Modality Matches Modality: Pretraining Modality-Disentangled Item Representations for Recommendation
International World Wide Web Conference(2022)
摘要
ABSTRACT Recent works have shown the effectiveness of incorporating textual and visual information to tackle the sparsity problem in recommendation scenarios. To fuse these useful heterogeneous modality information, an essential prerequisite is to align these information for modality-robust features learning and semantic understanding. Unfortunately, existing works mainly focus on tackling the learning of common knowledge across modalities, while the specific characteristics of each modality is discarded, which may inevitably degrade the recommendation performance. To this end, we propose a pretraining framework PAMD, which stands for PretrAining Modality-Disentangled Representations Model. Specifically, PAMD utilizes pretrained VGG19 and Glove to embed items’ both visual and textual modalities into the continuous embedding space. Based on these primitive heterogeneous representations, a disentangled encoder is devised to automatically extract their modality-common characteristics while preserving their modality-specific characteristics. After this, a contrastive learning is further designed to guarantee the consistence and gaps between modality-disentangled representations. To the best of our knowledge, this is the first pretraining framework to learn modality-disentangled representations in recommendation scenarios. Extensive experiments on three public real-world datasets demonstrate the effectiveness of our pretraining solution against a series of state-of-the-art alternatives, which results in the significant performance gain of 4.70%-17.44%.
更多查看译文
关键词
Pretraining, Disentangle Encoder, Contrasive Learning, ModalityDisentangled, Representation
AI 理解论文
溯源树
样例
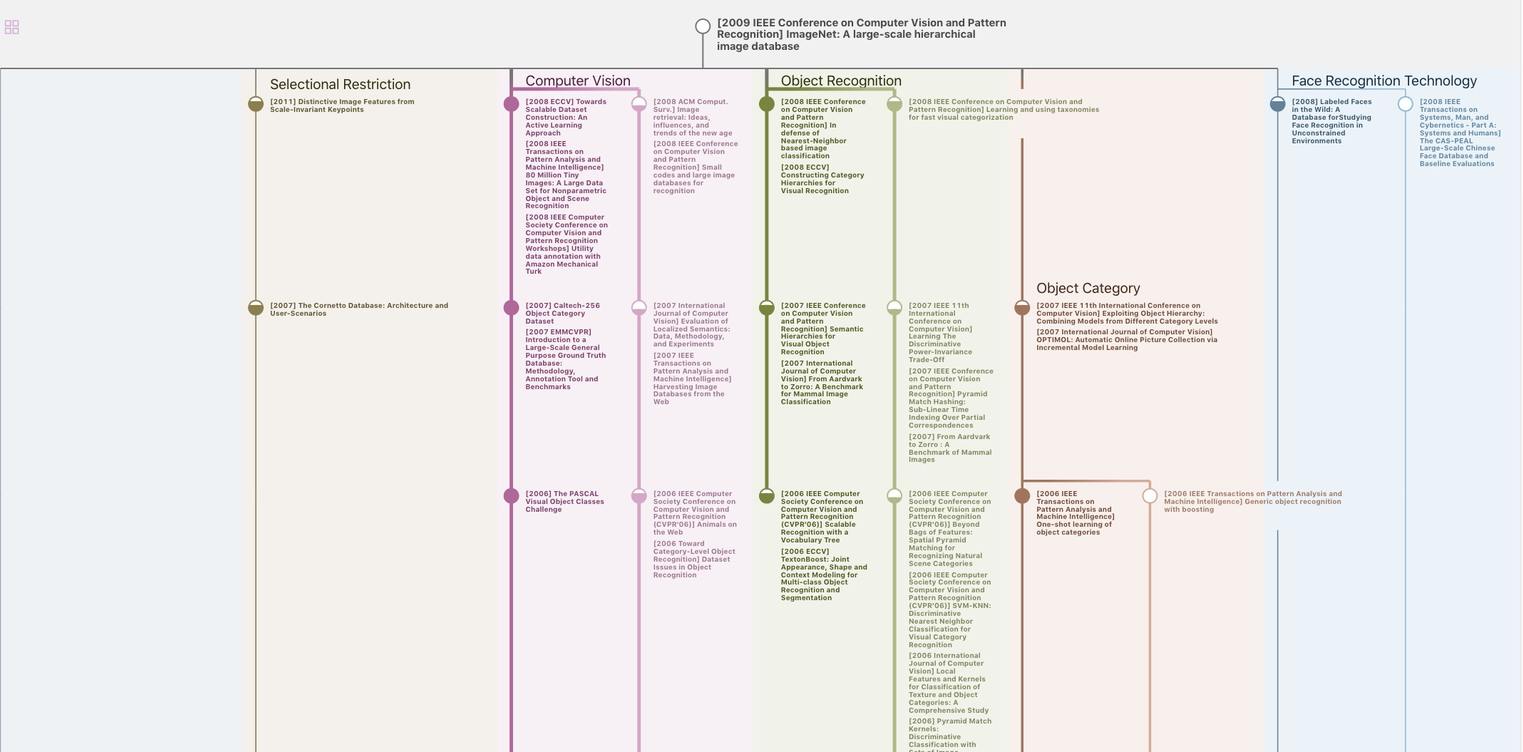
生成溯源树,研究论文发展脉络
Chat Paper
正在生成论文摘要