Solving PDEs by variational physics-informed neural networks: an a posteriori error analysis
arxiv(2022)
摘要
We consider the discretization of elliptic boundary-value problems by variational physics-informed neural networks (VPINNs), in which test functions are continuous, piecewise linear functions on a triangulation of the domain. We define an a posteriori error estimator, made of a residual-type term, a loss-function term, and data oscillation terms. We prove that the estimator is both reliable and efficient in controlling the energy norm of the error between the exact and VPINN solutions. Numerical results are in excellent agreement with the theoretical predictions.
更多查看译文
关键词
Deep neural networks,A posteriori error estimators,Petrov–Galerkin discretizations,Elliptic boundary-value problems
AI 理解论文
溯源树
样例
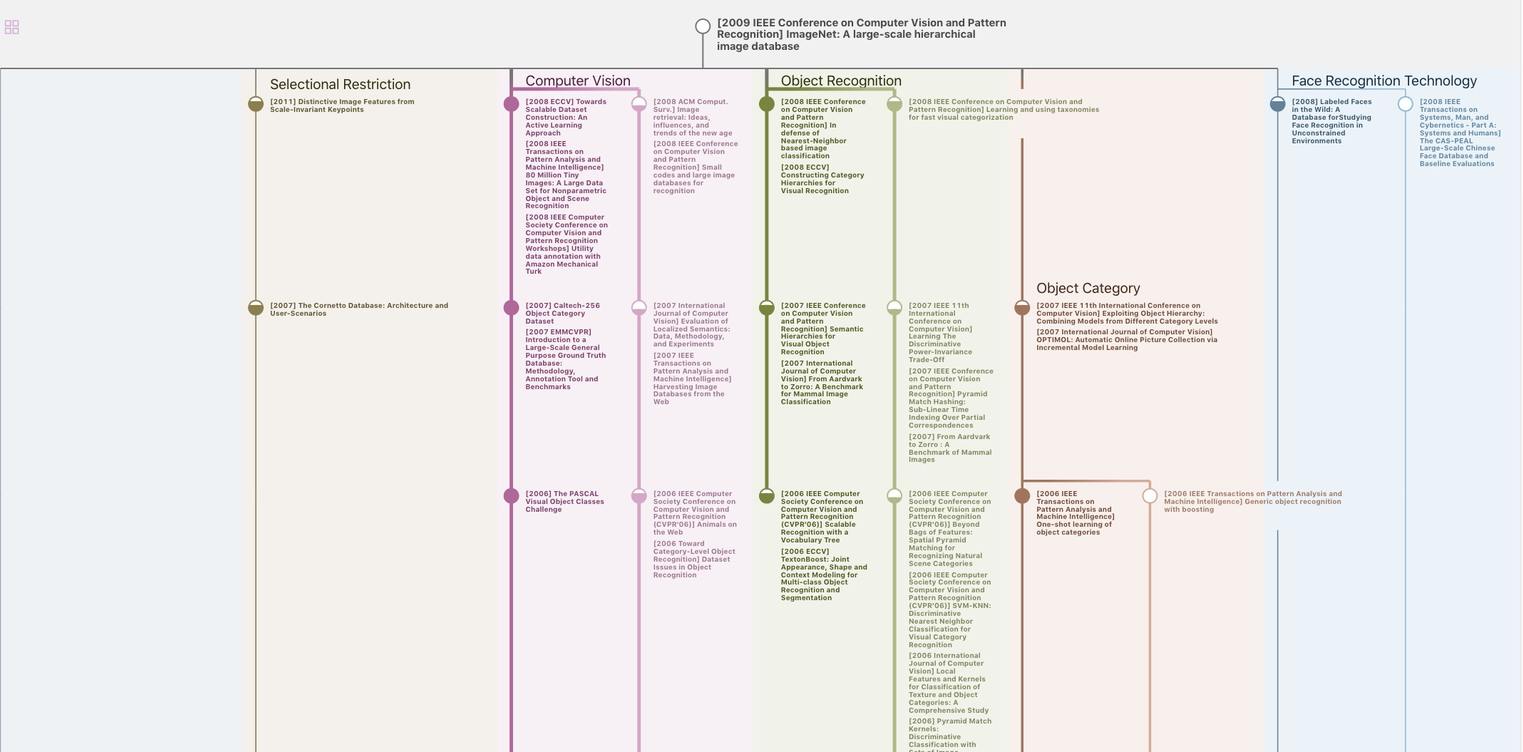
生成溯源树,研究论文发展脉络
Chat Paper
正在生成论文摘要