GPUNet: Searching the Deployable Convolution Neural Networks for GPUs.
CoRR(2022)
摘要
Customizing Convolution Neural Networks (CNN) for production use has been a challenging task for DL practitioners. This paper intends to expedite the model customization with a model hub that contains the optimized models tiered by their inference latency using Neural Architecture Search (NAS). To achieve this goal, we build a distributed NAS system to search on a novel search space that consists of prominent factors to impact latency and accuracy. Since we target GPU, we name the NAS optimized models as GPUNet, which establishes a new SOTA Pareto frontier in inference latency and accuracy. Within 1ms, GPUNet is 2x faster than EfficientNet-X and FBNetV3 with even better accuracy. We also validate GPUNet on detection tasks, and GPUNet consistently outperforms EfficientNet-X and FB-NetV3 on COCO detection tasks in both latency and accuracy. All of these data validate that our NAS system is effective and generic to handle different design tasks. With this NAS system, we expand GPUNet to cover a wide range of latency targets such that DL practitioners can deploy our models directly in different scenarios.
更多查看译文
关键词
Efficient learning and inferences, Vision applications and systems
AI 理解论文
溯源树
样例
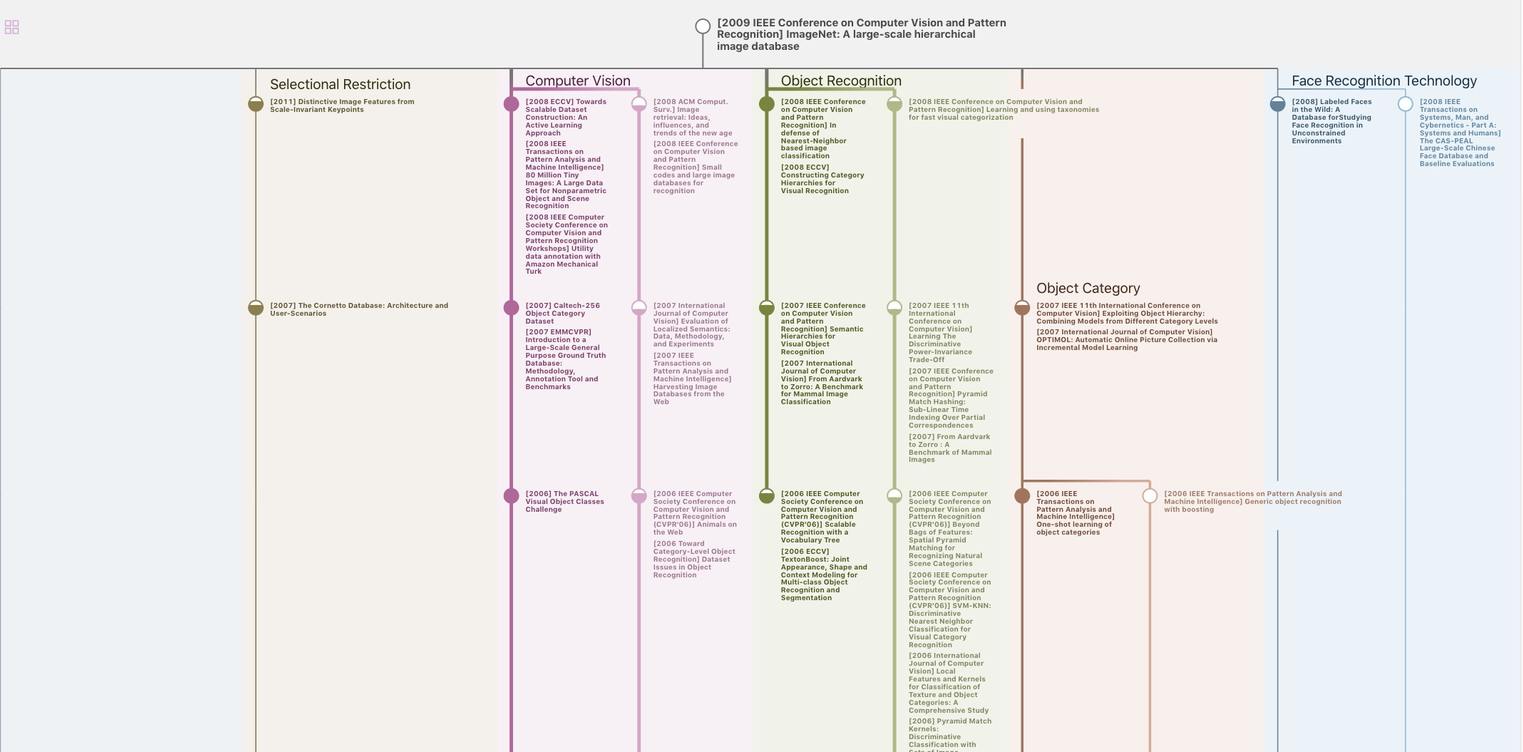
生成溯源树,研究论文发展脉络
Chat Paper
正在生成论文摘要