Conditional average treatment effect estimation with treatment offset models
arxiv(2022)
摘要
Treatment effect estimates are often available from randomized controlled trials as a single average treatment effect for a certain patient population. Estimates of the conditional average treatment effect (CATE) are more useful for individualized treatment decision making, but randomized trials are often too small to estimate the CATE. There are several examples in medical literature where the assumption of a known constant relative treatment effect (e.g. an odds-ratio) is used to estimate CATE models from large observational datasets. One approach to estimating these CATE models is by using the relative treatment effect as an offset, while estimating the covariate-specific baseline risk. Whether this is a valid approach in the presence of unobserved confounding is unknown. We demonstrate for a simple example that offset models do not recover the true CATE in the presence of unobserved confounding. We then explore the magnitude of this bias in numerical experiments. For virtually all plausible confounding magnitudes, estimating the CATE using offset models is more accurate than assuming a single absolute treatment effect whenever there is sufficient variation in the baseline risk. Next, we observe that the odds-ratios reported in randomized controlled trials are not the odds-ratios that are needed in offset models because trials often report the marginal odds-ratio. We introduce a constraint to better use marginal odds-ratios from randomized controlled trials and find that the newly introduced constrained offset models have lower bias than standard offset models. Finally, we highlight directions for future research for exploiting the assumption of a constant relative treatment effect with offset models.
更多查看译文
AI 理解论文
溯源树
样例
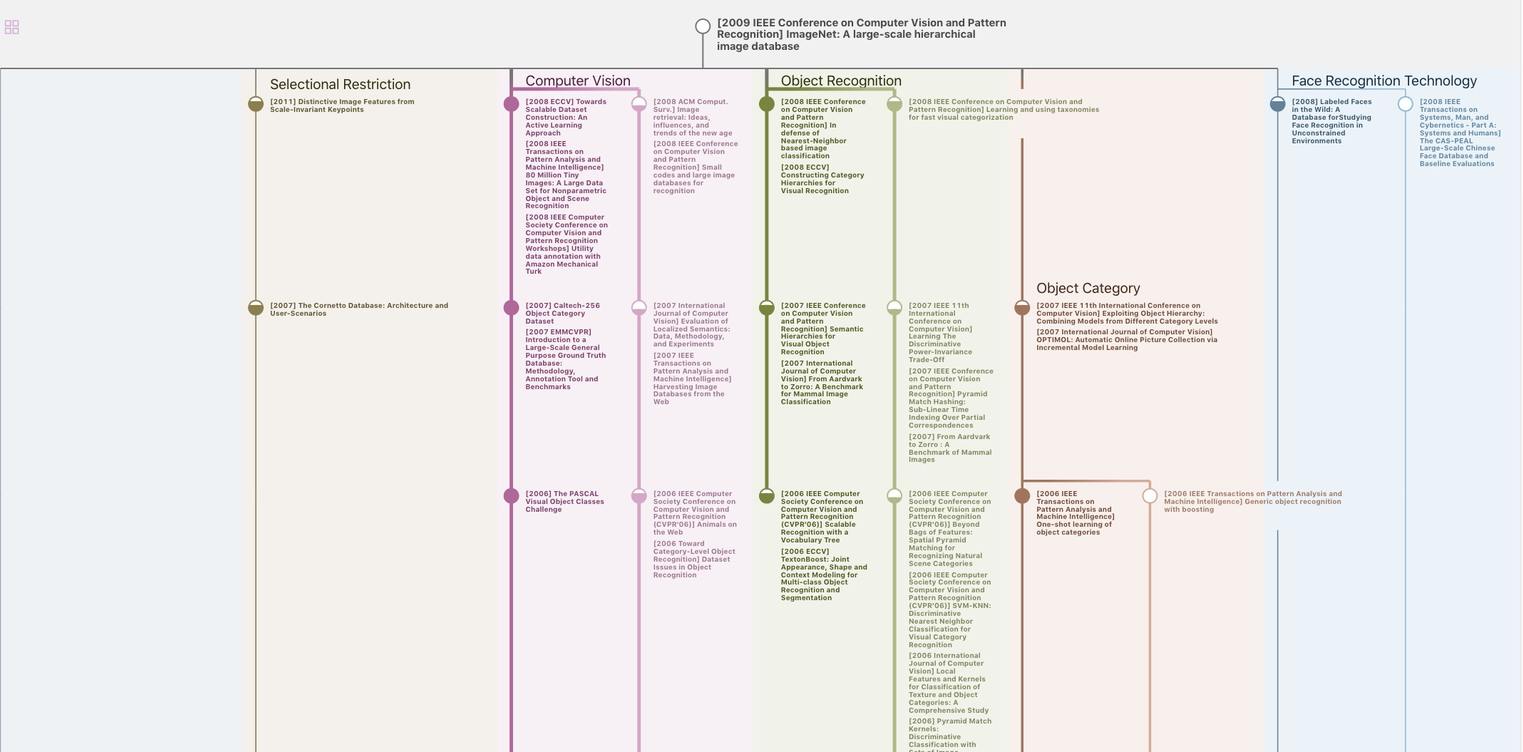
生成溯源树,研究论文发展脉络
Chat Paper
正在生成论文摘要