A deep learning approach to fast radiative transfer
Journal of Quantitative Spectroscopy and Radiative Transfer(2022)
Abstract
Due to the sheer volume of data, leveraging satellite instrument observations effectively in a data assimilation context for numerical weather prediction or for remote sensing requires a radiative transfer model as an observation operator that is both fast and accurate at the same time. Physics-based line-by-line radiative transfer (RT) models fulfil the requirement for accuracy, but are too slow and too costly in computational terms for operational applications. Therefore, fast methods were developed to be able to perform fast RT calculations using techniques such as spectral sampling or pre-computed look-up tables. The operational fast models currently calculate the absorption and scattering coefficients from the pre-computed regression coefficients and atmospheric state and cloud profiles. As a novel solution to this problem, this work investigates a deep learning approach to replace the regression coefficients in the fast RT models. A selection of hidden-layer neural network configurations is trained against atmospheric transmittance profile data computed by an accurate line-by-line model and their performance is evaluated and their advantages and disadvantages are discussed.
MoreTranslated text
Key words
Machine learning,Deep learning,Radiative transfer,Infrared radiation,Transmittance
AI Read Science
Must-Reading Tree
Example
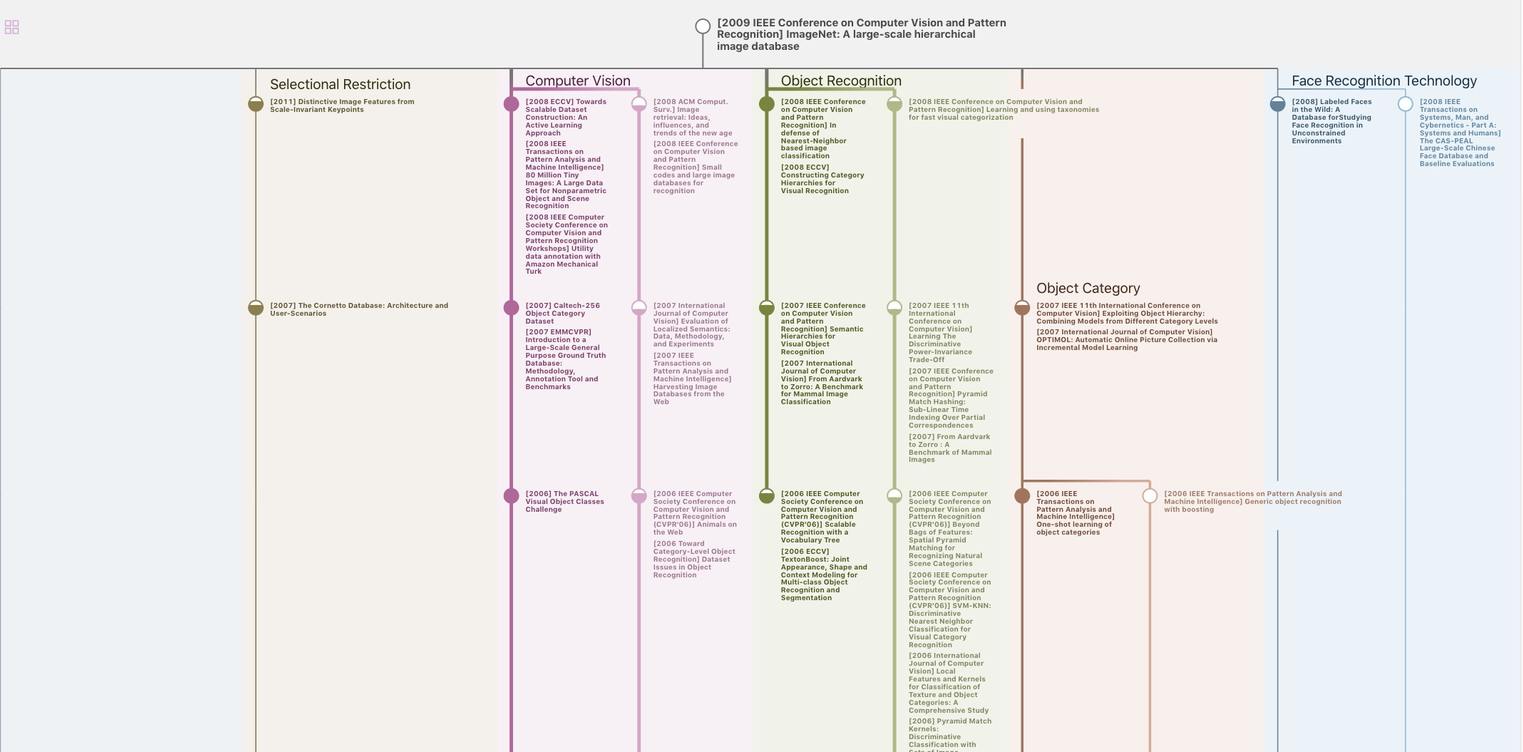
Generate MRT to find the research sequence of this paper
Chat Paper
Summary is being generated by the instructions you defined