Diversifying Neural Dialogue Generation via Negative Distillation
North American Chapter of the Association for Computational Linguistics (NAACL)(2022)
摘要
Generative dialogue models suffer badly from the generic response problem, limiting their applications to a few toy scenarios. Recently, an interesting approach, namely negative training, has been proposed to alleviate this problem by reminding the model not to generate high-frequency responses during training. However, its performance is hindered by two issues, ignoring low-frequency but generic responses and bringing low-frequency but meaningless responses. In this paper, we propose a novel negative training paradigm, called negative distillation, to keep the model away from the undesirable generic responses while avoiding the above problems. First, we introduce a negative teacher model that can produce query-wise generic responses, and then the student model is required to maximize the distance with multi-level negative knowledge. Empirical results show that our method outperforms previous negative training methods significantly.
更多查看译文
关键词
neural dialogue generation,negative distillation
AI 理解论文
溯源树
样例
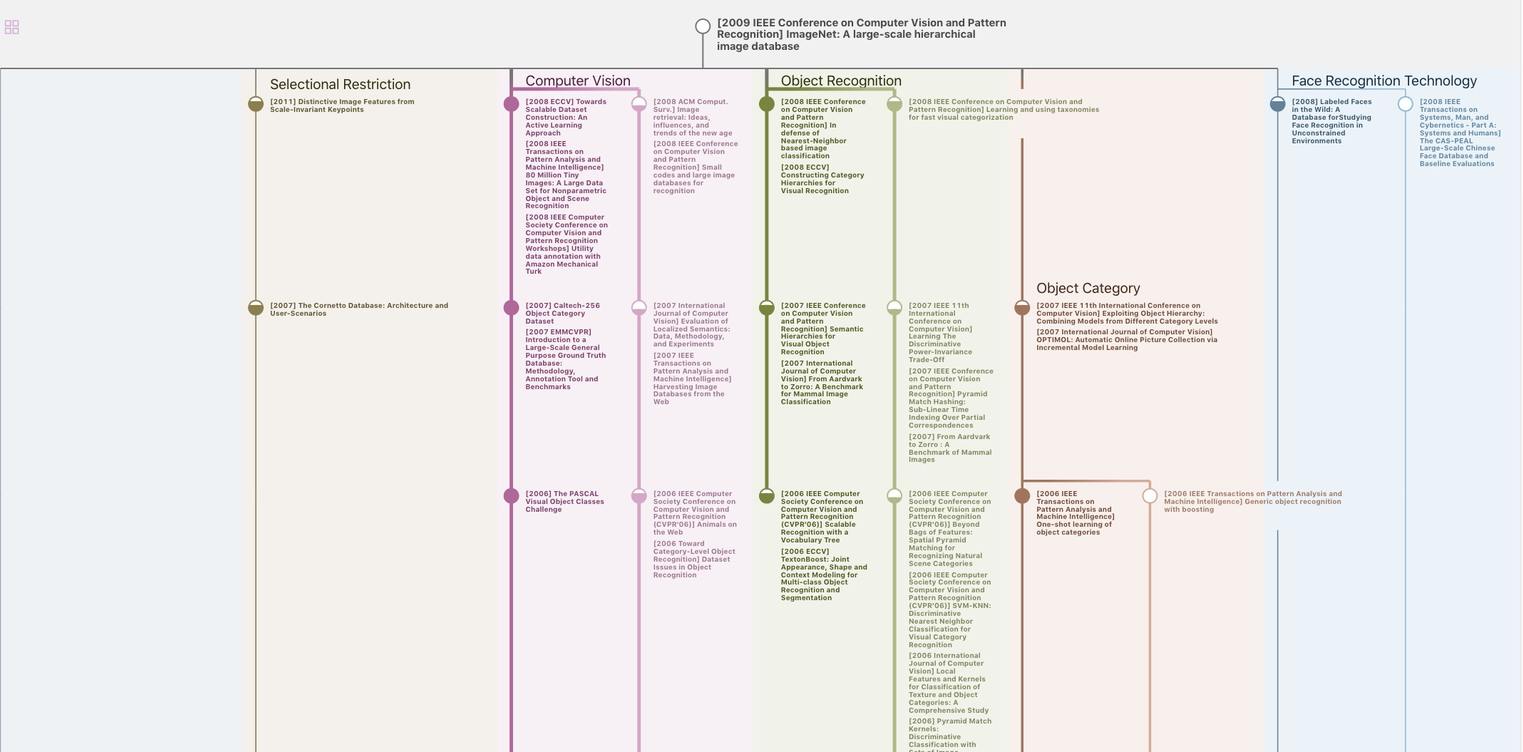
生成溯源树,研究论文发展脉络
Chat Paper
正在生成论文摘要