A distribution-free, Bayesian goodness-of-fit method for assessing similar scientific prediction equations
Journal of Mathematical Psychology(2022)
摘要
In this paper a new Bayesian distribution-free goodness-of-fit method is described. Unlike other goodness-of-fit tests, the new analysis does not examine the statistical structure of the residuals; rather it is based on an examination of the concordance or discordance of the experimental observations from the expectations of the scientific theory. The novel goodness-of-fit procedure is used to assess four highly similar mathematical theories for the probability weighting function from the risky choice literature. Prior research in mathematical psychology and behavioral economics has demonstrated the importance of a nonlinear weighting function for the probabilities that are associated with risky choice, but it has been a challenge to discriminate among a set of different nonlinear functions. However, a reanalysis of the data from a study by Barch and Chechile (2016) with the new goodness-of-fit procedure shows that each of the previously established leading models are sharply discriminated from one another. This analysis is thus an example of how scientific theories, which propose a specific mathematical function, can be assessed with the Bayesian distribution-free goodness-of-fit test. The method is quite general, and it can also be used to assess scientific models that predict multivariate outcomes.
更多查看译文
关键词
Goodness-of-fit,Testing prediction equations,Bayesian goodness-of-fit,Distribution-free statistics
AI 理解论文
溯源树
样例
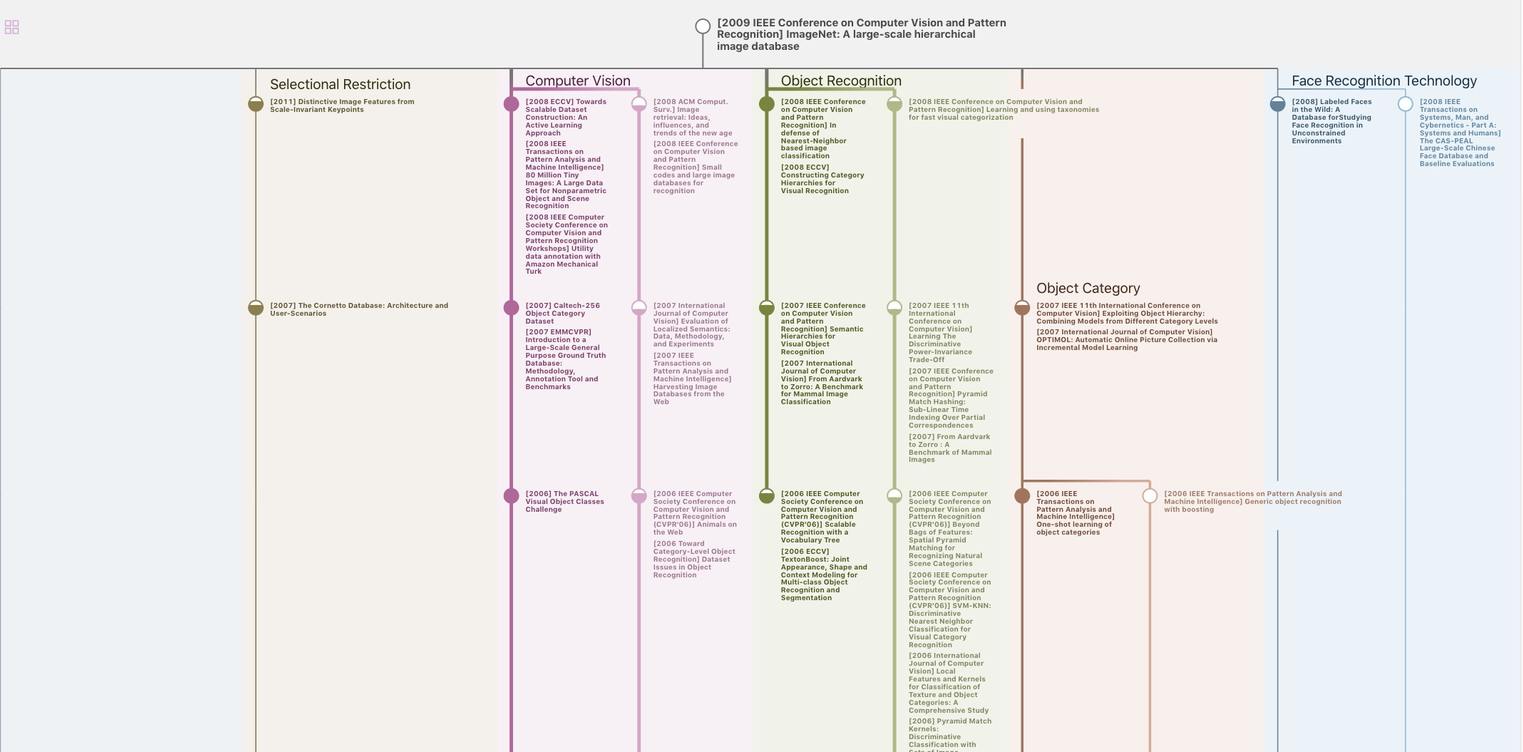
生成溯源树,研究论文发展脉络
Chat Paper
正在生成论文摘要