Fairness Audit of Machine Learning Models with Confidential Computing
International World Wide Web Conference(2022)
摘要
ABSTRACTAlgorithmic discrimination is one of the significant concerns in applying machine learning models to a real-world system. Many researchers have focused on developing fair machine learning algorithms without discrimination based on legally protected attributes. However, the existing research has barely explored various security issues that can occur while evaluating model fairness and verifying fair models. In this study, we propose a fairness audit framework that assesses the fairness of ML algorithms while addressing potential security issues such as data privacy, model secrecy, and trustworthiness. To this end, our proposed framework utilizes confidential computing and builds a chain of trust through enclave attestation primitives combined with public scrutiny and state-of-the-art software-based security techniques, enabling fair ML models to be securely certified and clients to verify a certified one. Our micro-benchmarks on various ML models and real-world datasets show the feasibility of the fairness certification implemented with Intel SGX in practice. In addition, we analyze the impact of data poisoning, which is an additional threat during data collection for fairness auditing. Based on the analysis, we illustrate the theoretical curves of fairness gap and minimal group size and the empirical results of fairness certification on poisoned datasets.
更多查看译文
关键词
Fairness, Algorithmic audit, Security and privacy, Confidential computing
AI 理解论文
溯源树
样例
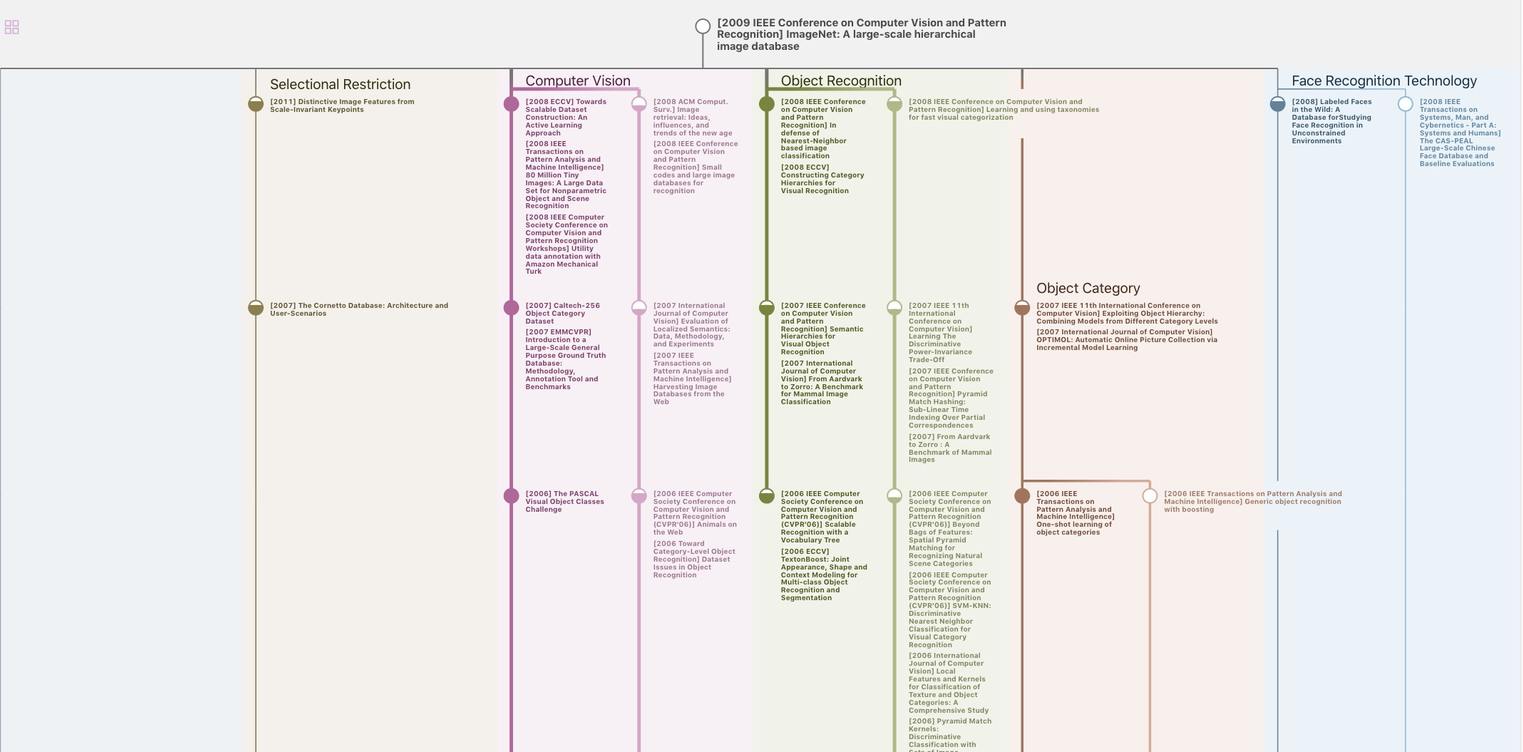
生成溯源树,研究论文发展脉络
Chat Paper
正在生成论文摘要