Open-Set Semi-Supervised Learning for 3D Point Cloud Understanding.
ICPR(2022)
摘要
Semantic understanding of 3D point cloud relies on learning models with massively annotated data, which, in many cases, are expensive or difficult to collect. This has led to an emerging research interest in semi-supervised learning (SSL) for 3D point cloud. It is commonly assumed in SSL that the unlabeled data are drawn from the same distribution as that of the labeled ones; This assumption, however, rarely holds true in realistic environments. Blindly using out-of-distribution (OOD) unlabeled data could harm SSL performance. In this work, we propose to selectively utilize unlabeled data through sample weighting, so that only conducive unlabeled data would be prioritized. To estimate the weights, we adopt a bi-level optimization framework which iteratively optimizes a metaobjective on a held-out validation set and a task-objective on a training set. Faced with the instability of efficient bi-level optimizers, we further propose three regularization techniques to enhance the training stability. Extensive experiments on 3D point cloud classification and segmentation tasks verify the effectiveness of our proposed method. We also demonstrate the feasibility of a more efficient training strategy.
更多查看译文
关键词
3D point cloud classification,3D point cloud segmentation,bi-level optimization,semantic understanding,semisupervised learning,SSL,training set,weight estimation
AI 理解论文
溯源树
样例
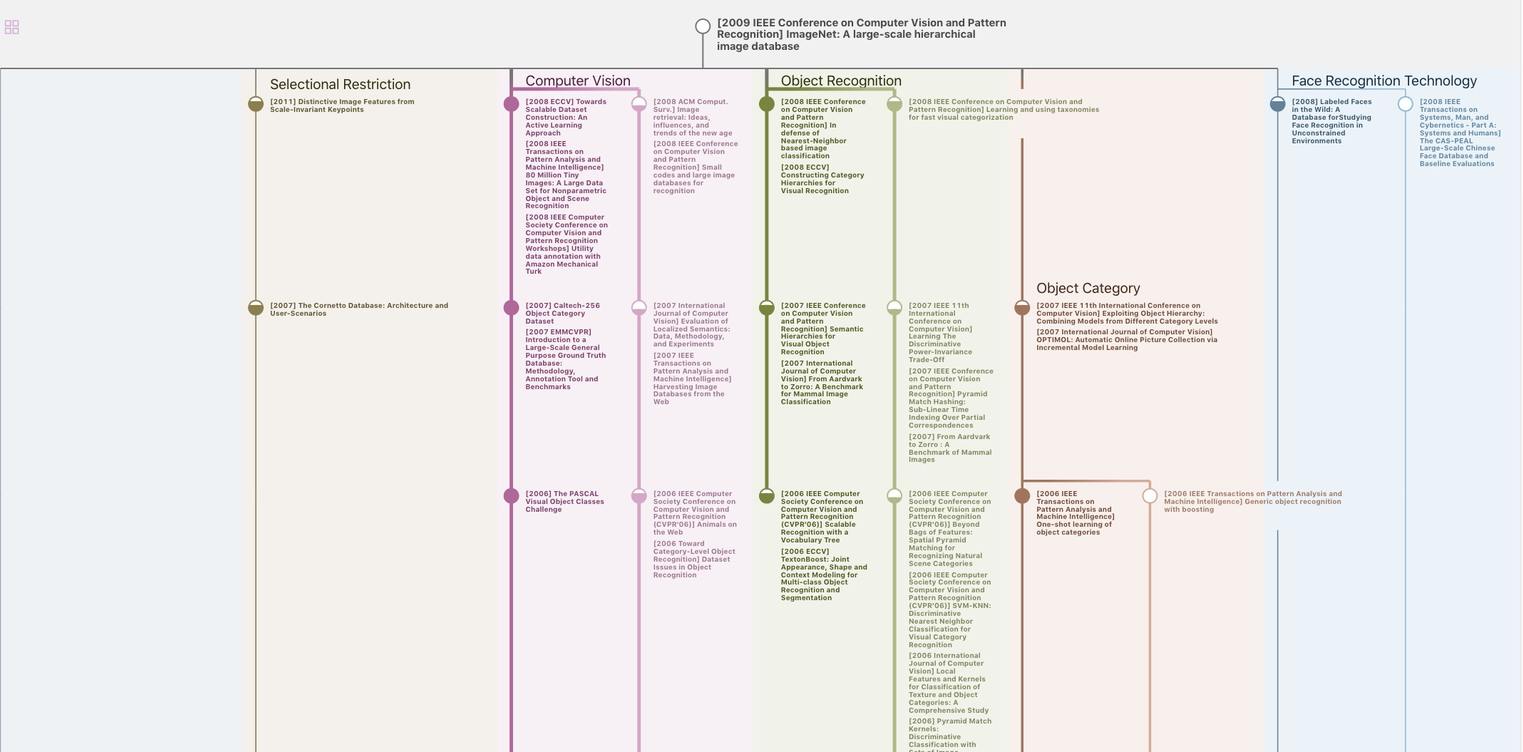
生成溯源树,研究论文发展脉络
Chat Paper
正在生成论文摘要