Moment-Based Invariants for Probabilistic Loops with Non-polynomial Assignments
QUANTITATIVE EVALUATION OF SYSTEMS (QEST 2022)(2022)
摘要
We present a method to automatically approximate moment-based invariants of probabilistic programs with non-polynomial updates of continuous state variables to accommodate more complex dynamics. Our approach leverages polynomial chaos expansion to approximate nonlinear functional updates as sums of orthogonal polynomials. We exploit this result to automatically estimate state-variable moments of all orders in Prob-solvable loops with non-polynomial updates. We showcase the accuracy of our estimation approach in several examples, such as the turning vehicle model and the Taylor rule in monetary policy.
更多查看译文
关键词
Probabilistic programs, Prob-solvable loops, Polynomial, Chaos Expansion, Non-linear updates
AI 理解论文
溯源树
样例
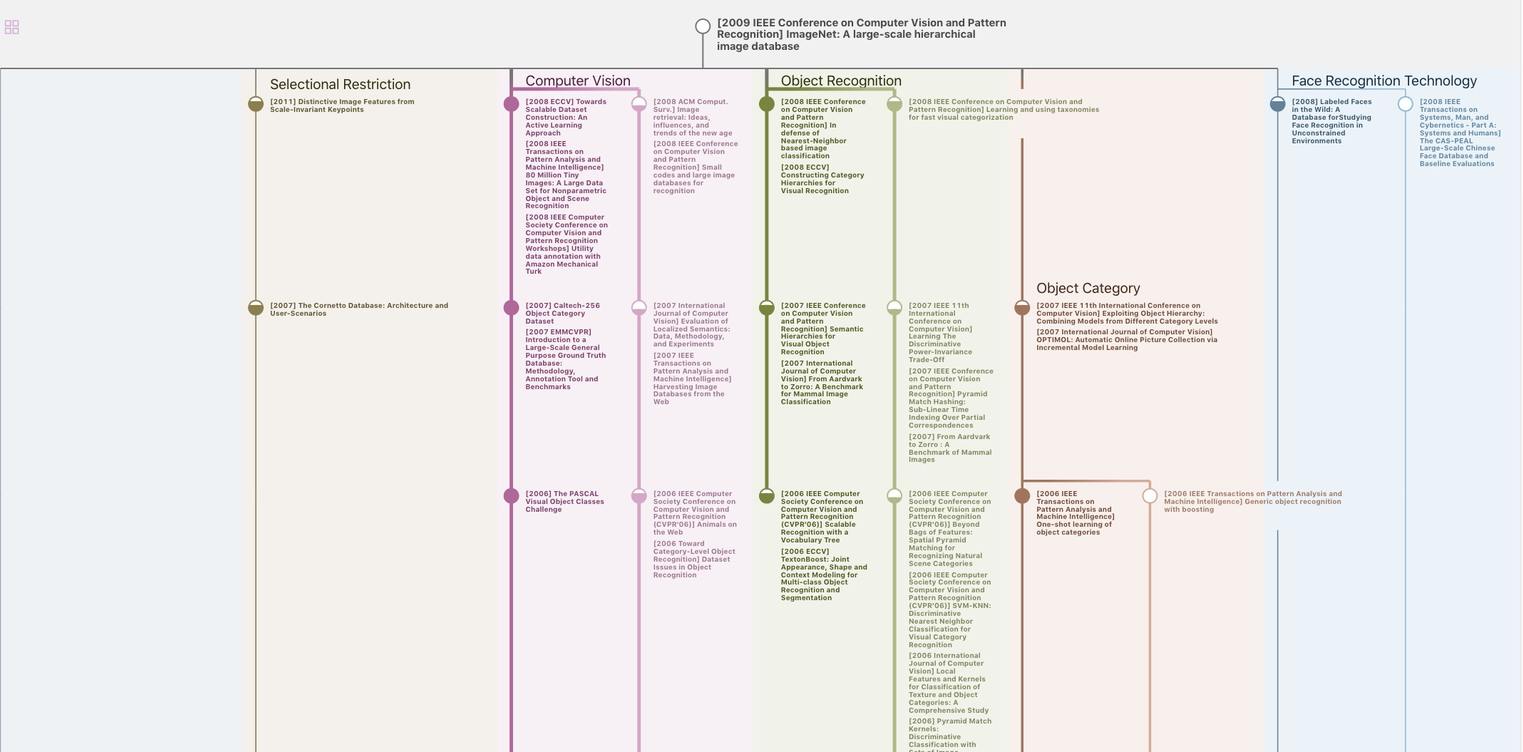
生成溯源树,研究论文发展脉络
Chat Paper
正在生成论文摘要