Adaptive initialization LSHADE algorithm enhanced with gradient-based repair for real-world constrained optimization
Knowledge-Based Systems(2022)
Abstract
A straightforward and effective scheme for adaptive initialization is proposed and coupled with the Linearly reduced population size Success History based Adaptive Differential Evolution (LSHADE) for solving constrained optimization problems. The novel contributions are in the initialization phase: (i) exploiting the information of problem dimensions and the corresponding computational budgets to determine the suitable initial population size and hence the use of linear size reduction. Based on the initial population size, the next two inputs in the constraint handling scheme are appropriately initialized: (ii) the use of tolerance for inequality constraints and (iii) the choice between the original and a proposed setting of a crucial parameter controlling the tolerance, in order to balance exploration–exploitation orientation. Additionally, a gradient-based repair technique is incorporated into the method to significantly improve solution feasibility in high-dimensional problems. The proposed algorithm, referred to as En(L)SHADE, is validated on the CEC2020 competition challenging test-suite of 57 real-world constrained problems in various fields. Beside the advantage of simplicity, En(L)SHADE also shows a superior performance over the benchmark challengers.
MoreTranslated text
Key words
Adaptive initialization,Gradient-based repair,LSHADE algorithm,Real-world optimization problem
AI Read Science
Must-Reading Tree
Example
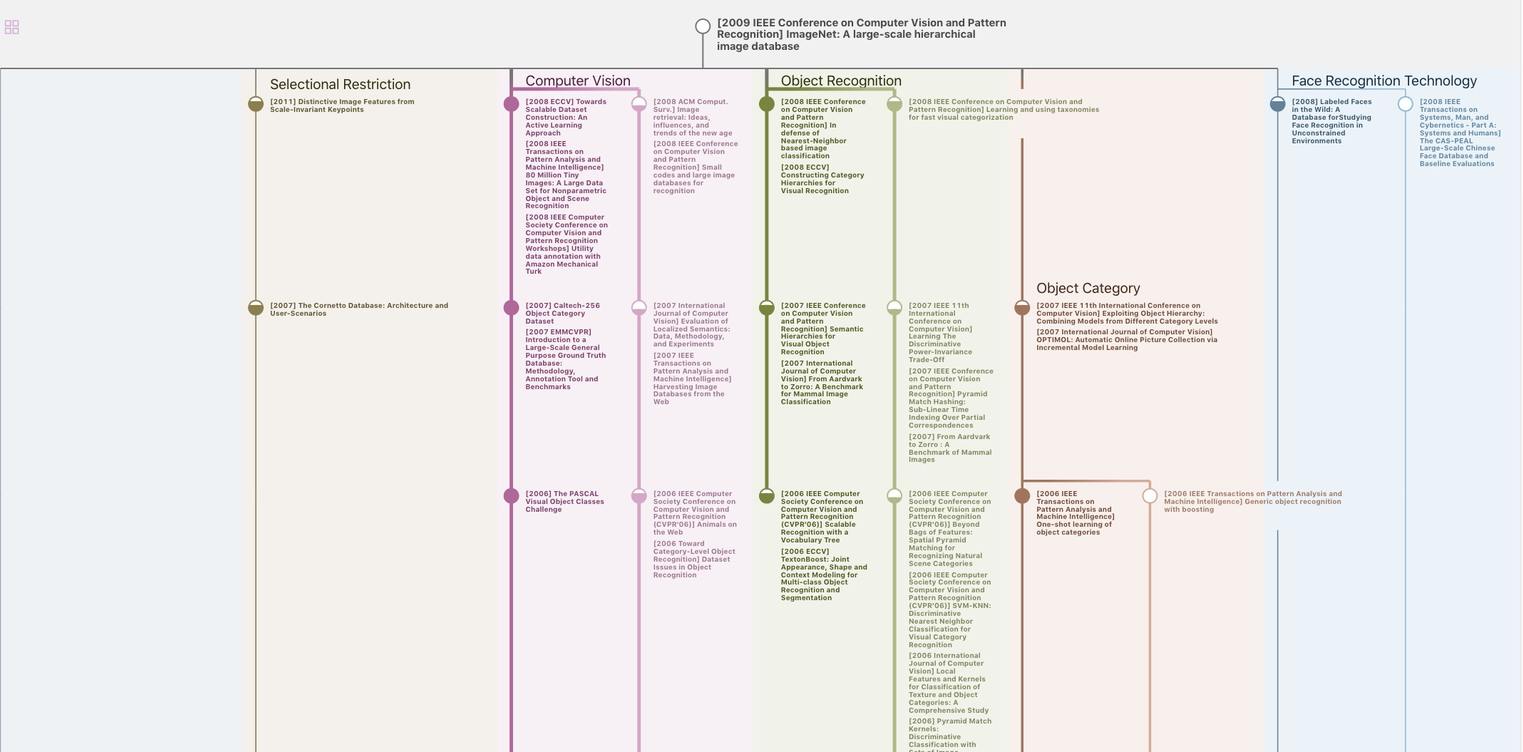
Generate MRT to find the research sequence of this paper
Chat Paper
Summary is being generated by the instructions you defined