Characterizing the optimal solutions to the isotonic regression problem for identifiable functionals
Annals of the Institute of Statistical Mathematics(2021)
摘要
In general, the solution to a regression problem is the minimizer of a given loss criterion and depends on the specified loss function. The nonparametric isotonic regression problem is special, in that optimal solutions can be found by solely specifying a functional. These solutions will then be minimizers under all loss functions simultaneously as long as the loss functions have the requested functional as the Bayes act. For the functional, the only requirement is that it can be defined via an identification function, with examples including the expectation, quantile, and expectile functionals. Generalizing classical results, we characterize the optimal solutions to the isotonic regression problem for identifiable functionals by rigorously treating these functionals as set-valued. The results hold in the case of totally or partially ordered explanatory variables. For total orders, we show that any solution resulting from the pool-adjacent-violators algorithm is optimal.
更多查看译文
关键词
Order-restricted optimization problems,Partial order,Simultaneous optimality,Pool-adjacent-violators algorithm,Consistent loss functions
AI 理解论文
溯源树
样例
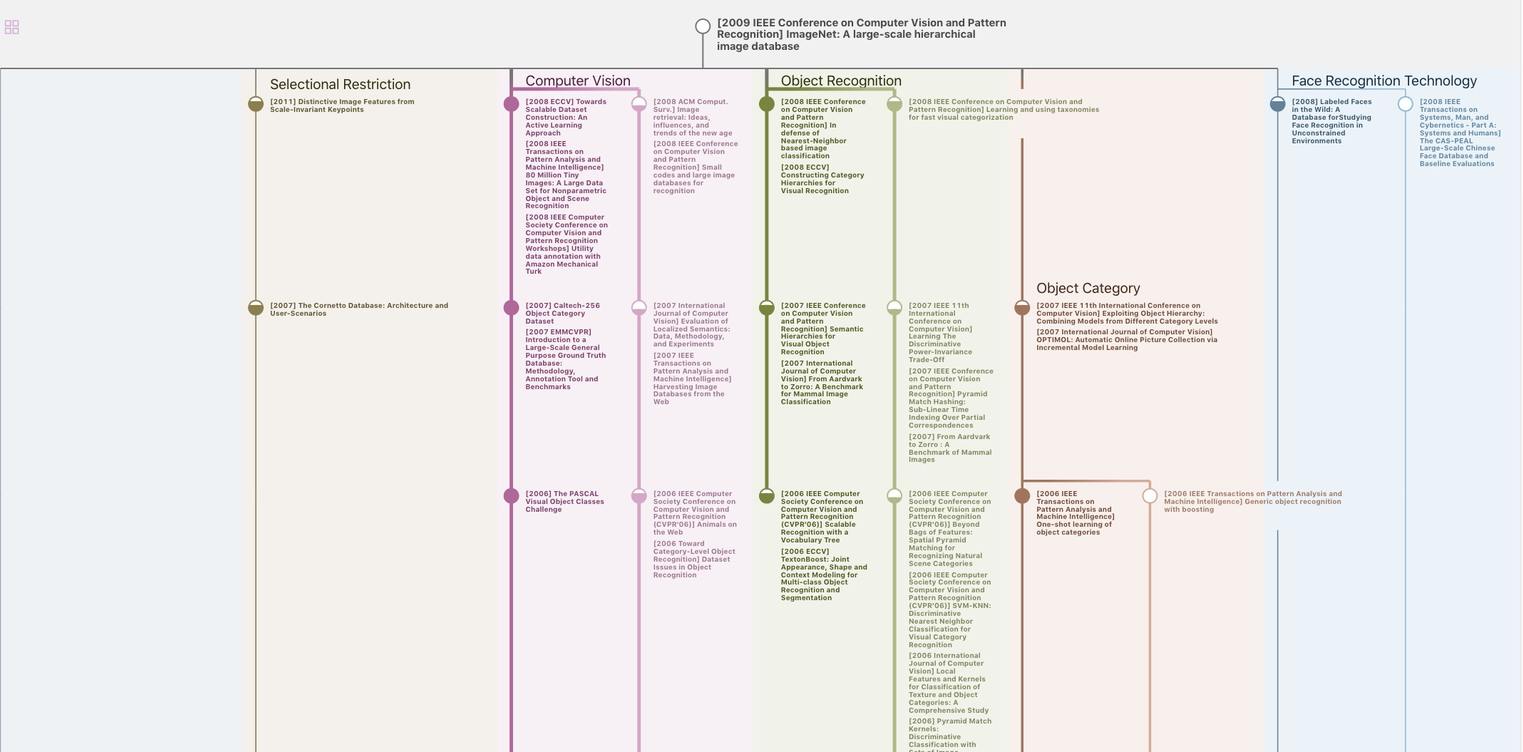
生成溯源树,研究论文发展脉络
Chat Paper
正在生成论文摘要