Implicit Regularization Properties of Variance Reduced Stochastic Mirror Descent.
International Symposium on Information Theory (ISIT)(2022)
摘要
In machine learning and statistical data analysis, we often run into objective function that is a summation: the number of terms in the summation possibly is equal to the sample size, which can be enormous. In such a setting, the stochastic mirror descent (SMD) algorithm is a numerically efficient method -- each iteration involving a very small subset of the data. The variance reduction version of SMD (VRSMD) can further improve SMD by inducing faster convergence. On the other hand, algorithms such as gradient descent and stochastic gradient descent have the implicit regularization property that leads to better performance in terms of the generalization errors. Little is known on whether such a property holds for VRSMD. We prove here that the discrete VRSMD estimator sequence converges to the minimum mirror interpolant in the linear regression. This establishes the implicit regularization property for VRSMD. As an application of the above result, we derive a model estimation accuracy result in the setting when the true model is sparse. We use numerical examples to illustrate the empirical power of VRSMD.
更多查看译文
关键词
implicit regularization,variance reduction,stochastic mirror descent,overparameterization
AI 理解论文
溯源树
样例
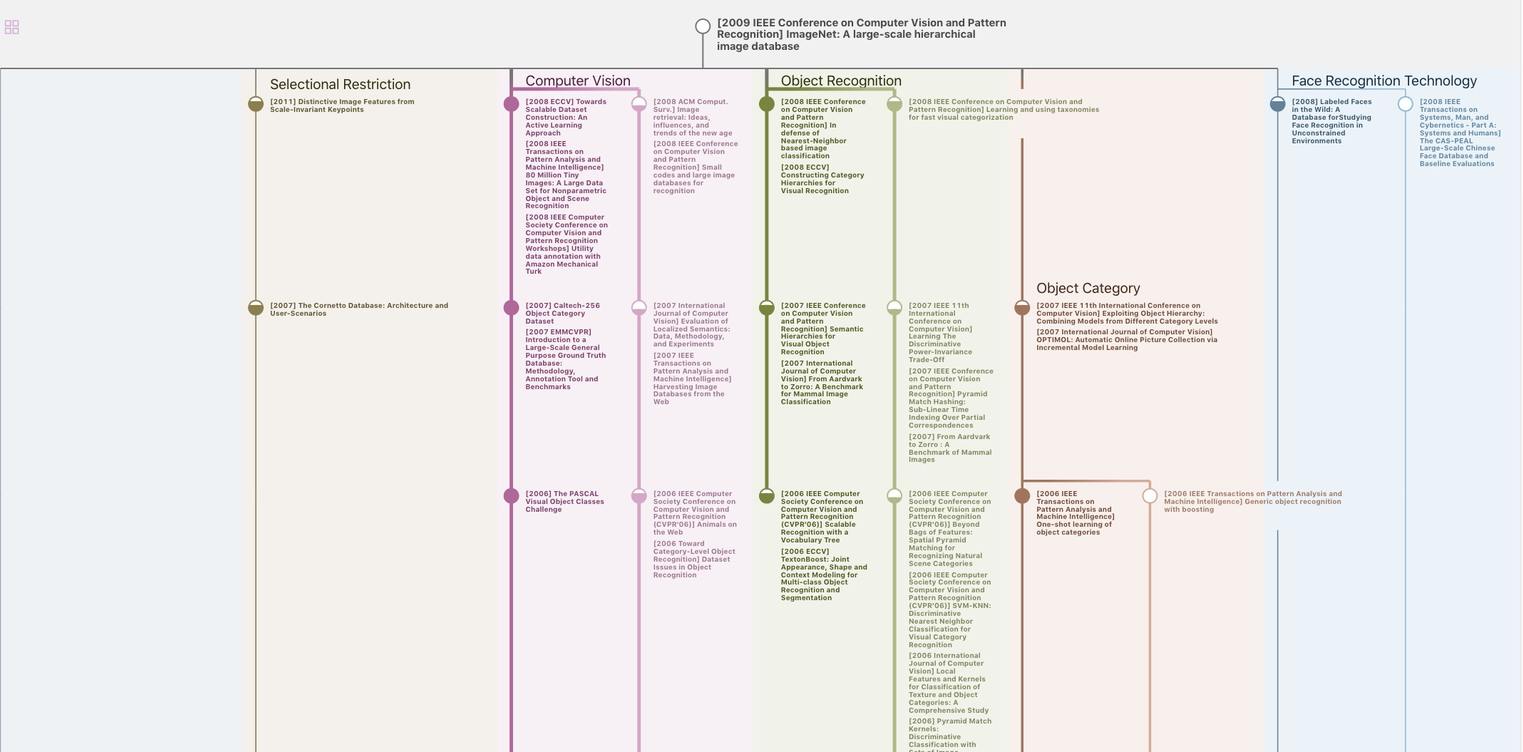
生成溯源树,研究论文发展脉络
Chat Paper
正在生成论文摘要