Comparison of machine learning techniques for predicting porosity of chalk
Journal of Petroleum Science and Engineering(2022)
摘要
Precise and fast estimation of porosity is a vital element of reservoir characterization. A new technology for fast and reliable porosity prediction of chalk samples is presented by applying machine learning methods and X-ray fluorescence (XRF) elemental analysis. Input parameters of prediction models are based on rapid and accurate elemental analysis of chalk samples obtained from Hand-held X-ray fluorescence (HH-XRF) measurements. The intelligent models, including Random Forest (RF), Multilayer perceptron (MLP), Random Forest integrated by Genetic Algorithm (GA-RF) and Multilayer Perceptron integrated by Genetic Algorithm (GA-MLP), are trained and tested based on samples consisting of outcrop chalk samples from Rørdal and Stevns Klint (ST) and core samples from Ekofisk Formation in the North Sea. Results are evaluated by sustainability index (SI), determination coefficient (R2), correlation coefficient (CC), and Willmott's Index of agreement (WI). Results indicate that the combination of GA-RF intelligent method with XRF elemental analysis successfully provides an accurate model by 0.99, 0.02, 0.995 and 0.99 respectively for CC, SI, WI and R2, respectively.
更多查看译文
关键词
Porosity,Chalk,Hand-held X-ray fluorescence,Random forest,Multilayer perceptron,Random forest optimized by genetic algorithm,Multilayer perceptron optimized by genetic algorithm
AI 理解论文
溯源树
样例
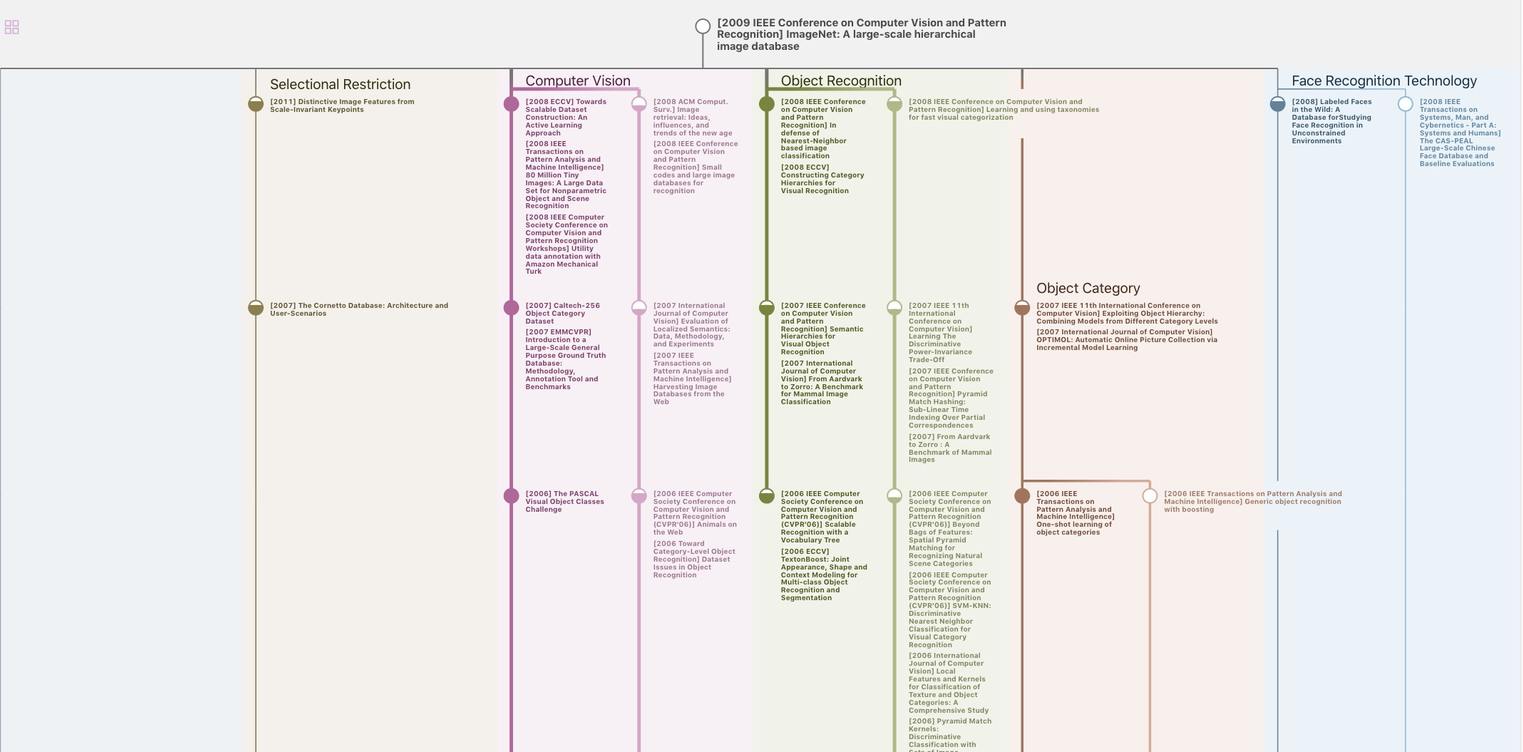
生成溯源树,研究论文发展脉络
Chat Paper
正在生成论文摘要