Toward automatic beam angle selection for pencil-beam scanning proton liver treatments: A deep learning-based approach
MEDICAL PHYSICS(2022)
摘要
Background Dose deposition characteristics of proton radiation can be advantageous over photons. Proton treatment planning, however, poses additional challenges for the planners. Proton therapy is usually delivered with only a small number of beam angles, and the quality of a proton treatment plan is largely determined by the beam angles employed. Finding the optimal beam angles for a proton treatment plan requires time and experience, motivating the investigation of automatic beam angle selection methods. Purpose A deep learning-based approach to automatic beam angle selection is proposed for the proton pencil-beam scanning treatment planning of liver lesions. Methods We cast beam-angle selection as a multi-label classification problem. To account for angular boundary discontinuity, the underlying convolution neural network is trained with the proposed Circular Earth Mover's Distance-based regularization and multi-label circular-smooth label technique. Furthermore, an analytical algorithm emulating proton treatment planners' clinical practice is employed in post-processing to improve the output of the model. Forty-nine patients that received proton liver treatments between 2017 and 2020 were randomly divided into training (n = 31), validation (n = 7), and test sets (n = 11). AI-selected beam angles were compared with those angles selected by human planners, and the dosimetric outcome was investigated by creating plans using knowledge-based treatment planning. Results For 7 of the 11 cases in the test set, AI-selected beam angles agreed with those chosen by human planners to within 20 degrees (median angle difference = 10 degrees; mean = 18.6 degrees). Moreover, out of the total 22 beam angles predicted by the model, 15 (68%) were within 10 degrees of the human-selected angles. The high correlation in beam angles resulted in comparable dosimetric statistics between proton treatment plans generated using AI- and human-selected angles. For the cases with beam angle differences exceeding 20 degrees, the dosimetric analysis showed similar plan quality although with different emphases on organ-at-risk sparing. Conclusions This pilot study demonstrated the feasibility of a novel deep learning-based beam angle selection technique. Testing on liver cancer patients showed that the resulting plans were clinically viable with comparable dosimetric quality to those using human-selected beam angles. In tandem with auto-contouring and knowledge-based treatment planning tools, the proposed model could represent a pathway for nearly fully automated treatment planning in proton therapy.
更多查看译文
关键词
beam angle selection, deep learning, knowledge-based treatment planning, pencil-beam scanning, proton therapy
AI 理解论文
溯源树
样例
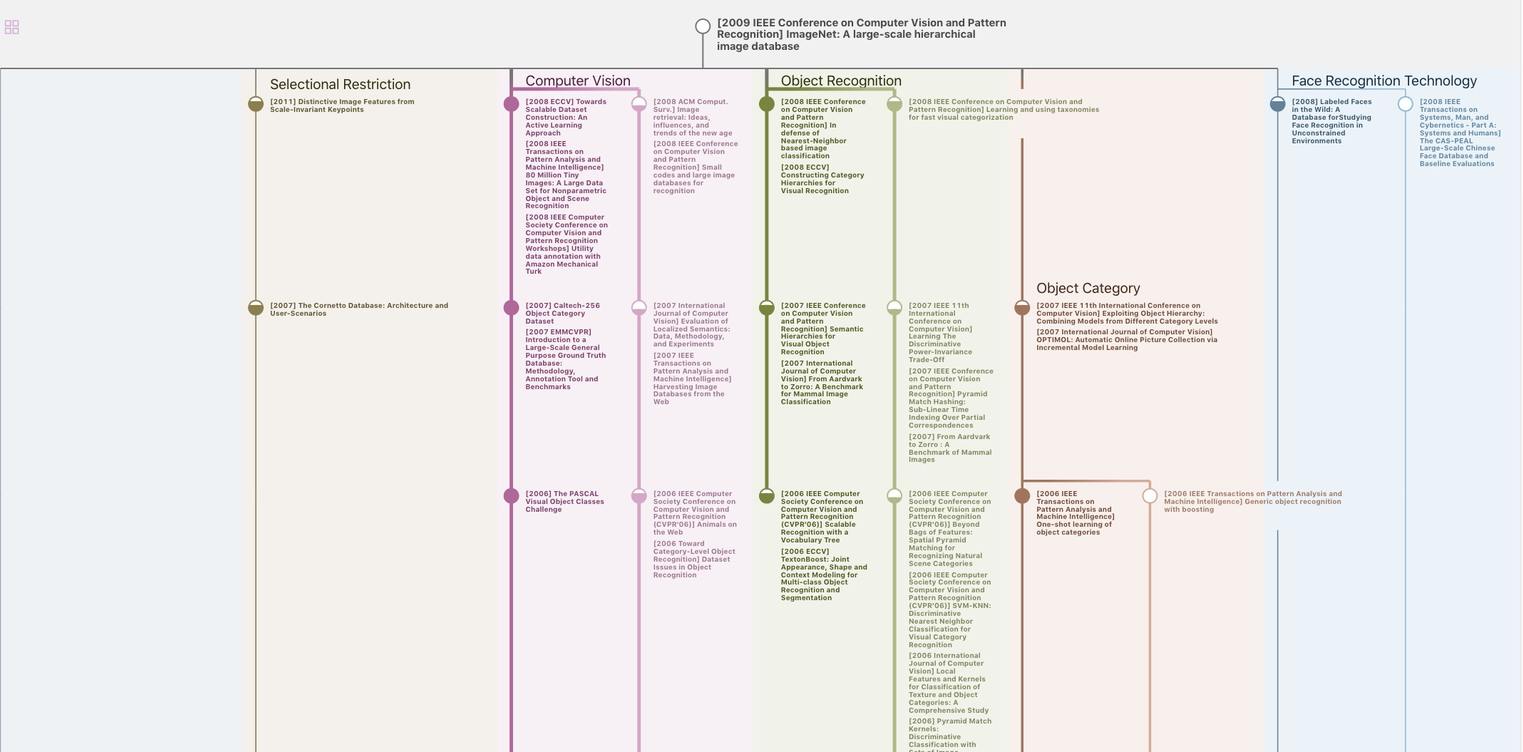
生成溯源树,研究论文发展脉络
Chat Paper
正在生成论文摘要