Neural Network Optimal Feedback Control With Guaranteed Local Stability
IEEE Open Journal of Control Systems(2022)
摘要
Recent research shows that supervised learning can be an effective tool for designing near-optimal feedback controllers for high-dimensional nonlinear dynamic systems. But the behavior of neural network controllers is still not well understood. In particular, some neural networks with high test accuracy can fail to even locally stabilize the dynamic system. To address this challenge we propose several novel neural network architectures, which we show guarantee local asymptotic stability while retaining the approximation capacity to learn the optimal feedback policy semi-globally. The proposed architectures are compared against standard neural network feedback controllers through numerical simulations of two high-dimensional nonlinear optimal control problems: stabilization of an unstable Burgers-type partial differential equation, and altitude and course tracking for an unmanned aerial vehicle. The simulations demonstrate that standard neural networks can fail to stabilize the dynamics even when trained well, while the proposed architectures are always at least locally stabilizing and can achieve near-optimal performance.
更多查看译文
关键词
Computational methods,machine learning and control,neural networks,nonlinear control systems,optimal control
AI 理解论文
溯源树
样例
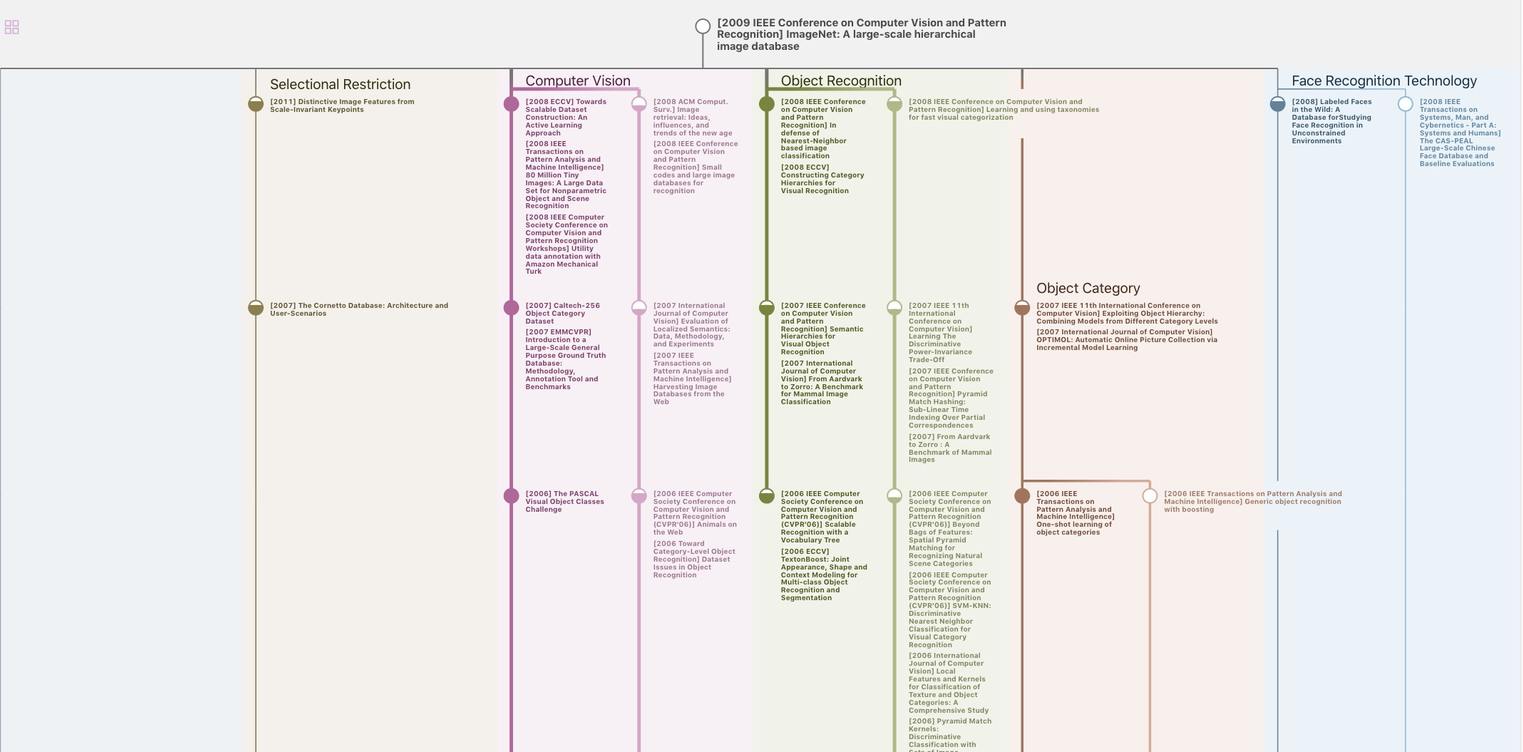
生成溯源树,研究论文发展脉络
Chat Paper
正在生成论文摘要