work, we investigate ondevice machine learning models that are used to provide a service and propose novel privacy attacks that can leak sensitive proprietary information of the service provider. We demonstrate that different adversaries can easily exploit such models to maximize their profit and accomplish content theft. Motivated by the need to preserve both client and server privacy, we present preliminary ideas on thwarting such attacks.
MoreTranslated text
Key words
privacy,machine learning,on-device models,distributed systems,personalization
AI Read Science
Must-Reading Tree
Example
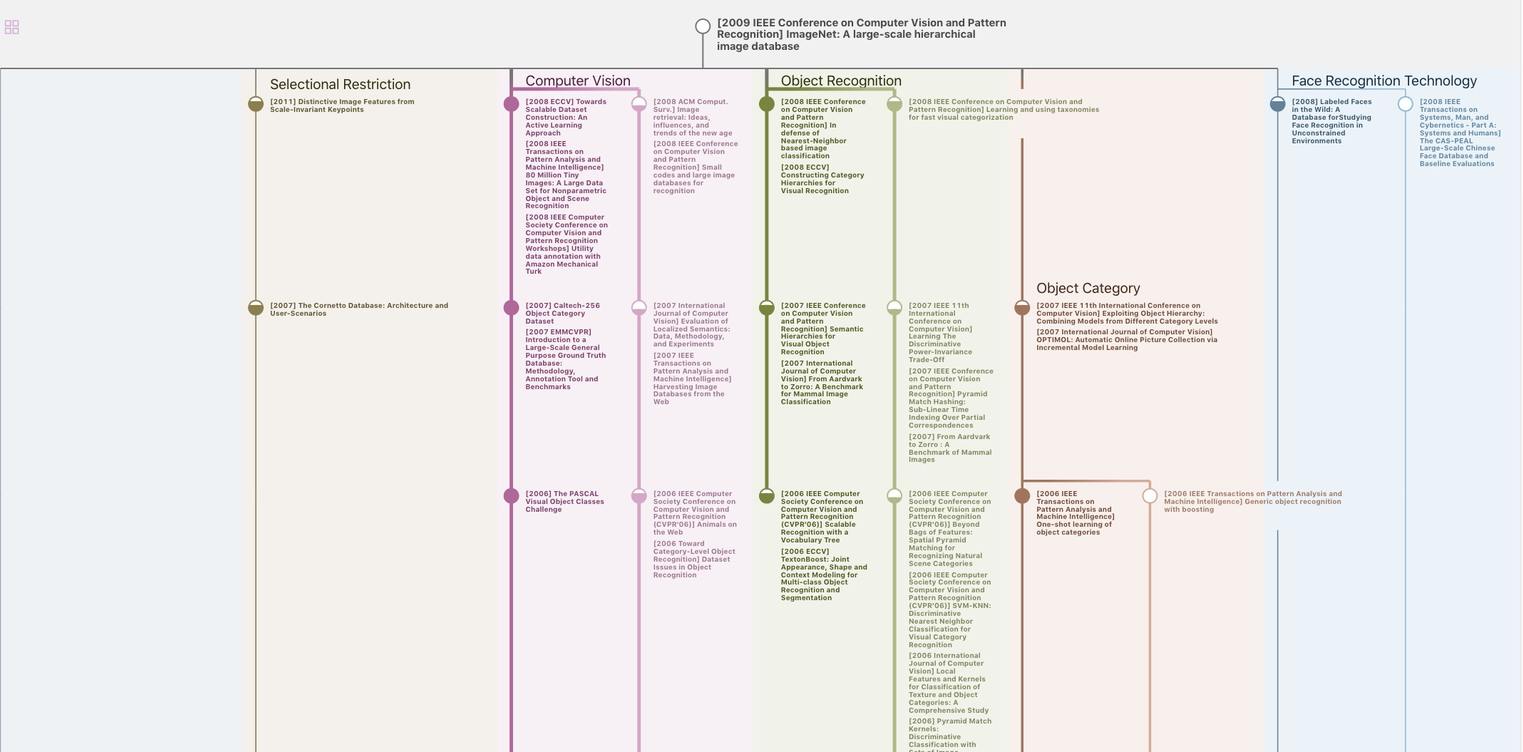
Generate MRT to find the research sequence of this paper
Chat Paper
Summary is being generated by the instructions you defined