Decision Support System for Predicting Survivability of Hepatitis Patients
FRONTIERS IN PUBLIC HEALTH(2022)
摘要
Background and ObjectiveViral hepatitis is a major public health concern on a global scale. It predominantly affects the world's least developed countries. The most endemic regions are resource constrained, with a low human development index. Chronic hepatitis can lead to cirrhosis, liver failure, cancer and eventually death. Early diagnosis and treatment of hepatitis infection can help to reduce disease burden and transmission to those at risk of infection or reinfection. Screening is critical for meeting the WHO's 2030 targets. Consequently, automated systems for the reliable prediction of hepatitis illness. When applied to the prediction of hepatitis using imbalanced datasets from testing, machine learning (ML) classifiers and known methodologies for encoding categorical data have demonstrated a wide range of unexpected results. Early research also made use of an artificial neural network to identify features without first gaining a thorough understanding of the sequence data. MethodsTo help in accurate binary classification of diagnosis (survivability or mortality) in patients with severe hepatitis, this paper suggests a deep learning-based decision support system (DSS) that makes use of bidirectional long/short-term memory (BiLSTM). Balanced data was utilized to predict hepatitis using the BiLSTM model. ResultsIn contrast to previous investigations, the trial results of this suggested model were encouraging: 95.08% accuracy, 94% precision, 93% recall, and a 93% F1-score. ConclusionsIn the field of hepatitis detection, the use of a BiLSTM model for classification is better than current methods by a significant margin in terms of improved accuracy.
更多查看译文
关键词
disease diagnosis, deep learning, hepatitis diagnostics, decision support system, bidirectional LSTM
AI 理解论文
溯源树
样例
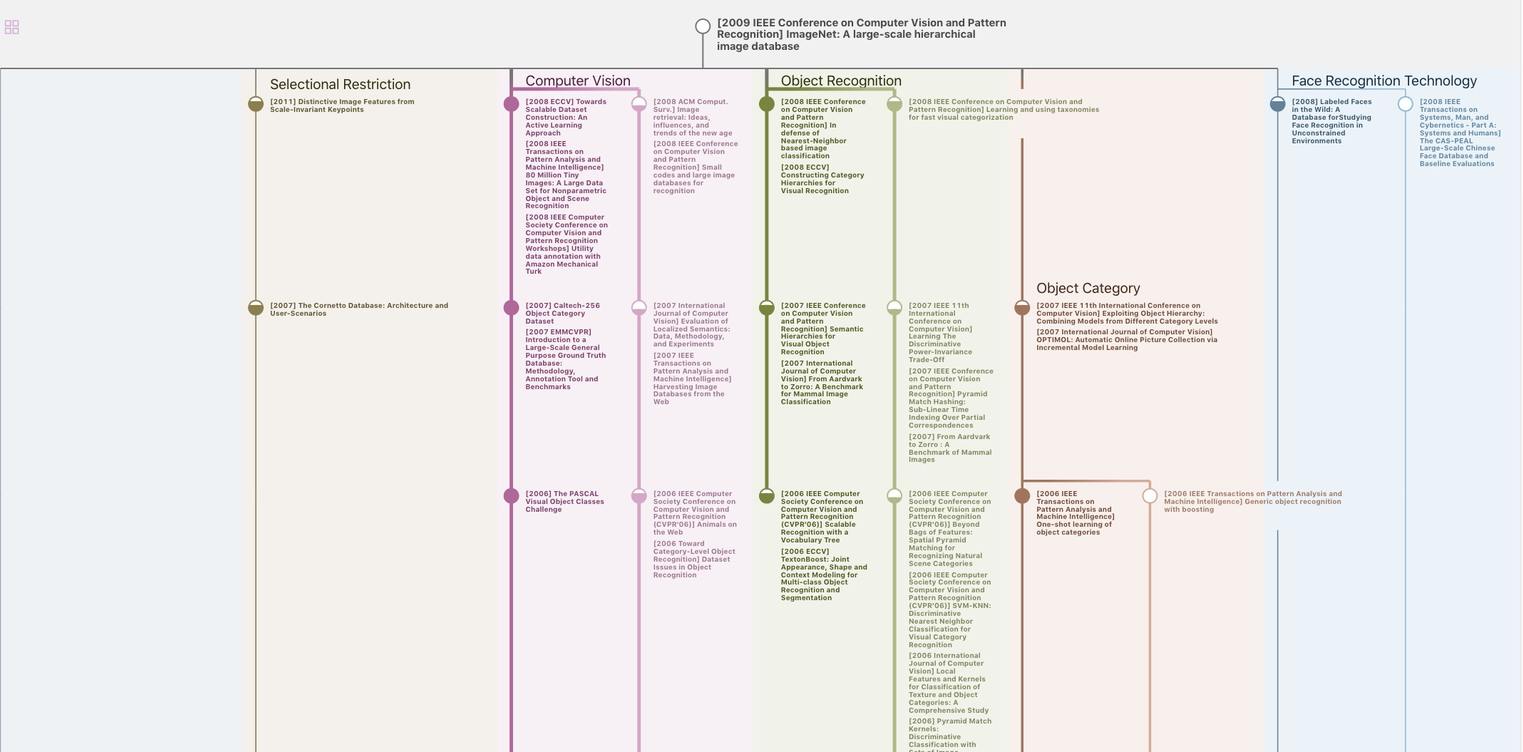
生成溯源树,研究论文发展脉络
Chat Paper
正在生成论文摘要