BERTops: Studying BERT Representations under a Topological Lens
IEEE International Joint Conference on Neural Network (IJCNN)(2022)
摘要
Proposing scoring functions to effectively understand, analyze and learn various properties of high dimensional hidden representations of large-scale transformer models like BERT can be a challenging task. In this work, we explore a new direction by studying the topological features of BERT hidden representations using persistent homology (PH). We propose a novel scoring function named "persistence scoring function (PSF)" which: (i) accurately captures the homology of the high-dimensional hidden representations and correlates well with the test set accuracy of a wide range of datasets and outperforms existing scoring metrics, (ii) captures interesting post fine-tuning "per-class" level properties from both qualitative and quantitative viewpoints, (iii) is more stable to perturbations as compared to the baseline functions, which makes it a very robust proxy, and (iv) finally, also serves as a predictor of the attack success rates for a wide category of black-box and white-box adversarial attack methods. Our extensive correlation experiments demonstrate the practical utility of PSF on various NLP tasks relevant to BERT.
更多查看译文
关键词
Machine Learning,Neural Networks,Persistent Homology,BERT
AI 理解论文
溯源树
样例
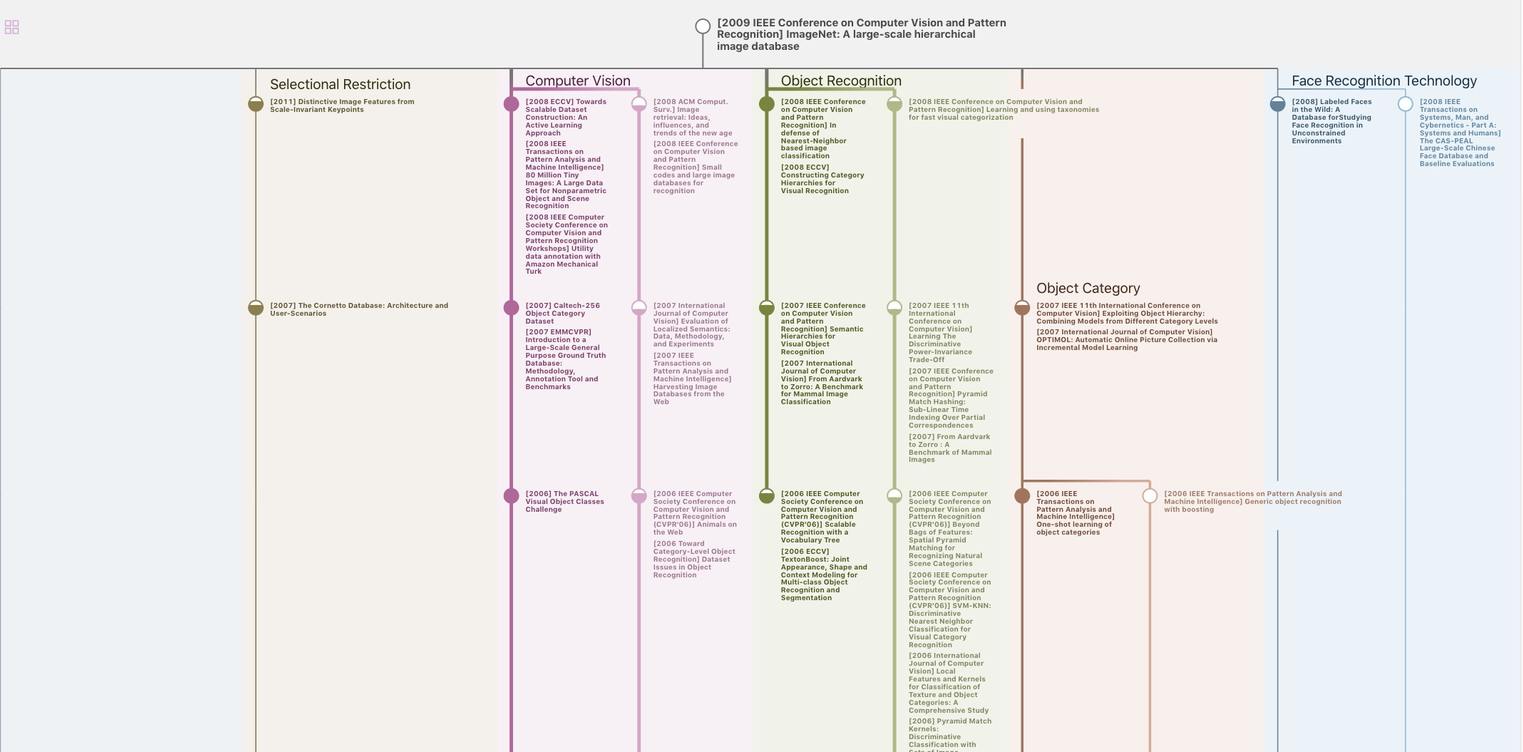
生成溯源树,研究论文发展脉络
Chat Paper
正在生成论文摘要