Bayesian Inference of Phenomenologycal EoS of Neutron Stars with Recent Observations
arxiv(2022)
摘要
The description of stellar interiors remains as a big challenge for the nuclear astrophysics community. The consolidated knowledge is restricted to density regions around the saturation of hadronic matter $\rho _{0} = 2.8\times 10^{14} {\rm\ g\ cm^{-3}}$, regimes where our nuclear models are successfully applied. As one moves towards higher densities and extreme conditions up to five to twenty times $\rho_{0}$, little can be said about the microphysics of such objects. Here, we employ a Markov Chain Monte Carlo (MCMC) strategy in order to access the variability of polytropic three-pircewised models for neutron star equation of states. With a fixed description of the hadronic matter, we explore a variety of models for the high density regimes leading to stellar masses up to 2.5 $M_{\odot}$. In addition, we also discuss the use of a Bayesian power regression model with heteroscedastic error. The set of EoS from LIGO was used as inputs and treated as data set for testing case.
更多查看译文
AI 理解论文
溯源树
样例
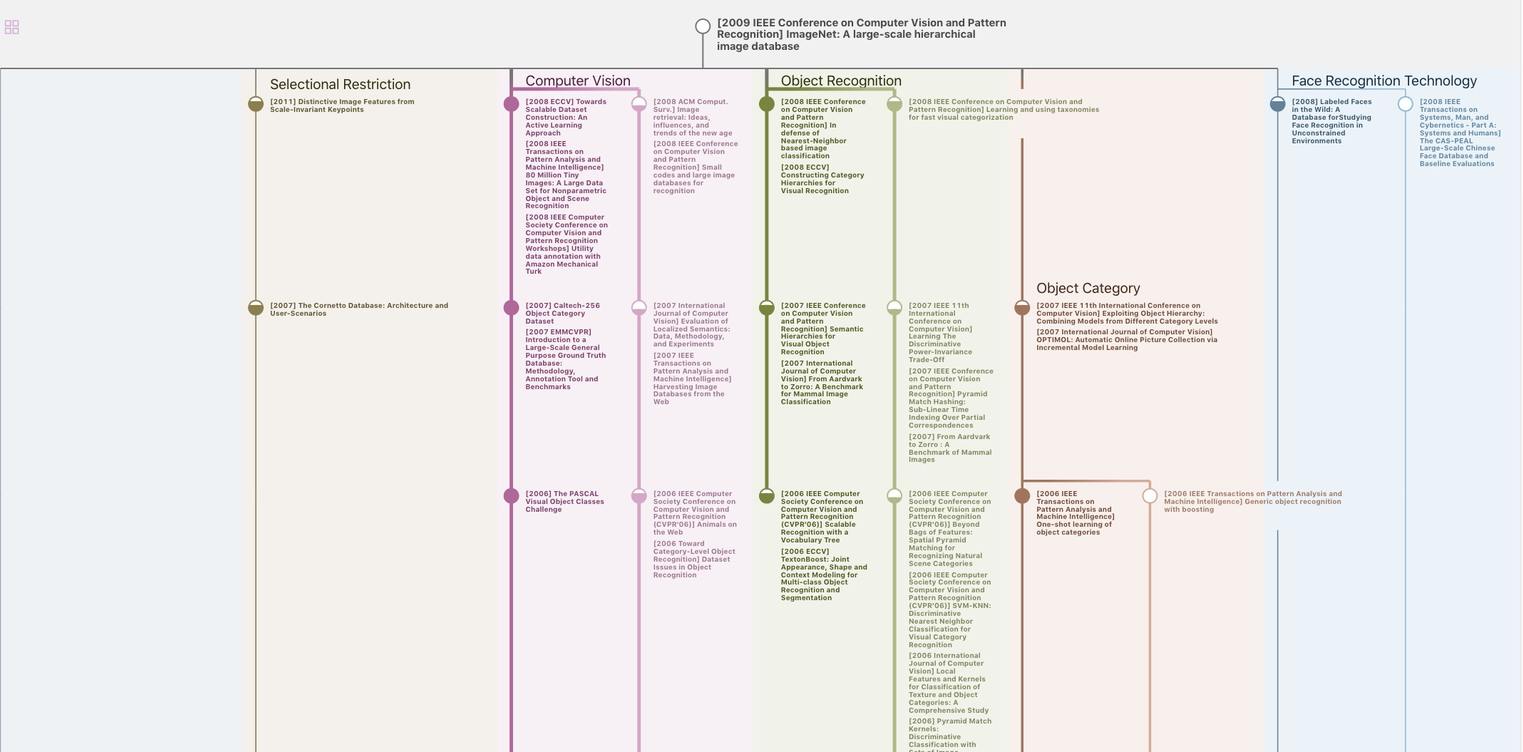
生成溯源树,研究论文发展脉络
Chat Paper
正在生成论文摘要