CT-based radiomics analysis in the prediction of response to neoadjuvant chemotherapy in locally advanced gastric cancer: A dual-center study.
Radiotherapy and oncology : journal of the European Society for Therapeutic Radiology and Oncology(2022)
摘要
BACKGROUND:To investigate the ability of the CT-based radiomics models for pretreatment prediction of the response to neoadjuvant chemotherapy (NAC) in patients with locally advanced gastric cancer (LAGC).
METHODS:This retrospective analysis included 279 consecutive LAGC patients from center I (training cohort, n = 196; internal validation cohort, n = 83) who were examined by contrast-enhanced CT before treatment and 211 consecutive patients from center II who were recruited as an external validation cohort. A total of 102 features were extracted from the portal venous phase CT images, and feature selection was further subjected to three-step procedures. Next, five classifications, including Logistic Regression (LR), Naive Bayes, Random forest (RF), Support Vector Machine (SVM), and Extreme Gradient Boosting (XGB) algorithms, were applied to construct radiomics models for predicting the good-responder (GR) to NAC in the training cohort. The prediction performances were evaluated using ROC and decision curve analysis (DCA).
RESULTS:No statistically significant difference was detected for all clinicopathological characteristics. Additionally, allsix key features were significantly different between GR and poor-responder (PR). Compared to models from other classifiers, the model obtained with XGB showed promising prediction performance with the highest AUC of 0.790(95%CI: 0.700-0.880) in the training cohort. The corresponding AUCs were 0.784(95%CI, 0.659-0.908) and 0.803(95%CI, 0.717-0.888) in the internal and external validation cohorts, respectively. DCA confirmed the clinical utility.
CONCLUSIONS:The proposed pretreatment CT-based radiomics models revealed good performances in predicting response to NAC and thus may be used to improve clinical treatment in LAGC patients.
更多查看译文
AI 理解论文
溯源树
样例
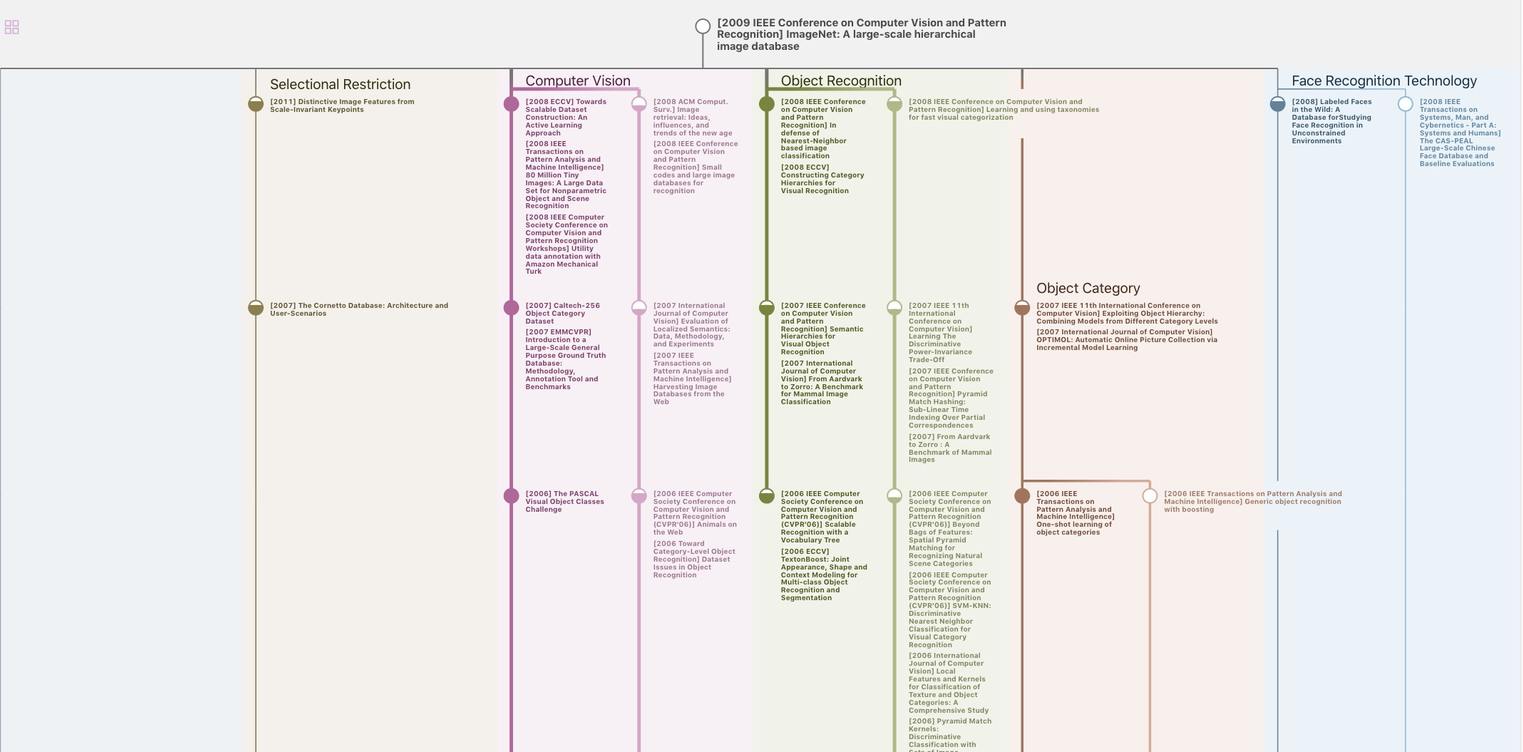
生成溯源树,研究论文发展脉络
Chat Paper
正在生成论文摘要