Multi-task GNN for Substitute Identification.
International Workshop on Multimodal Human Understanding for the Web and Social Media(2022)
摘要
Substitute product recommendation is important to improve customer satisfaction on E-commerce domain. E-commerce in nature provides rich sources of substitute relationships, e.g., customers purchase a substitute product when the viewed product is sold out, etc. However, existing recommendation systems usually learn the product substitution correlations without jointly considering variant customer behavior sources. In this paper, we propose a unified multi-task heterogeneous graph neural network (M-HetSage), which captures the complementary information across various customer behavior data sources. This allows us to explore synergy across sources with different attributes and quality. Moreover, we introduce a list-aware average precision (LaAP) loss, which exploits correlations among lists of substitutes and non-substitutes by directly optimizing an approximation of the target ranking metric. On top of that, LaAP leverages a list-aware attention mechanism to differentiate substitute qualities for better recommendations. Comprehensive experiments on Amazon proprietary datasets demonstrate the superiority of our proposed M-HetSage framework equipped with LaAP loss, showing 33%+ improvements on NDCG and mAP metrics comparing to traditional HetSage optimized by a single Triplet loss without differentiating customer behavior data sources.
更多查看译文
AI 理解论文
溯源树
样例
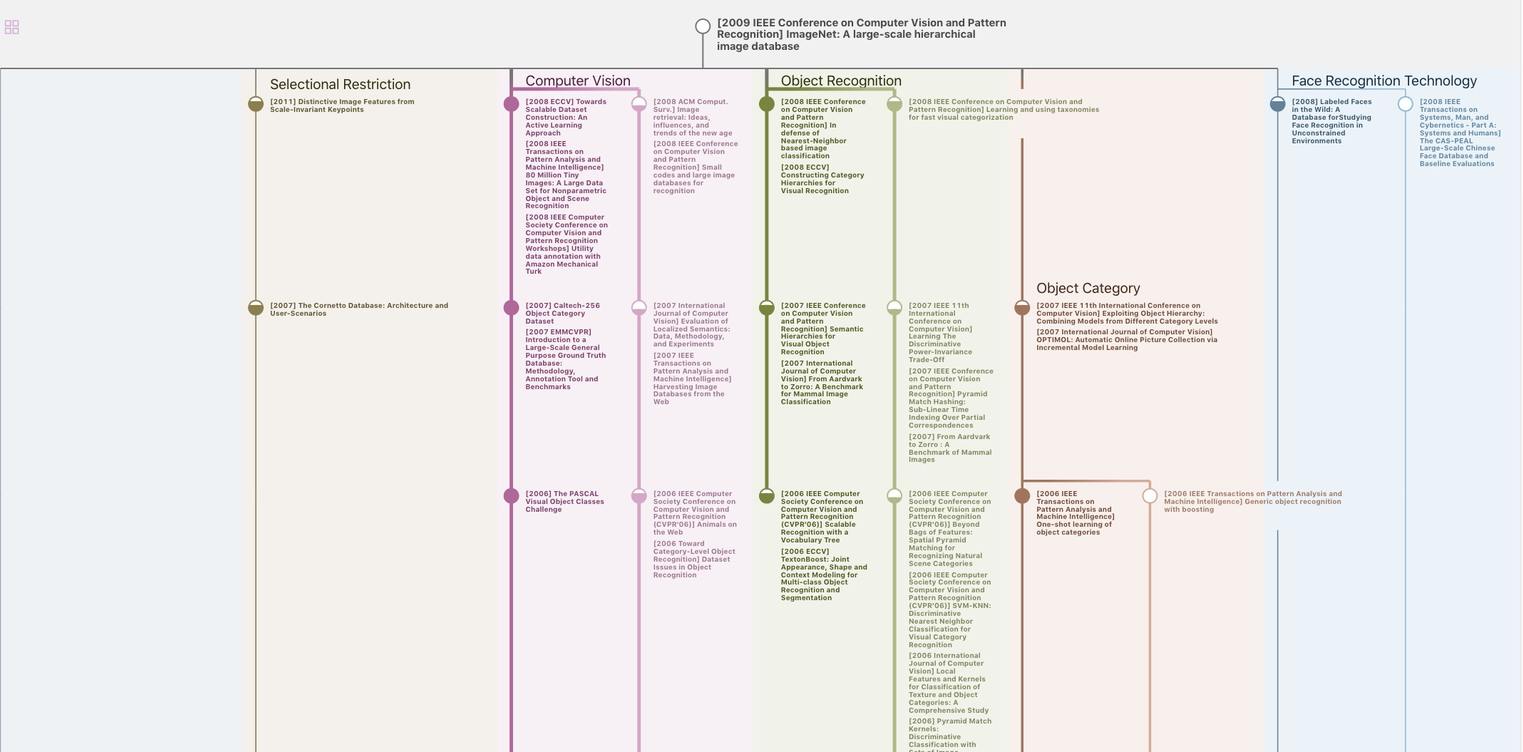
生成溯源树,研究论文发展脉络
Chat Paper
正在生成论文摘要