End-to-End Signal Classification in Signed Cumulative Distribution Transform Space.
arxiv(2024)
摘要
This paper presents a new end-to-end signal classification method using the signed cumulative distribution transform (SCDT). We adopt a transport generative model to define the classification problem. We then make use of mathematical properties of the SCDT to render the problem easier in transform domain, and solve for the class of an unknown sample using a nearest local subspace (NLS) search algorithm in SCDT domain. Experiments show that the proposed method provides high accuracy classification results while being computationally cheap, data efficient, and robust to out-of-distribution samples with respect to the existing end-to-end classification methods. The implementation of the proposed method in Python language is integrated as a part of the software package PyTransKit.
更多查看译文
关键词
signed cumulative distribution
AI 理解论文
溯源树
样例
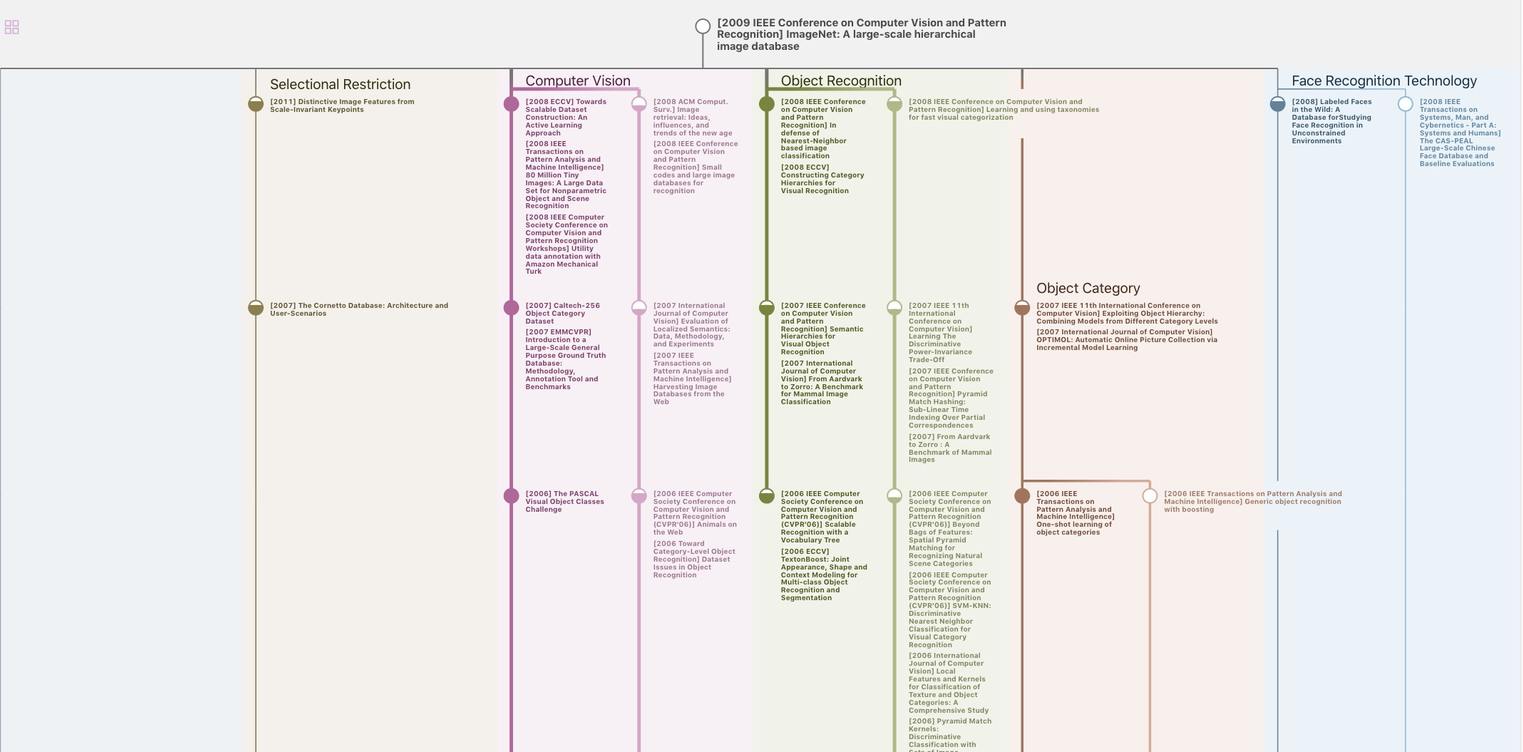
生成溯源树,研究论文发展脉络
Chat Paper
正在生成论文摘要