KERMIT - A Transformer-Based Approach for Knowledge Graph Matching
arxiv(2022)
摘要
One of the strongest signals for automated matching of knowledge graphs and ontologies are textual concept descriptions. With the rise of transformer-based language models, text comparison based on meaning (rather than lexical features) is available to researchers. However, performing pairwise comparisons of all textual descriptions of concepts in two knowledge graphs is expensive and scales quadratically (or even worse if concepts have more than one description). To overcome this problem, we follow a two-step approach: we first generate matching candidates using a pre-trained sentence transformer (so called bi-encoder). In a second step, we use fine-tuned transformer cross-encoders to generate the best candidates. We evaluate our approach on multiple datasets and show that it is feasible and produces competitive results.
更多查看译文
关键词
knowledge graph,transformer-based
AI 理解论文
溯源树
样例
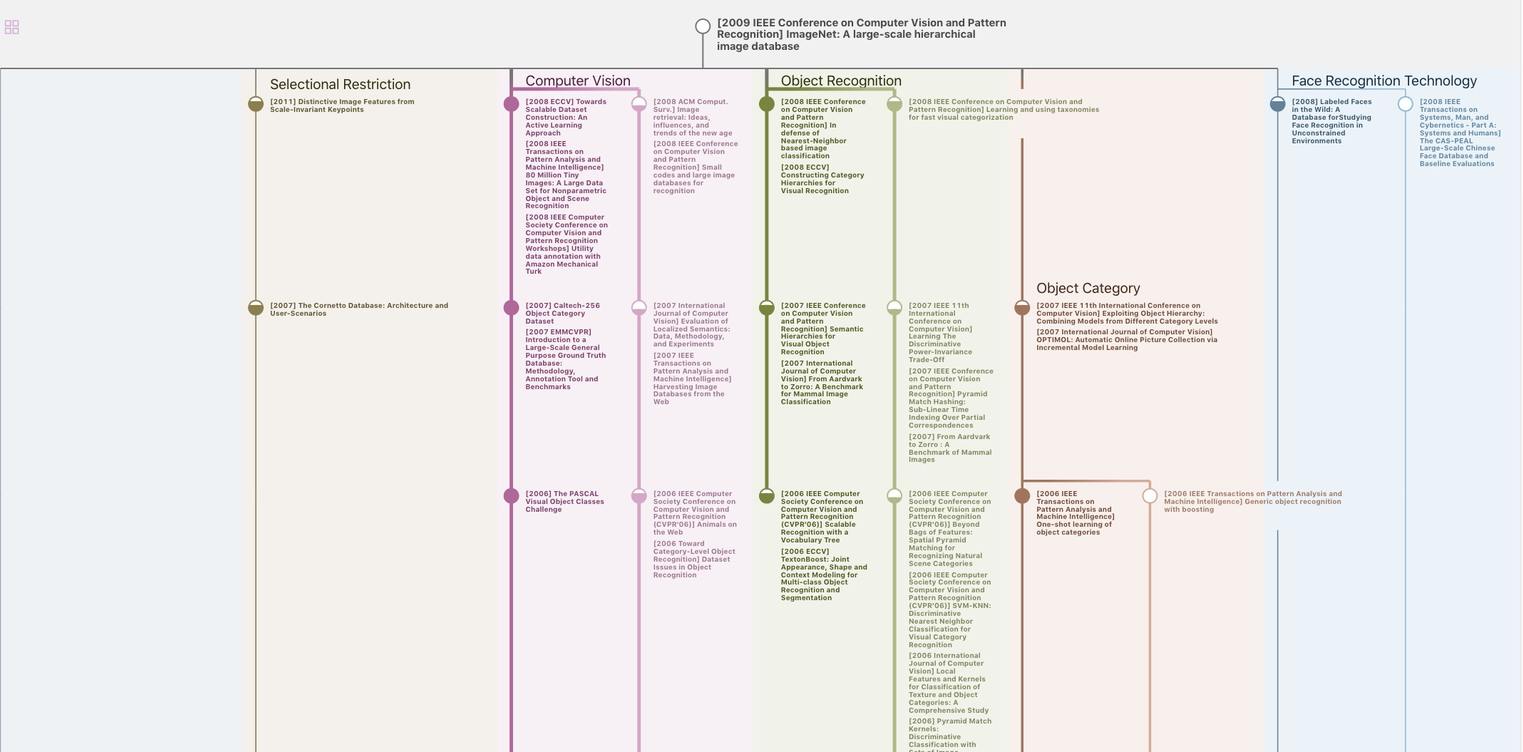
生成溯源树,研究论文发展脉络
Chat Paper
正在生成论文摘要