Divide-and-Conquer: Post-User Interaction Network for Fake News Detection on Social Media
International World Wide Web Conference(2022)
Abstract
ABSTRACTFake News detection has attracted much attention in recent years. Social context based detection methods attempt to model the spreading patterns of fake news by utilizing the collective wisdom from users on social media. This task is challenging for three reasons: (1) There are multiple types of entities and relations in social context, requiring methods to effectively model the heterogeneity. (2) The emergence of news in novel topics in social media causes distribution shifts, which can significantly degrade the performance of fake news detectors. (3) Existing fake news datasets usually lack of great scale, topic diversity and user social relations, impeding the development of this field. To solve these problems, we formulate social context based fake news detection as a heterogeneous graph classification problem, and propose a fake news detection model named Post-User Interaction Network (PSIN), which adopts a divide-and-conquer strategy to model the post-post, user-user and post-user interactions in social context effectively while maintaining their intrinsic characteristics. Moreover,we adopt an adversarial topic discriminator for topic-agnostic feature learning, in order to improve the generalizability of our method for new-emerging topics. Furthermore, we curate a new dataset for fake news detection, which contains over 27,155 news from 5 topics, 5 million posts, 2 million users and their induced social graph with 0.2 billion edges. It has been published on https://github.com/qwerfdsaplking/MC-Fake. Extensive experiments illustrate that our method outperforms SOTA baselines in both in-topic and out-of-topic settings.
MoreTranslated text
Key words
Fake News Detection, Social Media, Graph Neural Network
AI Read Science
Must-Reading Tree
Example
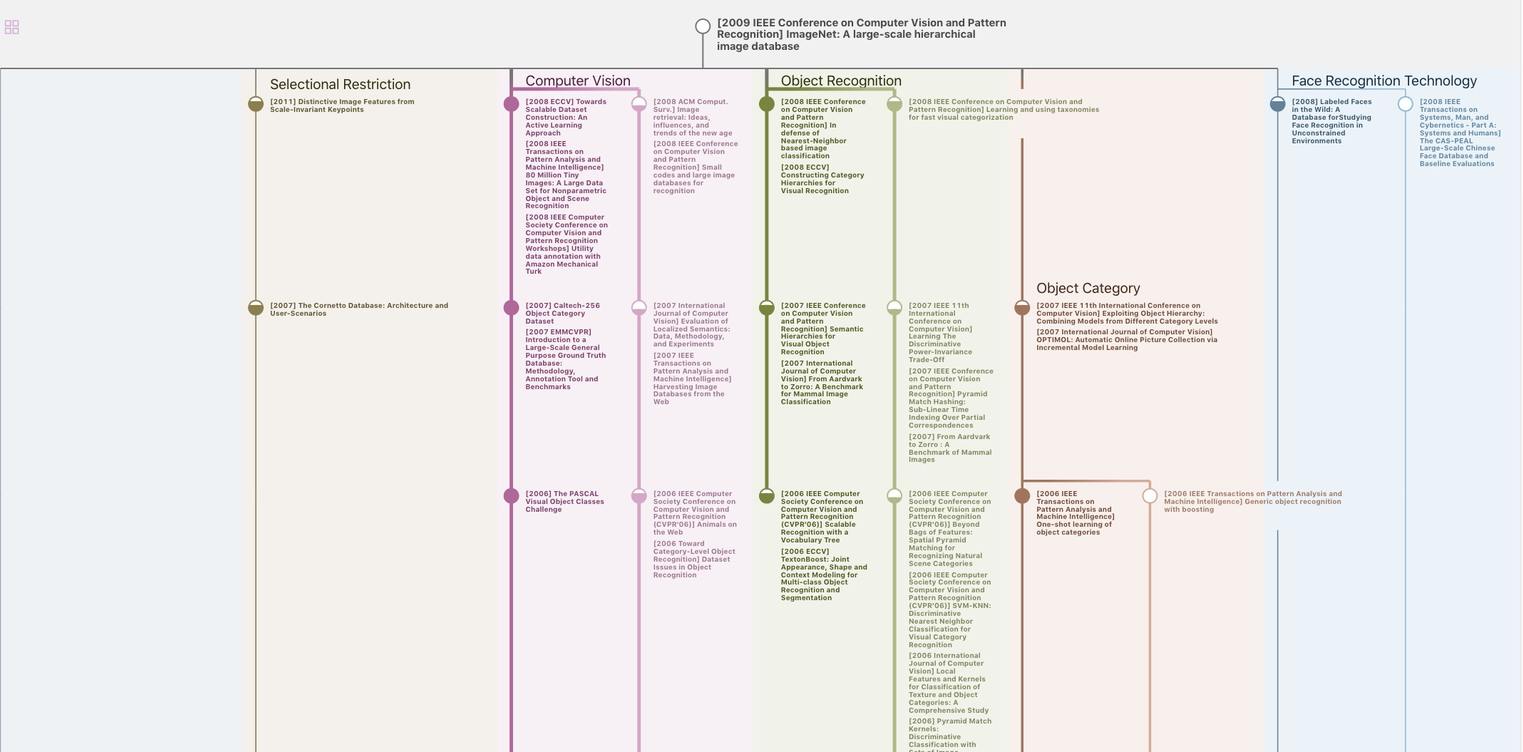
Generate MRT to find the research sequence of this paper
Chat Paper
Summary is being generated by the instructions you defined